ACT Basics
Overview
In this tutorial, you will learn some of the basic features of ACT! We we walk through the different displays you can use, and some of the helpful functionality ACT provides.
Installation
If you don’t have ACT already installed, it can be installed using pip or conda using the commands below. Additional information on installation can be found in the ACT User Guide.
pip install act-atmos
conda install -c conda-forge act-atmos
Some features of ACT are only available if you have some optional dependencies installed. For example, Skew-T plots of radiosonde data will require that MetPy is installed. Additional optional dependencies are listed in ACT’s documentation
Imports
First we are going to start by importing all the necessary python libraries that we need which is just act and matplotlib. We also need glob
to deal with files.
import act
import matplotlib.pyplot as plt
import glob
Download ARM Data
Next we are going to download the data we are going to use for this session using the ARM Live Data webservice.
Once you have an ARM account, you should be able to utilize this webservice as well. All you need to do is login to get your token. We have setup a sample token for you in this workshop, shown below!
# Set your own username and token if you have it
username = 'armlive_training'
token = '6413343e8c6a6ade'
# ACT module for downloading data from the ARM web service
results = act.discovery.download_data(username, token, 'sgpmfrsr7nchE11.b1', '2021-03-29', '2021-03-29')
[DOWNLOADING] sgpmfrsr7nchE11.b1.20210329.070000.nc
Reading in a NetCDF File
Congratulations, you just downloaded a file from just the command line! Next up is to read the file into an xarray object using the ACT reader. We then can use Jupyter to print out an interactive listing of everything in the object.
ds = act.io.armfiles.read_netcdf(results)
ds
/Users/mgrover/miniforge3/envs/pyart-docs/lib/python3.10/site-packages/tqdm/auto.py:22: TqdmWarning: IProgress not found. Please update jupyter and ipywidgets. See https://ipywidgets.readthedocs.io/en/stable/user_install.html
from .autonotebook import tqdm as notebook_tqdm
<xarray.Dataset> Dimensions: (time: 4320, bench_angle: 181, wavelength: 750) Coordinates: * time (time) datetime64[ns] 2021-03-29... * bench_angle (bench_angle) float32 0.0 ... 180.0 Dimensions without coordinates: wavelength Data variables: (12/158) base_time datetime64[ns] 2021-03-29 time_offset (time) datetime64[ns] 2021-03-29... hemisp_narrowband_filter1 (time) float32 dask.array<chunksize=(4320,), meta=np.ndarray> qc_hemisp_narrowband_filter1 (time) int32 dask.array<chunksize=(4320,), meta=np.ndarray> hemisp_narrowband_filter2 (time) float32 dask.array<chunksize=(4320,), meta=np.ndarray> qc_hemisp_narrowband_filter2 (time) int32 dask.array<chunksize=(4320,), meta=np.ndarray> ... ... nominal_calibration_factor_filter5 float32 ... nominal_calibration_factor_filter6 float32 ... nominal_calibration_factor_filter7 float32 ... lat float32 ... lon float32 ... alt float32 ... Attributes: (12/38) command_line: mfrsr7nch_ingest -s sgp -f E11 Conventions: ARM-1.2 process_version: ingest-mfrsr7nch-1.3-0.el7 dod_version: mfrsr7nch-b1-1.1 input_source: /data/collection/sgp/sgpmfrsr7nchE11.00/MFRS... site_id: sgp ... ... doi: 10.5439/1429369 history: created by user dsmgr on machine flint at 20... _file_dates: ['20210329'] _file_times: ['070000'] _datastream: sgpmfrsr7nchE11.b1 _arm_standards_flag: 1
- time: 4320
- bench_angle: 181
- wavelength: 750
- time(time)datetime64[ns]2021-03-29T07:00:00 ... 2021-03-...
- long_name :
- Time offset from midnight
- standard_name :
- time
array(['2021-03-29T07:00:00.000000000', '2021-03-29T07:00:20.000000000', '2021-03-29T07:00:40.000000000', ..., '2021-03-30T06:59:00.000000000', '2021-03-30T06:59:20.000000000', '2021-03-30T06:59:40.000000000'], dtype='datetime64[ns]')
- bench_angle(bench_angle)float320.0 1.0 2.0 ... 178.0 179.0 180.0
- long_name :
- Angle of incidence during cosine bench measurements
- units :
- degree
- head_orientation :
- Head is oriented so shadowband is south
- South_to_North :
- 0 is S, 90 is zenith, 180 is N
- West_to_East :
- 0 is W, 90 is zenith, 180 is E
array([ 0., 1., 2., 3., 4., 5., 6., 7., 8., 9., 10., 11., 12., 13., 14., 15., 16., 17., 18., 19., 20., 21., 22., 23., 24., 25., 26., 27., 28., 29., 30., 31., 32., 33., 34., 35., 36., 37., 38., 39., 40., 41., 42., 43., 44., 45., 46., 47., 48., 49., 50., 51., 52., 53., 54., 55., 56., 57., 58., 59., 60., 61., 62., 63., 64., 65., 66., 67., 68., 69., 70., 71., 72., 73., 74., 75., 76., 77., 78., 79., 80., 81., 82., 83., 84., 85., 86., 87., 88., 89., 90., 91., 92., 93., 94., 95., 96., 97., 98., 99., 100., 101., 102., 103., 104., 105., 106., 107., 108., 109., 110., 111., 112., 113., 114., 115., 116., 117., 118., 119., 120., 121., 122., 123., 124., 125., 126., 127., 128., 129., 130., 131., 132., 133., 134., 135., 136., 137., 138., 139., 140., 141., 142., 143., 144., 145., 146., 147., 148., 149., 150., 151., 152., 153., 154., 155., 156., 157., 158., 159., 160., 161., 162., 163., 164., 165., 166., 167., 168., 169., 170., 171., 172., 173., 174., 175., 176., 177., 178., 179., 180.], dtype=float32)
- base_time()datetime64[ns]2021-03-29
- string :
- 2021-03-29 00:00:00 0:00
- long_name :
- Base time in Epoch
- ancillary_variables :
- time_offset
array('2021-03-29T00:00:00.000000000', dtype='datetime64[ns]')
- time_offset(time)datetime64[ns]2021-03-29T07:00:00 ... 2021-03-...
- long_name :
- Time offset from base_time
- ancillary_variables :
- base_time
array(['2021-03-29T07:00:00.000000000', '2021-03-29T07:00:20.000000000', '2021-03-29T07:00:40.000000000', ..., '2021-03-30T06:59:00.000000000', '2021-03-30T06:59:20.000000000', '2021-03-30T06:59:40.000000000'], dtype='datetime64[ns]')
- hemisp_narrowband_filter1(time)float32dask.array<chunksize=(4320,), meta=np.ndarray>
- long_name :
- Narrowband hemispheric irradiance, filter 1, offset and cosine corrected
- units :
- W/(m^2 nm)
- valid_min :
- 0.0
- valid_max :
- 1.875
- explanation_of_narrowband_channel :
- The nominal center wavelength is 415 nm, nominal half-power width is 10 nm
- corrections :
- offset subtracted, cosine corrected
- comment :
- sum of diffuse_hemisp_filter1 and direct_horizontal_filter1
- centroid_wavelength :
- 413.3 nm
- FWHM :
- 10.9 nm
- ancillary_variables :
- qc_hemisp_narrowband_filter1
Array Chunk Bytes 16.88 kiB 16.88 kiB Shape (4320,) (4320,) Count 2 Graph Layers 1 Chunks Type float32 numpy.ndarray - qc_hemisp_narrowband_filter1(time)int32dask.array<chunksize=(4320,), meta=np.ndarray>
- long_name :
- Quality check results on field: Narrowband hemispheric irradiance, filter 1, offset and cosine corrected
- units :
- 1
- description :
- See global attributes for individual bit descriptions.
Array Chunk Bytes 16.88 kiB 16.88 kiB Shape (4320,) (4320,) Count 2 Graph Layers 1 Chunks Type int32 numpy.ndarray - hemisp_narrowband_filter2(time)float32dask.array<chunksize=(4320,), meta=np.ndarray>
- long_name :
- Narrowband hemispheric irradiance, filter 2, offset and cosine corrected
- units :
- W/(m^2 nm)
- valid_min :
- 0.0
- valid_max :
- 2.0
- explanation_of_narrowband_channel :
- The nominal center wavelength is 500 nm, nominal half-power width is 10 nm
- corrections :
- offset subtracted, cosine corrected
- comment :
- sum of diffuse_hemisp_filter2 and direct_horizontal_filter2
- centroid_wavelength :
- 501.0 nm
- FWHM :
- 10.8 nm
- ancillary_variables :
- qc_hemisp_narrowband_filter2
Array Chunk Bytes 16.88 kiB 16.88 kiB Shape (4320,) (4320,) Count 2 Graph Layers 1 Chunks Type float32 numpy.ndarray - qc_hemisp_narrowband_filter2(time)int32dask.array<chunksize=(4320,), meta=np.ndarray>
- long_name :
- Quality check results on field: Narrowband hemispheric irradiance, filter 2, offset and cosine corrected
- units :
- 1
- description :
- See global attributes for individual bit descriptions.
Array Chunk Bytes 16.88 kiB 16.88 kiB Shape (4320,) (4320,) Count 2 Graph Layers 1 Chunks Type int32 numpy.ndarray - hemisp_narrowband_filter3(time)float32dask.array<chunksize=(4320,), meta=np.ndarray>
- long_name :
- Narrowband hemispheric irradiance, filter 3, offset and cosine corrected
- units :
- W/(m^2 nm)
- valid_min :
- 0.0
- valid_max :
- 1.875
- explanation_of_narrowband_channel :
- The nominal center wavelength is 615 nm, nominal half-power width is 10 nm
- corrections :
- offset subtracted, cosine corrected
- comment :
- sum of diffuse_hemisp_filter3 and direct_horizontal_filter3
- centroid_wavelength :
- 613.5 nm
- FWHM :
- 10.8 nm
- ancillary_variables :
- qc_hemisp_narrowband_filter3
Array Chunk Bytes 16.88 kiB 16.88 kiB Shape (4320,) (4320,) Count 2 Graph Layers 1 Chunks Type float32 numpy.ndarray - qc_hemisp_narrowband_filter3(time)int32dask.array<chunksize=(4320,), meta=np.ndarray>
- long_name :
- Quality check results on field: Narrowband hemispheric irradiance, filter 3, offset and cosine corrected
- units :
- 1
- description :
- See global attributes for individual bit descriptions.
Array Chunk Bytes 16.88 kiB 16.88 kiB Shape (4320,) (4320,) Count 2 Graph Layers 1 Chunks Type int32 numpy.ndarray - hemisp_narrowband_filter4(time)float32dask.array<chunksize=(4320,), meta=np.ndarray>
- long_name :
- Narrowband hemispheric irradiance, filter 4, offset and cosine corrected
- units :
- W/(m^2 nm)
- valid_min :
- 0.0
- valid_max :
- 1.75
- explanation_of_narrowband_channel :
- The nominal center wavelength is 673 nm, nominal half-power width is 10 nm
- corrections :
- offset subtracted, cosine corrected
- comment :
- sum of diffuse_hemisp_filter4 and direct_horizontal_filter4
- centroid_wavelength :
- 671.4 nm
- FWHM :
- 10.5 nm
- ancillary_variables :
- qc_hemisp_narrowband_filter4
Array Chunk Bytes 16.88 kiB 16.88 kiB Shape (4320,) (4320,) Count 2 Graph Layers 1 Chunks Type float32 numpy.ndarray - qc_hemisp_narrowband_filter4(time)int32dask.array<chunksize=(4320,), meta=np.ndarray>
- long_name :
- Quality check results on field: Narrowband hemispheric irradiance, filter 4, offset and cosine corrected
- units :
- 1
- description :
- See global attributes for individual bit descriptions.
Array Chunk Bytes 16.88 kiB 16.88 kiB Shape (4320,) (4320,) Count 2 Graph Layers 1 Chunks Type int32 numpy.ndarray - hemisp_narrowband_filter5(time)float32dask.array<chunksize=(4320,), meta=np.ndarray>
- long_name :
- Narrowband hemispheric irradiance, filter 5, offset and cosine corrected
- units :
- W/(m^2 nm)
- valid_min :
- 0.0
- valid_max :
- 1.25
- explanation_of_narrowband_channel :
- The nominal center wavelength is 870 nm, nominal half-power width is 10 nm
- corrections :
- offset subtracted, cosine corrected
- comment :
- sum of diffuse_hemisp_filter5 and direct_horizontal_filter5
- centroid_wavelength :
- 869.3 nm
- FWHM :
- 10.0 nm
- ancillary_variables :
- qc_hemisp_narrowband_filter5
Array Chunk Bytes 16.88 kiB 16.88 kiB Shape (4320,) (4320,) Count 2 Graph Layers 1 Chunks Type float32 numpy.ndarray - qc_hemisp_narrowband_filter5(time)int32dask.array<chunksize=(4320,), meta=np.ndarray>
- long_name :
- Quality check results on field: Narrowband hemispheric irradiance, filter 5, offset and cosine corrected
- units :
- 1
- description :
- See global attributes for individual bit descriptions.
Array Chunk Bytes 16.88 kiB 16.88 kiB Shape (4320,) (4320,) Count 2 Graph Layers 1 Chunks Type int32 numpy.ndarray - hemisp_narrowband_filter6(time)float32dask.array<chunksize=(4320,), meta=np.ndarray>
- long_name :
- Narrowband hemispheric irradiance, filter 6, offset and cosine corrected
- units :
- W/(m^2 nm)
- valid_min :
- 0.0
- valid_max :
- 1.5
- explanation_of_narrowband_channel :
- The nominal center wavelength is 940 nm, nominal half-power width is 10 nm
- corrections :
- offset subtracted, cosine corrected
- comment :
- sum of diffuse_hemisp_filter6 and direct_horizontal_filter6
- centroid_wavelength :
- 939.4 nm
- FWHM :
- 6.7 nm
- ancillary_variables :
- qc_hemisp_narrowband_filter6
Array Chunk Bytes 16.88 kiB 16.88 kiB Shape (4320,) (4320,) Count 2 Graph Layers 1 Chunks Type float32 numpy.ndarray - qc_hemisp_narrowband_filter6(time)int32dask.array<chunksize=(4320,), meta=np.ndarray>
- long_name :
- Quality check results on field: Narrowband hemispheric irradiance, filter 6, offset and cosine corrected
- units :
- 1
- description :
- See global attributes for individual bit descriptions.
Array Chunk Bytes 16.88 kiB 16.88 kiB Shape (4320,) (4320,) Count 2 Graph Layers 1 Chunks Type int32 numpy.ndarray - hemisp_narrowband_filter7(time)float32dask.array<chunksize=(4320,), meta=np.ndarray>
- long_name :
- Narrowband hemispheric irradiance, filter 7, offset and cosine corrected
- units :
- W/(m^2 nm)
- valid_min :
- 0.0
- valid_max :
- 5.0
- explanation_of_narrowband_channel :
- The nominal center wavelength is 1625 nm, nominal half-power width is 10 nm
- corrections :
- offset subtracted, cosine corrected
- comment :
- sum of diffuse_hemisp_filter7 and direct_horizontal_filter7
- centroid_wavelength :
- 1624.2 nm
- FWHM :
- 14.8 nm
- ancillary_variables :
- qc_hemisp_narrowband_filter7
Array Chunk Bytes 16.88 kiB 16.88 kiB Shape (4320,) (4320,) Count 2 Graph Layers 1 Chunks Type float32 numpy.ndarray - qc_hemisp_narrowband_filter7(time)int32dask.array<chunksize=(4320,), meta=np.ndarray>
- long_name :
- Quality check results on field: Narrowband hemispheric irradiance, filter 7, offset and cosine corrected
- units :
- 1
- description :
- See global attributes for individual bit descriptions.
Array Chunk Bytes 16.88 kiB 16.88 kiB Shape (4320,) (4320,) Count 2 Graph Layers 1 Chunks Type int32 numpy.ndarray - diffuse_hemisp_narrowband_filter1(time)float32dask.array<chunksize=(4320,), meta=np.ndarray>
- long_name :
- Narrowband diffuse hemispheric irradiance, filter 1, offset and cosine corrected
- units :
- W/(m^2 nm)
- valid_min :
- 0.0
- valid_max :
- 1.875
- explanation_of_narrowband_channel :
- The nominal center wavelength is 415 nm, nominal half-power width is 10 nm
- corrections :
- offset subtracted, cosine corrected
- centroid_wavelength :
- 413.3 nm
- FWHM :
- 10.9 nm
- ancillary_variables :
- qc_diffuse_hemisp_narrowband_filter1
Array Chunk Bytes 16.88 kiB 16.88 kiB Shape (4320,) (4320,) Count 2 Graph Layers 1 Chunks Type float32 numpy.ndarray - qc_diffuse_hemisp_narrowband_filter1(time)int32dask.array<chunksize=(4320,), meta=np.ndarray>
- long_name :
- Quality check results on field: Narrowband diffuse hemispheric irradiance, filter 1, offset and cosine corrected
- units :
- 1
- description :
- See global attributes for individual bit descriptions.
Array Chunk Bytes 16.88 kiB 16.88 kiB Shape (4320,) (4320,) Count 2 Graph Layers 1 Chunks Type int32 numpy.ndarray - diffuse_hemisp_narrowband_filter2(time)float32dask.array<chunksize=(4320,), meta=np.ndarray>
- long_name :
- Narrowband diffuse hemispheric irradiance, filter 2, offset and cosine corrected
- units :
- W/(m^2 nm)
- valid_min :
- 0.0
- valid_max :
- 2.0
- explanation_of_narrowband_channel :
- The nominal center wavelength is 500 nm, nominal half-power width is 10 nm
- corrections :
- offset subtracted, cosine corrected
- centroid_wavelength :
- 501.0 nm
- FWHM :
- 10.8 nm
- ancillary_variables :
- qc_diffuse_hemisp_narrowband_filter2
Array Chunk Bytes 16.88 kiB 16.88 kiB Shape (4320,) (4320,) Count 2 Graph Layers 1 Chunks Type float32 numpy.ndarray - qc_diffuse_hemisp_narrowband_filter2(time)int32dask.array<chunksize=(4320,), meta=np.ndarray>
- long_name :
- Quality check results on field: Narrowband diffuse hemispheric irradiance, filter 2, offset and cosine corrected
- units :
- 1
- description :
- See global attributes for individual bit descriptions.
Array Chunk Bytes 16.88 kiB 16.88 kiB Shape (4320,) (4320,) Count 2 Graph Layers 1 Chunks Type int32 numpy.ndarray - diffuse_hemisp_narrowband_filter3(time)float32dask.array<chunksize=(4320,), meta=np.ndarray>
- long_name :
- Narrowband diffuse hemispheric irradiance, filter 3, offset and cosine corrected
- units :
- W/(m^2 nm)
- valid_min :
- 0.0
- valid_max :
- 1.875
- explanation_of_narrowband_channel :
- The nominal center wavelength is 615 nm, nominal half-power width is 10 nm
- corrections :
- offset subtracted, cosine corrected
- centroid_wavelength :
- 613.5 nm
- FWHM :
- 10.8 nm
- ancillary_variables :
- qc_diffuse_hemisp_narrowband_filter3
Array Chunk Bytes 16.88 kiB 16.88 kiB Shape (4320,) (4320,) Count 2 Graph Layers 1 Chunks Type float32 numpy.ndarray - qc_diffuse_hemisp_narrowband_filter3(time)int32dask.array<chunksize=(4320,), meta=np.ndarray>
- long_name :
- Quality check results on field: Narrowband diffuse hemispheric irradiance, filter 3, offset and cosine corrected
- units :
- 1
- description :
- See global attributes for individual bit descriptions.
Array Chunk Bytes 16.88 kiB 16.88 kiB Shape (4320,) (4320,) Count 2 Graph Layers 1 Chunks Type int32 numpy.ndarray - diffuse_hemisp_narrowband_filter4(time)float32dask.array<chunksize=(4320,), meta=np.ndarray>
- long_name :
- Narrowband diffuse hemispheric irradiance, filter 4, offset and cosine corrected
- units :
- W/(m^2 nm)
- valid_min :
- 0.0
- valid_max :
- 1.75
- explanation_of_narrowband_channel :
- The nominal center wavelength is 673 nm, nominal half-power width is 10 nm
- corrections :
- offset subtracted, cosine corrected
- centroid_wavelength :
- 671.4 nm
- FWHM :
- 10.5 nm
- ancillary_variables :
- qc_diffuse_hemisp_narrowband_filter4
Array Chunk Bytes 16.88 kiB 16.88 kiB Shape (4320,) (4320,) Count 2 Graph Layers 1 Chunks Type float32 numpy.ndarray - qc_diffuse_hemisp_narrowband_filter4(time)int32dask.array<chunksize=(4320,), meta=np.ndarray>
- long_name :
- Quality check results on field: Narrowband diffuse hemispheric irradiance, filter 4, offset and cosine corrected
- units :
- 1
- description :
- See global attributes for individual bit descriptions.
Array Chunk Bytes 16.88 kiB 16.88 kiB Shape (4320,) (4320,) Count 2 Graph Layers 1 Chunks Type int32 numpy.ndarray - diffuse_hemisp_narrowband_filter5(time)float32dask.array<chunksize=(4320,), meta=np.ndarray>
- long_name :
- Narrowband diffuse hemispheric irradiance, filter 5, offset and cosine corrected
- units :
- W/(m^2 nm)
- valid_min :
- 0.0
- valid_max :
- 1.25
- explanation_of_narrowband_channel :
- The nominal center wavelength is 870 nm, nominal half-power width is 10 nm
- corrections :
- offset subtracted, cosine corrected
- centroid_wavelength :
- 869.3 nm
- FWHM :
- 10.0 nm
- ancillary_variables :
- qc_diffuse_hemisp_narrowband_filter5
Array Chunk Bytes 16.88 kiB 16.88 kiB Shape (4320,) (4320,) Count 2 Graph Layers 1 Chunks Type float32 numpy.ndarray - qc_diffuse_hemisp_narrowband_filter5(time)int32dask.array<chunksize=(4320,), meta=np.ndarray>
- long_name :
- Quality check results on field: Narrowband diffuse hemispheric irradiance, filter 5, offset and cosine corrected
- units :
- 1
- description :
- See global attributes for individual bit descriptions.
Array Chunk Bytes 16.88 kiB 16.88 kiB Shape (4320,) (4320,) Count 2 Graph Layers 1 Chunks Type int32 numpy.ndarray - diffuse_hemisp_narrowband_filter6(time)float32dask.array<chunksize=(4320,), meta=np.ndarray>
- long_name :
- Narrowband diffuse hemispheric irradiance, filter 6, offset and cosine corrected
- units :
- W/(m^2 nm)
- valid_min :
- 0.0
- valid_max :
- 1.5
- explanation_of_narrowband_channel :
- The nominal center wavelength is 940 nm, nominal half-power width is 10 nm
- corrections :
- offset subtracted, cosine corrected
- centroid_wavelength :
- 939.4 nm
- FWHM :
- 6.7 nm
- ancillary_variables :
- qc_diffuse_hemisp_narrowband_filter6
Array Chunk Bytes 16.88 kiB 16.88 kiB Shape (4320,) (4320,) Count 2 Graph Layers 1 Chunks Type float32 numpy.ndarray - qc_diffuse_hemisp_narrowband_filter6(time)int32dask.array<chunksize=(4320,), meta=np.ndarray>
- long_name :
- Quality check results on field: Narrowband diffuse hemispheric irradiance, filter 6, offset and cosine corrected
- units :
- 1
- description :
- See global attributes for individual bit descriptions.
Array Chunk Bytes 16.88 kiB 16.88 kiB Shape (4320,) (4320,) Count 2 Graph Layers 1 Chunks Type int32 numpy.ndarray - diffuse_hemisp_narrowband_filter7(time)float32dask.array<chunksize=(4320,), meta=np.ndarray>
- long_name :
- Narrowband diffuse hemispheric irradiance, filter 7, offset and cosine corrected
- units :
- W/(m^2 nm)
- valid_min :
- 0.0
- valid_max :
- 5.0
- explanation_of_narrowband_channel :
- The nominal center wavelength is 1625 nm, nominal half-power width is 10 nm
- corrections :
- offset subtracted, cosine corrected
- centroid_wavelength :
- 1624.2 nm
- FWHM :
- 14.8 nm
- ancillary_variables :
- qc_diffuse_hemisp_narrowband_filter7
Array Chunk Bytes 16.88 kiB 16.88 kiB Shape (4320,) (4320,) Count 2 Graph Layers 1 Chunks Type float32 numpy.ndarray - qc_diffuse_hemisp_narrowband_filter7(time)int32dask.array<chunksize=(4320,), meta=np.ndarray>
- long_name :
- Quality check results on field: Narrowband diffuse hemispheric irradiance, filter 7, offset and cosine corrected
- units :
- 1
- description :
- See global attributes for individual bit descriptions.
Array Chunk Bytes 16.88 kiB 16.88 kiB Shape (4320,) (4320,) Count 2 Graph Layers 1 Chunks Type int32 numpy.ndarray - direct_normal_narrowband_filter1(time)float32dask.array<chunksize=(4320,), meta=np.ndarray>
- long_name :
- Narrowband direct normal irradiance, filter 1, cosine corrected
- units :
- W/(m^2 nm)
- valid_min :
- 0.0
- valid_max :
- 1.875
- explanation_of_narrowband_channel :
- The nominal center wavelength is 415 nm, nominal half-power width is 10 nm
- corrections :
- cosine corrected
- centroid_wavelength :
- 413.3 nm
- FWHM :
- 10.9 nm
- ancillary_variables :
- qc_direct_normal_narrowband_filter1
Array Chunk Bytes 16.88 kiB 16.88 kiB Shape (4320,) (4320,) Count 2 Graph Layers 1 Chunks Type float32 numpy.ndarray - qc_direct_normal_narrowband_filter1(time)int32dask.array<chunksize=(4320,), meta=np.ndarray>
- long_name :
- Quality check results on field: Narrowband direct normal irradiance, filter 1, cosine corrected
- units :
- 1
- description :
- See global attributes for individual bit descriptions.
Array Chunk Bytes 16.88 kiB 16.88 kiB Shape (4320,) (4320,) Count 2 Graph Layers 1 Chunks Type int32 numpy.ndarray - direct_normal_narrowband_filter2(time)float32dask.array<chunksize=(4320,), meta=np.ndarray>
- long_name :
- Narrowband direct normal irradiance, filter 2, cosine corrected
- units :
- W/(m^2 nm)
- valid_min :
- 0.0
- valid_max :
- 2.0
- explanation_of_narrowband_channel :
- The nominal center wavelength is 500 nm, nominal half-power width is 10 nm
- corrections :
- cosine corrected
- centroid_wavelength :
- 501.0 nm
- FWHM :
- 10.8 nm
- ancillary_variables :
- qc_direct_normal_narrowband_filter2
Array Chunk Bytes 16.88 kiB 16.88 kiB Shape (4320,) (4320,) Count 2 Graph Layers 1 Chunks Type float32 numpy.ndarray - qc_direct_normal_narrowband_filter2(time)int32dask.array<chunksize=(4320,), meta=np.ndarray>
- long_name :
- Quality check results on field: Narrowband direct normal irradiance, filter 2, cosine corrected
- units :
- 1
- description :
- See global attributes for individual bit descriptions.
Array Chunk Bytes 16.88 kiB 16.88 kiB Shape (4320,) (4320,) Count 2 Graph Layers 1 Chunks Type int32 numpy.ndarray - direct_normal_narrowband_filter3(time)float32dask.array<chunksize=(4320,), meta=np.ndarray>
- long_name :
- Narrowband direct normal irradiance, filter 3, cosine corrected
- units :
- W/(m^2 nm)
- valid_min :
- 0.0
- valid_max :
- 1.875
- explanation_of_narrowband_channel :
- The nominal center wavelength is 615 nm, nominal half-power width is 10 nm
- corrections :
- cosine corrected
- centroid_wavelength :
- 613.5 nm
- FWHM :
- 10.8 nm
- ancillary_variables :
- qc_direct_normal_narrowband_filter3
Array Chunk Bytes 16.88 kiB 16.88 kiB Shape (4320,) (4320,) Count 2 Graph Layers 1 Chunks Type float32 numpy.ndarray - qc_direct_normal_narrowband_filter3(time)int32dask.array<chunksize=(4320,), meta=np.ndarray>
- long_name :
- Quality check results on field: Narrowband direct normal irradiance, filter 3, cosine corrected
- units :
- 1
- description :
- See global attributes for individual bit descriptions.
Array Chunk Bytes 16.88 kiB 16.88 kiB Shape (4320,) (4320,) Count 2 Graph Layers 1 Chunks Type int32 numpy.ndarray - direct_normal_narrowband_filter4(time)float32dask.array<chunksize=(4320,), meta=np.ndarray>
- long_name :
- Narrowband direct normal irradiance, filter 4, cosine corrected
- units :
- W/(m^2 nm)
- valid_min :
- 0.0
- valid_max :
- 1.75
- explanation_of_narrowband_channel :
- The nominal center wavelength is 673 nm, nominal half-power width is 10 nm
- corrections :
- cosine corrected
- centroid_wavelength :
- 671.4 nm
- FWHM :
- 10.5 nm
- ancillary_variables :
- qc_direct_normal_narrowband_filter4
Array Chunk Bytes 16.88 kiB 16.88 kiB Shape (4320,) (4320,) Count 2 Graph Layers 1 Chunks Type float32 numpy.ndarray - qc_direct_normal_narrowband_filter4(time)int32dask.array<chunksize=(4320,), meta=np.ndarray>
- long_name :
- Quality check results on field: Narrowband direct normal irradiance, filter 4, cosine corrected
- units :
- 1
- description :
- See global attributes for individual bit descriptions.
Array Chunk Bytes 16.88 kiB 16.88 kiB Shape (4320,) (4320,) Count 2 Graph Layers 1 Chunks Type int32 numpy.ndarray - direct_normal_narrowband_filter5(time)float32dask.array<chunksize=(4320,), meta=np.ndarray>
- long_name :
- Narrowband direct normal irradiance, filter 5, cosine corrected
- units :
- W/(m^2 nm)
- valid_min :
- 0.0
- valid_max :
- 1.25
- explanation_of_narrowband_channel :
- The nominal center wavelength is 870 nm, nominal half-power width is 10 nm
- corrections :
- cosine corrected
- centroid_wavelength :
- 869.3 nm
- FWHM :
- 10.0 nm
- ancillary_variables :
- qc_direct_normal_narrowband_filter5
Array Chunk Bytes 16.88 kiB 16.88 kiB Shape (4320,) (4320,) Count 2 Graph Layers 1 Chunks Type float32 numpy.ndarray - qc_direct_normal_narrowband_filter5(time)int32dask.array<chunksize=(4320,), meta=np.ndarray>
- long_name :
- Quality check results on field: Narrowband direct normal irradiance, filter 5, cosine corrected
- units :
- 1
- description :
- See global attributes for individual bit descriptions.
Array Chunk Bytes 16.88 kiB 16.88 kiB Shape (4320,) (4320,) Count 2 Graph Layers 1 Chunks Type int32 numpy.ndarray - direct_normal_narrowband_filter6(time)float32dask.array<chunksize=(4320,), meta=np.ndarray>
- long_name :
- Narrowband direct normal irradiance, filter 6, cosine corrected
- units :
- W/(m^2 nm)
- valid_min :
- 0.0
- valid_max :
- 1.5
- explanation_of_narrowband_channel :
- The nominal center wavelength is 940 nm, nominal half-power width is 10 nm
- corrections :
- cosine corrected
- centroid_wavelength :
- 939.4 nm
- FWHM :
- 6.7 nm
- ancillary_variables :
- qc_direct_normal_narrowband_filter6
Array Chunk Bytes 16.88 kiB 16.88 kiB Shape (4320,) (4320,) Count 2 Graph Layers 1 Chunks Type float32 numpy.ndarray - qc_direct_normal_narrowband_filter6(time)int32dask.array<chunksize=(4320,), meta=np.ndarray>
- long_name :
- Quality check results on field: Narrowband direct normal irradiance, filter 6, cosine corrected
- units :
- 1
- description :
- See global attributes for individual bit descriptions.
Array Chunk Bytes 16.88 kiB 16.88 kiB Shape (4320,) (4320,) Count 2 Graph Layers 1 Chunks Type int32 numpy.ndarray - direct_normal_narrowband_filter7(time)float32dask.array<chunksize=(4320,), meta=np.ndarray>
- long_name :
- Narrowband direct normal irradiance, filter 7, cosine corrected
- units :
- W/(m^2 nm)
- valid_min :
- 0.0
- valid_max :
- 5.0
- explanation_of_narrowband_channel :
- The nominal center wavelength is 1625 nm, nominal half-power width is 10 nm
- corrections :
- cosine corrected
- centroid_wavelength :
- 1624.2 nm
- FWHM :
- 14.8 nm
- ancillary_variables :
- qc_direct_normal_narrowband_filter7
Array Chunk Bytes 16.88 kiB 16.88 kiB Shape (4320,) (4320,) Count 2 Graph Layers 1 Chunks Type float32 numpy.ndarray - qc_direct_normal_narrowband_filter7(time)int32dask.array<chunksize=(4320,), meta=np.ndarray>
- long_name :
- Quality check results on field: Narrowband direct normal irradiance, filter 7, cosine corrected
- units :
- 1
- description :
- See global attributes for individual bit descriptions.
Array Chunk Bytes 16.88 kiB 16.88 kiB Shape (4320,) (4320,) Count 2 Graph Layers 1 Chunks Type int32 numpy.ndarray - alltime_hemisp_narrowband_filter1(time)float32dask.array<chunksize=(4320,), meta=np.ndarray>
- long_name :
- Alltime narrowband hemispheric irradiance, filter 1
- units :
- mV
- valid_min :
- -50.0
- valid_max :
- 4095.0
- explanation_of_narrowband_channel :
- The nominal center wavelength is 415 nm, nominal half-power width is 10 nm
- corrections :
- none
- centroid_wavelength :
- 413.3 nm
- FWHM :
- 10.9 nm
- ancillary_variables :
- qc_alltime_hemisp_narrowband_filter1
Array Chunk Bytes 16.88 kiB 16.88 kiB Shape (4320,) (4320,) Count 2 Graph Layers 1 Chunks Type float32 numpy.ndarray - qc_alltime_hemisp_narrowband_filter1(time)int32dask.array<chunksize=(4320,), meta=np.ndarray>
- long_name :
- Quality check results on field: Alltime narrowband hemispheric irradiance, filter 1
- units :
- 1
- description :
- See global attributes for individual bit descriptions.
Array Chunk Bytes 16.88 kiB 16.88 kiB Shape (4320,) (4320,) Count 2 Graph Layers 1 Chunks Type int32 numpy.ndarray - alltime_hemisp_narrowband_filter2(time)float32dask.array<chunksize=(4320,), meta=np.ndarray>
- long_name :
- Alltime narrowband hemispheric irradiance, filter 2
- units :
- mV
- valid_min :
- -50.0
- valid_max :
- 4095.0
- explanation_of_narrowband_channel :
- The nominal center wavelength is 500 nm, nominal half-power width is 10 nm
- corrections :
- none
- centroid_wavelength :
- 501.0 nm
- FWHM :
- 10.8 nm
- ancillary_variables :
- qc_alltime_hemisp_narrowband_filter2
Array Chunk Bytes 16.88 kiB 16.88 kiB Shape (4320,) (4320,) Count 2 Graph Layers 1 Chunks Type float32 numpy.ndarray - qc_alltime_hemisp_narrowband_filter2(time)int32dask.array<chunksize=(4320,), meta=np.ndarray>
- long_name :
- Quality check results on field: Alltime narrowband hemispheric irradiance, filter 2
- units :
- 1
- description :
- See global attributes for individual bit descriptions.
Array Chunk Bytes 16.88 kiB 16.88 kiB Shape (4320,) (4320,) Count 2 Graph Layers 1 Chunks Type int32 numpy.ndarray - alltime_hemisp_narrowband_filter3(time)float32dask.array<chunksize=(4320,), meta=np.ndarray>
- long_name :
- Alltime narrowband hemispheric irradiance, filter 3
- units :
- mV
- valid_min :
- -50.0
- valid_max :
- 4095.0
- explanation_of_narrowband_channel :
- The nominal center wavelength is 615 nm, nominal half-power width is 10 nm
- corrections :
- none
- centroid_wavelength :
- 613.5 nm
- FWHM :
- 10.8 nm
- ancillary_variables :
- qc_alltime_hemisp_narrowband_filter3
Array Chunk Bytes 16.88 kiB 16.88 kiB Shape (4320,) (4320,) Count 2 Graph Layers 1 Chunks Type float32 numpy.ndarray - qc_alltime_hemisp_narrowband_filter3(time)int32dask.array<chunksize=(4320,), meta=np.ndarray>
- long_name :
- Quality check results on field: Alltime narrowband hemispheric irradiance, filter 3
- units :
- 1
- description :
- See global attributes for individual bit descriptions.
Array Chunk Bytes 16.88 kiB 16.88 kiB Shape (4320,) (4320,) Count 2 Graph Layers 1 Chunks Type int32 numpy.ndarray - alltime_hemisp_narrowband_filter4(time)float32dask.array<chunksize=(4320,), meta=np.ndarray>
- long_name :
- Alltime narrowband hemispheric irradiance, filter 4
- units :
- mV
- valid_min :
- -50.0
- valid_max :
- 4095.0
- explanation_of_narrowband_channel :
- The nominal center wavelength is 673 nm, nominal half-power width is 10 nm
- corrections :
- none
- centroid_wavelength :
- 671.4 nm
- FWHM :
- 10.5 nm
- ancillary_variables :
- qc_alltime_hemisp_narrowband_filter4
Array Chunk Bytes 16.88 kiB 16.88 kiB Shape (4320,) (4320,) Count 2 Graph Layers 1 Chunks Type float32 numpy.ndarray - qc_alltime_hemisp_narrowband_filter4(time)int32dask.array<chunksize=(4320,), meta=np.ndarray>
- long_name :
- Quality check results on field: Alltime narrowband hemispheric irradiance, filter 4
- units :
- 1
- description :
- See global attributes for individual bit descriptions.
Array Chunk Bytes 16.88 kiB 16.88 kiB Shape (4320,) (4320,) Count 2 Graph Layers 1 Chunks Type int32 numpy.ndarray - alltime_hemisp_narrowband_filter5(time)float32dask.array<chunksize=(4320,), meta=np.ndarray>
- long_name :
- Alltime narrowband hemispheric irradiance, filter 5
- units :
- mV
- valid_min :
- -50.0
- valid_max :
- 4095.0
- explanation_of_narrowband_channel :
- The nominal center wavelength is 870 nm, nominal half-power width is 10 nm
- corrections :
- none
- centroid_wavelength :
- 869.3 nm
- FWHM :
- 10.0 nm
- ancillary_variables :
- qc_alltime_hemisp_narrowband_filter5
Array Chunk Bytes 16.88 kiB 16.88 kiB Shape (4320,) (4320,) Count 2 Graph Layers 1 Chunks Type float32 numpy.ndarray - qc_alltime_hemisp_narrowband_filter5(time)int32dask.array<chunksize=(4320,), meta=np.ndarray>
- long_name :
- Quality check results on field: Alltime narrowband hemispheric irradiance, filter 5
- units :
- 1
- description :
- See global attributes for individual bit descriptions.
Array Chunk Bytes 16.88 kiB 16.88 kiB Shape (4320,) (4320,) Count 2 Graph Layers 1 Chunks Type int32 numpy.ndarray - alltime_hemisp_narrowband_filter6(time)float32dask.array<chunksize=(4320,), meta=np.ndarray>
- long_name :
- Alltime narrowband hemispheric irradiance, filter 6
- units :
- mV
- valid_min :
- -50.0
- valid_max :
- 4095.0
- explanation_of_narrowband_channel :
- The nominal center wavelength is 940 nm, nominal half-power width is 10 nm
- corrections :
- none
- centroid_wavelength :
- 939.4 nm
- FWHM :
- 6.7 nm
- ancillary_variables :
- qc_alltime_hemisp_narrowband_filter6
Array Chunk Bytes 16.88 kiB 16.88 kiB Shape (4320,) (4320,) Count 2 Graph Layers 1 Chunks Type float32 numpy.ndarray - qc_alltime_hemisp_narrowband_filter6(time)int32dask.array<chunksize=(4320,), meta=np.ndarray>
- long_name :
- Quality check results on field: Alltime narrowband hemispheric irradiance, filter 6
- units :
- 1
- description :
- See global attributes for individual bit descriptions.
Array Chunk Bytes 16.88 kiB 16.88 kiB Shape (4320,) (4320,) Count 2 Graph Layers 1 Chunks Type int32 numpy.ndarray - alltime_hemisp_narrowband_filter7(time)float32dask.array<chunksize=(4320,), meta=np.ndarray>
- long_name :
- Alltime narrowband hemispheric irradiance, filter 7
- units :
- mV
- valid_min :
- -50.0
- valid_max :
- 4095.0
- explanation_of_narrowband_channel :
- The nominal center wavelength is 1625 nm, nominal half-power width is 10 nm
- corrections :
- none
- centroid_wavelength :
- 1624.2 nm
- FWHM :
- 14.8 nm
- ancillary_variables :
- qc_alltime_hemisp_narrowband_filter7
Array Chunk Bytes 16.88 kiB 16.88 kiB Shape (4320,) (4320,) Count 2 Graph Layers 1 Chunks Type float32 numpy.ndarray - qc_alltime_hemisp_narrowband_filter7(time)int32dask.array<chunksize=(4320,), meta=np.ndarray>
- long_name :
- Quality check results on field: Alltime narrowband hemispheric irradiance, filter 7
- units :
- 1
- description :
- See global attributes for individual bit descriptions.
Array Chunk Bytes 16.88 kiB 16.88 kiB Shape (4320,) (4320,) Count 2 Graph Layers 1 Chunks Type int32 numpy.ndarray - direct_horizontal_narrowband_filter1(time)float32dask.array<chunksize=(4320,), meta=np.ndarray>
- long_name :
- Narrowband direct horizontal irradiance, filter 1, cosine corrected
- units :
- W/(m^2 nm)
- valid_min :
- 0.0
- valid_max :
- 1.875
- explanation_of_narrowband_channel :
- The nominal center wavelength is 415 nm, nominal half-power width is 10 nm
- corrections :
- cosine corrected
- centroid_wavelength :
- 413.3 nm
- FWHM :
- 10.9 nm
- ancillary_variables :
- qc_direct_horizontal_narrowband_filter1
Array Chunk Bytes 16.88 kiB 16.88 kiB Shape (4320,) (4320,) Count 2 Graph Layers 1 Chunks Type float32 numpy.ndarray - qc_direct_horizontal_narrowband_filter1(time)int32dask.array<chunksize=(4320,), meta=np.ndarray>
- long_name :
- Quality check results on field: Narrowband direct horizontal irradiance, filter 1, cosine corrected
- units :
- 1
- description :
- See global attributes for individual bit descriptions.
Array Chunk Bytes 16.88 kiB 16.88 kiB Shape (4320,) (4320,) Count 2 Graph Layers 1 Chunks Type int32 numpy.ndarray - direct_horizontal_narrowband_filter2(time)float32dask.array<chunksize=(4320,), meta=np.ndarray>
- long_name :
- Narrowband direct horizontal irradiance, filter 2, cosine corrected
- units :
- W/(m^2 nm)
- valid_min :
- 0.0
- valid_max :
- 2.0
- explanation_of_narrowband_channel :
- The nominal center wavelength is 500 nm, nominal half-power width is 10 nm
- corrections :
- cosine corrected
- centroid_wavelength :
- 501.0 nm
- FWHM :
- 10.8 nm
- ancillary_variables :
- qc_direct_horizontal_narrowband_filter2
Array Chunk Bytes 16.88 kiB 16.88 kiB Shape (4320,) (4320,) Count 2 Graph Layers 1 Chunks Type float32 numpy.ndarray - qc_direct_horizontal_narrowband_filter2(time)int32dask.array<chunksize=(4320,), meta=np.ndarray>
- long_name :
- Quality check results on field: Narrowband direct horizontal irradiance, filter 2, cosine corrected
- units :
- 1
- description :
- See global attributes for individual bit descriptions.
Array Chunk Bytes 16.88 kiB 16.88 kiB Shape (4320,) (4320,) Count 2 Graph Layers 1 Chunks Type int32 numpy.ndarray - direct_horizontal_narrowband_filter3(time)float32dask.array<chunksize=(4320,), meta=np.ndarray>
- long_name :
- Narrowband direct horizontal irradiance, filter 3, cosine corrected
- units :
- W/(m^2 nm)
- valid_min :
- 0.0
- valid_max :
- 1.875
- explanation_of_narrowband_channel :
- The nominal center wavelength is 615 nm, nominal half-power width is 10 nm
- corrections :
- cosine corrected
- centroid_wavelength :
- 613.5 nm
- FWHM :
- 10.8 nm
- ancillary_variables :
- qc_direct_horizontal_narrowband_filter3
Array Chunk Bytes 16.88 kiB 16.88 kiB Shape (4320,) (4320,) Count 2 Graph Layers 1 Chunks Type float32 numpy.ndarray - qc_direct_horizontal_narrowband_filter3(time)int32dask.array<chunksize=(4320,), meta=np.ndarray>
- long_name :
- Quality check results on field: Narrowband direct horizontal irradiance, filter 3, cosine corrected
- units :
- 1
- description :
- See global attributes for individual bit descriptions.
Array Chunk Bytes 16.88 kiB 16.88 kiB Shape (4320,) (4320,) Count 2 Graph Layers 1 Chunks Type int32 numpy.ndarray - direct_horizontal_narrowband_filter4(time)float32dask.array<chunksize=(4320,), meta=np.ndarray>
- long_name :
- Narrowband direct horizontal irradiance, filter 4, cosine corrected
- units :
- W/(m^2 nm)
- valid_min :
- 0.0
- valid_max :
- 1.75
- explanation_of_narrowband_channel :
- The nominal center wavelength is 673 nm, nominal half-power width is 10 nm
- corrections :
- cosine corrected
- centroid_wavelength :
- 671.4 nm
- FWHM :
- 10.5 nm
- ancillary_variables :
- qc_direct_horizontal_narrowband_filter4
Array Chunk Bytes 16.88 kiB 16.88 kiB Shape (4320,) (4320,) Count 2 Graph Layers 1 Chunks Type float32 numpy.ndarray - qc_direct_horizontal_narrowband_filter4(time)int32dask.array<chunksize=(4320,), meta=np.ndarray>
- long_name :
- Quality check results on field: Narrowband direct horizontal irradiance, filter 4, cosine corrected
- units :
- 1
- description :
- See global attributes for individual bit descriptions.
Array Chunk Bytes 16.88 kiB 16.88 kiB Shape (4320,) (4320,) Count 2 Graph Layers 1 Chunks Type int32 numpy.ndarray - direct_horizontal_narrowband_filter5(time)float32dask.array<chunksize=(4320,), meta=np.ndarray>
- long_name :
- Narrowband direct horizontal irradiance, filter 5, cosine corrected
- units :
- W/(m^2 nm)
- valid_min :
- 0.0
- valid_max :
- 1.25
- explanation_of_narrowband_channel :
- The nominal center wavelength is 870 nm, nominal half-power width is 10 nm
- corrections :
- cosine corrected
- centroid_wavelength :
- 869.3 nm
- FWHM :
- 10.0 nm
- ancillary_variables :
- qc_direct_horizontal_narrowband_filter5
Array Chunk Bytes 16.88 kiB 16.88 kiB Shape (4320,) (4320,) Count 2 Graph Layers 1 Chunks Type float32 numpy.ndarray - qc_direct_horizontal_narrowband_filter5(time)int32dask.array<chunksize=(4320,), meta=np.ndarray>
- long_name :
- Quality check results on field: Narrowband direct horizontal irradiance, filter 5, cosine corrected
- units :
- 1
- description :
- See global attributes for individual bit descriptions.
Array Chunk Bytes 16.88 kiB 16.88 kiB Shape (4320,) (4320,) Count 2 Graph Layers 1 Chunks Type int32 numpy.ndarray - direct_horizontal_narrowband_filter6(time)float32dask.array<chunksize=(4320,), meta=np.ndarray>
- long_name :
- Narrowband direct horizontal irradiance, filter 6, cosine corrected
- units :
- W/(m^2 nm)
- valid_min :
- 0.0
- valid_max :
- 1.5
- explanation_of_narrowband_channel :
- The nominal center wavelength is 940 nm, nominal half-power width is 10 nm
- corrections :
- cosine corrected
- centroid_wavelength :
- 939.4 nm
- FWHM :
- 6.7 nm
- ancillary_variables :
- qc_direct_horizontal_narrowband_filter6
Array Chunk Bytes 16.88 kiB 16.88 kiB Shape (4320,) (4320,) Count 2 Graph Layers 1 Chunks Type float32 numpy.ndarray - qc_direct_horizontal_narrowband_filter6(time)int32dask.array<chunksize=(4320,), meta=np.ndarray>
- long_name :
- Quality check results on field: Narrowband direct horizontal irradiance, filter 6, cosine corrected
- units :
- 1
- description :
- See global attributes for individual bit descriptions.
Array Chunk Bytes 16.88 kiB 16.88 kiB Shape (4320,) (4320,) Count 2 Graph Layers 1 Chunks Type int32 numpy.ndarray - direct_horizontal_narrowband_filter7(time)float32dask.array<chunksize=(4320,), meta=np.ndarray>
- long_name :
- Narrowband direct horizontal irradiance, filter 7, cosine corrected
- units :
- W/(m^2 nm)
- valid_min :
- 0.0
- valid_max :
- 5.0
- explanation_of_narrowband_channel :
- The nominal center wavelength is 1625 nm, nominal half-power width is 10 nm
- corrections :
- cosine corrected
- centroid_wavelength :
- 1624.2 nm
- FWHM :
- 14.8 nm
- ancillary_variables :
- qc_direct_horizontal_narrowband_filter7
Array Chunk Bytes 16.88 kiB 16.88 kiB Shape (4320,) (4320,) Count 2 Graph Layers 1 Chunks Type float32 numpy.ndarray - qc_direct_horizontal_narrowband_filter7(time)int32dask.array<chunksize=(4320,), meta=np.ndarray>
- long_name :
- Quality check results on field: Narrowband direct horizontal irradiance, filter 7, cosine corrected
- units :
- 1
- description :
- See global attributes for individual bit descriptions.
Array Chunk Bytes 16.88 kiB 16.88 kiB Shape (4320,) (4320,) Count 2 Graph Layers 1 Chunks Type int32 numpy.ndarray - direct_diffuse_ratio_filter1(time)float32dask.array<chunksize=(4320,), meta=np.ndarray>
- long_name :
- Ratio of direct_normal_filter1 to diffuse_hemisp_filter1
- units :
- 1
- valid_min :
- 0.0
- valid_max :
- 5.0
- centroid_wavelength :
- 413.3 nm
- FWHM :
- 10.9 nm
- ancillary_variables :
- qc_direct_diffuse_ratio_filter1
Array Chunk Bytes 16.88 kiB 16.88 kiB Shape (4320,) (4320,) Count 2 Graph Layers 1 Chunks Type float32 numpy.ndarray - qc_direct_diffuse_ratio_filter1(time)int32dask.array<chunksize=(4320,), meta=np.ndarray>
- long_name :
- Quality check results on field: Ratio of direct_normal_filter1 to diffuse_hemisp_filter1
- units :
- 1
- description :
- See global attributes for individual bit descriptions.
Array Chunk Bytes 16.88 kiB 16.88 kiB Shape (4320,) (4320,) Count 2 Graph Layers 1 Chunks Type int32 numpy.ndarray - direct_diffuse_ratio_filter2(time)float32dask.array<chunksize=(4320,), meta=np.ndarray>
- long_name :
- Ratio of direct_normal_filter2 to diffuse_hemisp_filter2
- units :
- 1
- valid_min :
- 0.0
- valid_max :
- 6.0
- centroid_wavelength :
- 501.0 nm
- FWHM :
- 10.8 nm
- ancillary_variables :
- qc_direct_diffuse_ratio_filter2
Array Chunk Bytes 16.88 kiB 16.88 kiB Shape (4320,) (4320,) Count 2 Graph Layers 1 Chunks Type float32 numpy.ndarray - qc_direct_diffuse_ratio_filter2(time)int32dask.array<chunksize=(4320,), meta=np.ndarray>
- long_name :
- Quality check results on field: Ratio of direct_normal_filter2 to diffuse_hemisp_filter2
- units :
- 1
- description :
- See global attributes for individual bit descriptions.
Array Chunk Bytes 16.88 kiB 16.88 kiB Shape (4320,) (4320,) Count 2 Graph Layers 1 Chunks Type int32 numpy.ndarray - direct_diffuse_ratio_filter3(time)float32dask.array<chunksize=(4320,), meta=np.ndarray>
- long_name :
- Ratio of direct_normal_filter3 to diffuse_hemisp_filter3
- units :
- 1
- valid_min :
- 0.0
- valid_max :
- 10.0
- centroid_wavelength :
- 613.5 nm
- FWHM :
- 10.8 nm
- ancillary_variables :
- qc_direct_diffuse_ratio_filter3
Array Chunk Bytes 16.88 kiB 16.88 kiB Shape (4320,) (4320,) Count 2 Graph Layers 1 Chunks Type float32 numpy.ndarray - qc_direct_diffuse_ratio_filter3(time)int32dask.array<chunksize=(4320,), meta=np.ndarray>
- long_name :
- Quality check results on field: Ratio of direct_normal_filter3 to diffuse_hemisp_filter3
- units :
- 1
- description :
- See global attributes for individual bit descriptions.
Array Chunk Bytes 16.88 kiB 16.88 kiB Shape (4320,) (4320,) Count 2 Graph Layers 1 Chunks Type int32 numpy.ndarray - direct_diffuse_ratio_filter4(time)float32dask.array<chunksize=(4320,), meta=np.ndarray>
- long_name :
- Ratio of direct_normal_filter4 to diffuse_hemisp_filter4
- units :
- 1
- valid_min :
- 0.0
- valid_max :
- 14.0
- centroid_wavelength :
- 671.4 nm
- FWHM :
- 10.5 nm
- ancillary_variables :
- qc_direct_diffuse_ratio_filter4
Array Chunk Bytes 16.88 kiB 16.88 kiB Shape (4320,) (4320,) Count 2 Graph Layers 1 Chunks Type float32 numpy.ndarray - qc_direct_diffuse_ratio_filter4(time)int32dask.array<chunksize=(4320,), meta=np.ndarray>
- long_name :
- Quality check results on field: Ratio of direct_normal_filter4 to diffuse_hemisp_filter4
- units :
- 1
- description :
- See global attributes for individual bit descriptions.
Array Chunk Bytes 16.88 kiB 16.88 kiB Shape (4320,) (4320,) Count 2 Graph Layers 1 Chunks Type int32 numpy.ndarray - direct_diffuse_ratio_filter5(time)float32dask.array<chunksize=(4320,), meta=np.ndarray>
- long_name :
- Ratio of direct_normal_filter5 to diffuse_hemisp_filter5
- units :
- 1
- valid_min :
- 0.0
- valid_max :
- 25.0
- centroid_wavelength :
- 869.3 nm
- FWHM :
- 10.0 nm
- ancillary_variables :
- qc_direct_diffuse_ratio_filter5
Array Chunk Bytes 16.88 kiB 16.88 kiB Shape (4320,) (4320,) Count 2 Graph Layers 1 Chunks Type float32 numpy.ndarray - qc_direct_diffuse_ratio_filter5(time)int32dask.array<chunksize=(4320,), meta=np.ndarray>
- long_name :
- Quality check results on field: Ratio of direct_normal_filter5 to diffuse_hemisp_filter5
- units :
- 1
- description :
- See global attributes for individual bit descriptions.
Array Chunk Bytes 16.88 kiB 16.88 kiB Shape (4320,) (4320,) Count 2 Graph Layers 1 Chunks Type int32 numpy.ndarray - direct_diffuse_ratio_filter6(time)float32dask.array<chunksize=(4320,), meta=np.ndarray>
- long_name :
- Ratio of direct_normal_filter6 to diffuse_hemisp_filter6
- units :
- 1
- valid_min :
- 0.0
- valid_max :
- 25.0
- centroid_wavelength :
- 939.4 nm
- FWHM :
- 6.7 nm
- ancillary_variables :
- qc_direct_diffuse_ratio_filter6
Array Chunk Bytes 16.88 kiB 16.88 kiB Shape (4320,) (4320,) Count 2 Graph Layers 1 Chunks Type float32 numpy.ndarray - qc_direct_diffuse_ratio_filter6(time)int32dask.array<chunksize=(4320,), meta=np.ndarray>
- long_name :
- Quality check results on field: Ratio of direct_normal_filter6 to diffuse_hemisp_filter6
- units :
- 1
- description :
- See global attributes for individual bit descriptions.
Array Chunk Bytes 16.88 kiB 16.88 kiB Shape (4320,) (4320,) Count 2 Graph Layers 1 Chunks Type int32 numpy.ndarray - direct_diffuse_ratio_filter7(time)float32dask.array<chunksize=(4320,), meta=np.ndarray>
- long_name :
- Ratio of direct_normal_filter6 to diffuse_hemisp_filter7
- units :
- 1
- valid_min :
- 0.0
- valid_max :
- 25.0
- centroid_wavelength :
- 1624.2 nm
- FWHM :
- 14.8 nm
- ancillary_variables :
- qc_direct_diffuse_ratio_filter7
Array Chunk Bytes 16.88 kiB 16.88 kiB Shape (4320,) (4320,) Count 2 Graph Layers 1 Chunks Type float32 numpy.ndarray - qc_direct_diffuse_ratio_filter7(time)int32dask.array<chunksize=(4320,), meta=np.ndarray>
- long_name :
- Quality check results on field: Ratio of direct_normal_filter6 to diffuse_hemisp_filter7
- units :
- 1
- description :
- See global attributes for individual bit descriptions.
Array Chunk Bytes 16.88 kiB 16.88 kiB Shape (4320,) (4320,) Count 2 Graph Layers 1 Chunks Type int32 numpy.ndarray - head_temp(time)float32dask.array<chunksize=(4320,), meta=np.ndarray>
- long_name :
- Detector cluster temperature
- units :
- degC
- valid_min :
- 30.0
- valid_max :
- 50.0
- ancillary_variables :
- qc_head_temp
Array Chunk Bytes 16.88 kiB 16.88 kiB Shape (4320,) (4320,) Count 2 Graph Layers 1 Chunks Type float32 numpy.ndarray - qc_head_temp(time)int32dask.array<chunksize=(4320,), meta=np.ndarray>
- long_name :
- Quality check results on field: Detector cluster temperature
- units :
- 1
- description :
- See global attributes for individual bit descriptions.
Array Chunk Bytes 16.88 kiB 16.88 kiB Shape (4320,) (4320,) Count 2 Graph Layers 1 Chunks Type int32 numpy.ndarray - head_temp2(time)float32dask.array<chunksize=(4320,), meta=np.ndarray>
- long_name :
- HTC300 Heater controller temperature
- units :
- degC
- valid_min :
- 30.0
- valid_max :
- 50.0
- ancillary_variables :
- qc_head_temp2
Array Chunk Bytes 16.88 kiB 16.88 kiB Shape (4320,) (4320,) Count 2 Graph Layers 1 Chunks Type float32 numpy.ndarray - qc_head_temp2(time)int32dask.array<chunksize=(4320,), meta=np.ndarray>
- long_name :
- Quality check results on field: HTC300 Heater controller temperature
- units :
- 1
- description :
- See global attributes for individual bit descriptions.
Array Chunk Bytes 16.88 kiB 16.88 kiB Shape (4320,) (4320,) Count 2 Graph Layers 1 Chunks Type int32 numpy.ndarray - logger_temperature(time)float32dask.array<chunksize=(4320,), meta=np.ndarray>
- long_name :
- Internal logger temperature
- units :
- degC
- valid_min :
- -55.0
- valid_max :
- 85.0
- ancillary_variables :
- qc_logger_temperature
Array Chunk Bytes 16.88 kiB 16.88 kiB Shape (4320,) (4320,) Count 2 Graph Layers 1 Chunks Type float32 numpy.ndarray - qc_logger_temperature(time)int32dask.array<chunksize=(4320,), meta=np.ndarray>
- long_name :
- Quality check results on field: Internal logger temperature
- units :
- 1
- description :
- See global attributes for individual bit descriptions.
Array Chunk Bytes 16.88 kiB 16.88 kiB Shape (4320,) (4320,) Count 2 Graph Layers 1 Chunks Type int32 numpy.ndarray - logger_volt(time)float32dask.array<chunksize=(4320,), meta=np.ndarray>
- long_name :
- Data logger supply voltage
- units :
- V
- valid_min :
- 10.0
- valid_max :
- 15.0
- ancillary_variables :
- qc_logger_volt
Array Chunk Bytes 16.88 kiB 16.88 kiB Shape (4320,) (4320,) Count 2 Graph Layers 1 Chunks Type float32 numpy.ndarray - qc_logger_volt(time)int32dask.array<chunksize=(4320,), meta=np.ndarray>
- long_name :
- Quality check results on field: Data logger supply voltage
- units :
- 1
- description :
- See global attributes for individual bit descriptions.
Array Chunk Bytes 16.88 kiB 16.88 kiB Shape (4320,) (4320,) Count 2 Graph Layers 1 Chunks Type int32 numpy.ndarray - solar_zenith_angle(time)float32dask.array<chunksize=(4320,), meta=np.ndarray>
- long_name :
- Apparent solar zenith angle
- units :
- degree
- corrections :
- Solar zenith angle corrected for refraction by atmosphere
Array Chunk Bytes 16.88 kiB 16.88 kiB Shape (4320,) (4320,) Count 2 Graph Layers 1 Chunks Type float32 numpy.ndarray - cosine_solar_zenith_angle(time)float32dask.array<chunksize=(4320,), meta=np.ndarray>
- long_name :
- Cosine of apparent solar zenith angle
- units :
- 1
- corrections :
- Cosine solar zenith angle corrected for refraction by atmosphere
Array Chunk Bytes 16.88 kiB 16.88 kiB Shape (4320,) (4320,) Count 2 Graph Layers 1 Chunks Type float32 numpy.ndarray - elevation_angle(time)float32dask.array<chunksize=(4320,), meta=np.ndarray>
- long_name :
- Elevation angle
- units :
- degree
Array Chunk Bytes 16.88 kiB 16.88 kiB Shape (4320,) (4320,) Count 2 Graph Layers 1 Chunks Type float32 numpy.ndarray - airmass(time)float32dask.array<chunksize=(4320,), meta=np.ndarray>
- long_name :
- Airmass
- units :
- 1
- corrections :
- Approximately 1/cosine_solar_zenith_angle with corrections for refraction and spherical atmosphere
Array Chunk Bytes 16.88 kiB 16.88 kiB Shape (4320,) (4320,) Count 2 Graph Layers 1 Chunks Type float32 numpy.ndarray - azimuth_angle(time)float32dask.array<chunksize=(4320,), meta=np.ndarray>
- long_name :
- Azimuth angle
- units :
- degree
Array Chunk Bytes 16.88 kiB 16.88 kiB Shape (4320,) (4320,) Count 2 Graph Layers 1 Chunks Type float32 numpy.ndarray - computed_cosine_correction_filter1(time)float32dask.array<chunksize=(4320,), meta=np.ndarray>
- long_name :
- Cosine correction applied to direct_horizontal_filter1
- units :
- 1
- centroid_wavelength :
- 413.3 nm
- FWHM :
- 10.9 nm
Array Chunk Bytes 16.88 kiB 16.88 kiB Shape (4320,) (4320,) Count 2 Graph Layers 1 Chunks Type float32 numpy.ndarray - computed_cosine_correction_filter2(time)float32dask.array<chunksize=(4320,), meta=np.ndarray>
- long_name :
- Cosine correction applied to direct_horizontal_filter2
- units :
- 1
- centroid_wavelength :
- 501.0 nm
- FWHM :
- 10.8 nm
Array Chunk Bytes 16.88 kiB 16.88 kiB Shape (4320,) (4320,) Count 2 Graph Layers 1 Chunks Type float32 numpy.ndarray - computed_cosine_correction_filter3(time)float32dask.array<chunksize=(4320,), meta=np.ndarray>
- long_name :
- Cosine correction applied to direct_horizontal_filter3
- units :
- 1
- centroid_wavelength :
- 613.5 nm
- FWHM :
- 10.8 nm
Array Chunk Bytes 16.88 kiB 16.88 kiB Shape (4320,) (4320,) Count 2 Graph Layers 1 Chunks Type float32 numpy.ndarray - computed_cosine_correction_filter4(time)float32dask.array<chunksize=(4320,), meta=np.ndarray>
- long_name :
- Cosine correction applied to direct_horizontal_filter4
- units :
- 1
- centroid_wavelength :
- 671.4 nm
- FWHM :
- 10.5 nm
Array Chunk Bytes 16.88 kiB 16.88 kiB Shape (4320,) (4320,) Count 2 Graph Layers 1 Chunks Type float32 numpy.ndarray - computed_cosine_correction_filter5(time)float32dask.array<chunksize=(4320,), meta=np.ndarray>
- long_name :
- Cosine correction applied to direct_horizontal_filter5
- units :
- 1
- centroid_wavelength :
- 869.3 nm
- FWHM :
- 10.0 nm
Array Chunk Bytes 16.88 kiB 16.88 kiB Shape (4320,) (4320,) Count 2 Graph Layers 1 Chunks Type float32 numpy.ndarray - computed_cosine_correction_filter6(time)float32dask.array<chunksize=(4320,), meta=np.ndarray>
- long_name :
- Cosine correction applied to direct_horizontal_filter6
- units :
- 1
- centroid_wavelength :
- 939.4 nm
- FWHM :
- 6.7 nm
Array Chunk Bytes 16.88 kiB 16.88 kiB Shape (4320,) (4320,) Count 2 Graph Layers 1 Chunks Type float32 numpy.ndarray - computed_cosine_correction_filter7(time)float32dask.array<chunksize=(4320,), meta=np.ndarray>
- long_name :
- Cosine correction applied to direct_horizontal_filter7
- units :
- 1
- centroid_wavelength :
- 1624.2 nm
- FWHM :
- 14.8 nm
Array Chunk Bytes 16.88 kiB 16.88 kiB Shape (4320,) (4320,) Count 2 Graph Layers 1 Chunks Type float32 numpy.ndarray - cosine_correction_sn_filter1(bench_angle)float32dask.array<chunksize=(181,), meta=np.ndarray>
- long_name :
- Cosine correction, south to north, filter1
- units :
- 1
- comment :
- Read from cosine_correction_source
- centroid_wavelength :
- 413.3 nm
- FWHM :
- 10.9 nm
Array Chunk Bytes 724 B 724 B Shape (181,) (181,) Count 2 Graph Layers 1 Chunks Type float32 numpy.ndarray - cosine_correction_sn_filter2(bench_angle)float32dask.array<chunksize=(181,), meta=np.ndarray>
- long_name :
- Cosine correction, south to north, filter2
- units :
- 1
- comment :
- Read from cosine_correction_source
- centroid_wavelength :
- 501.0 nm
- FWHM :
- 10.8 nm
Array Chunk Bytes 724 B 724 B Shape (181,) (181,) Count 2 Graph Layers 1 Chunks Type float32 numpy.ndarray - cosine_correction_sn_filter3(bench_angle)float32dask.array<chunksize=(181,), meta=np.ndarray>
- long_name :
- Cosine correction, south to north, filter3
- units :
- 1
- comment :
- Read from cosine_correction_source
- centroid_wavelength :
- 613.5 nm
- FWHM :
- 10.8 nm
Array Chunk Bytes 724 B 724 B Shape (181,) (181,) Count 2 Graph Layers 1 Chunks Type float32 numpy.ndarray - cosine_correction_sn_filter4(bench_angle)float32dask.array<chunksize=(181,), meta=np.ndarray>
- long_name :
- Cosine correction, south to north, filter4
- units :
- 1
- comment :
- Read from cosine_correction_source
- centroid_wavelength :
- 671.4 nm
- FWHM :
- 10.5 nm
Array Chunk Bytes 724 B 724 B Shape (181,) (181,) Count 2 Graph Layers 1 Chunks Type float32 numpy.ndarray - cosine_correction_sn_filter5(bench_angle)float32dask.array<chunksize=(181,), meta=np.ndarray>
- long_name :
- Cosine correction, south to north, filter5
- units :
- 1
- comment :
- Read from cosine_correction_source
- centroid_wavelength :
- 869.3 nm
- FWHM :
- 10.0 nm
Array Chunk Bytes 724 B 724 B Shape (181,) (181,) Count 2 Graph Layers 1 Chunks Type float32 numpy.ndarray - cosine_correction_sn_filter6(bench_angle)float32dask.array<chunksize=(181,), meta=np.ndarray>
- long_name :
- Cosine correction, south to north, filter6
- units :
- 1
- comment :
- Read from cosine_correction_source
- centroid_wavelength :
- 939.4 nm
- FWHM :
- 6.7 nm
Array Chunk Bytes 724 B 724 B Shape (181,) (181,) Count 2 Graph Layers 1 Chunks Type float32 numpy.ndarray - cosine_correction_sn_filter7(bench_angle)float32dask.array<chunksize=(181,), meta=np.ndarray>
- long_name :
- Cosine correction, south to north, filter7
- units :
- 1
- comment :
- Read from cosine_correction_source
- centroid_wavelength :
- 1624.2 nm
- FWHM :
- 14.8 nm
Array Chunk Bytes 724 B 724 B Shape (181,) (181,) Count 2 Graph Layers 1 Chunks Type float32 numpy.ndarray - cosine_correction_we_filter1(bench_angle)float32dask.array<chunksize=(181,), meta=np.ndarray>
- long_name :
- Cosine correction, west to east, filter1
- units :
- 1
- comment :
- Read from cosine_correction_source
- centroid_wavelength :
- 413.3 nm
- FWHM :
- 10.9 nm
Array Chunk Bytes 724 B 724 B Shape (181,) (181,) Count 2 Graph Layers 1 Chunks Type float32 numpy.ndarray - cosine_correction_we_filter2(bench_angle)float32dask.array<chunksize=(181,), meta=np.ndarray>
- long_name :
- Cosine correction, west to east, filter2
- units :
- 1
- comment :
- Read from cosine_correction_source
- centroid_wavelength :
- 501.0 nm
- FWHM :
- 10.8 nm
Array Chunk Bytes 724 B 724 B Shape (181,) (181,) Count 2 Graph Layers 1 Chunks Type float32 numpy.ndarray - cosine_correction_we_filter3(bench_angle)float32dask.array<chunksize=(181,), meta=np.ndarray>
- long_name :
- Cosine correction, west to east, filter3
- units :
- 1
- comment :
- Read from cosine_correction_source
- centroid_wavelength :
- 613.5 nm
- FWHM :
- 10.8 nm
Array Chunk Bytes 724 B 724 B Shape (181,) (181,) Count 2 Graph Layers 1 Chunks Type float32 numpy.ndarray - cosine_correction_we_filter4(bench_angle)float32dask.array<chunksize=(181,), meta=np.ndarray>
- long_name :
- Cosine correction, west to east, filter4
- units :
- 1
- comment :
- Read from cosine_correction_source
- centroid_wavelength :
- 671.4 nm
- FWHM :
- 10.5 nm
Array Chunk Bytes 724 B 724 B Shape (181,) (181,) Count 2 Graph Layers 1 Chunks Type float32 numpy.ndarray - cosine_correction_we_filter5(bench_angle)float32dask.array<chunksize=(181,), meta=np.ndarray>
- long_name :
- Cosine correction, west to east, filter5
- units :
- 1
- comment :
- Read from cosine_correction_source
- centroid_wavelength :
- 869.3 nm
- FWHM :
- 10.0 nm
Array Chunk Bytes 724 B 724 B Shape (181,) (181,) Count 2 Graph Layers 1 Chunks Type float32 numpy.ndarray - cosine_correction_we_filter6(bench_angle)float32dask.array<chunksize=(181,), meta=np.ndarray>
- long_name :
- Cosine correction, west to east, filter6
- units :
- 1
- comment :
- Read from cosine_correction_source
- centroid_wavelength :
- 939.4 nm
- FWHM :
- 6.7 nm
Array Chunk Bytes 724 B 724 B Shape (181,) (181,) Count 2 Graph Layers 1 Chunks Type float32 numpy.ndarray - cosine_correction_we_filter7(bench_angle)float32dask.array<chunksize=(181,), meta=np.ndarray>
- long_name :
- Cosine correction, west to east, filter7
- units :
- 1
- comment :
- Read from cosine_correction_source
- centroid_wavelength :
- 1624.2 nm
- FWHM :
- 14.8 nm
Array Chunk Bytes 724 B 724 B Shape (181,) (181,) Count 2 Graph Layers 1 Chunks Type float32 numpy.ndarray - wavelength_filter1(wavelength)float32dask.array<chunksize=(750,), meta=np.ndarray>
- long_name :
- Normalized filter function data, filter 1, wavelength value obtained during instrument characterization
- units :
- nm
- centroid_wavelength :
- 413.3 nm
- FWHM :
- 10.9 nm
Array Chunk Bytes 2.93 kiB 2.93 kiB Shape (750,) (750,) Count 2 Graph Layers 1 Chunks Type float32 numpy.ndarray - normalized_transmittance_filter1(wavelength)float32dask.array<chunksize=(750,), meta=np.ndarray>
- long_name :
- Normalized filter function data, filter 1. Measured transmittance value normalized to area under curve.
- units :
- 1
- centroid_wavelength :
- 413.3 nm
- FWHM :
- 10.9 nm
Array Chunk Bytes 2.93 kiB 2.93 kiB Shape (750,) (750,) Count 2 Graph Layers 1 Chunks Type float32 numpy.ndarray - wavelength_filter2(wavelength)float32dask.array<chunksize=(750,), meta=np.ndarray>
- long_name :
- Normalized filter function data, filter 2, wavelength value obtained during instrument characterization
- units :
- nm
- centroid_wavelength :
- 501.0 nm
- FWHM :
- 10.8 nm
Array Chunk Bytes 2.93 kiB 2.93 kiB Shape (750,) (750,) Count 2 Graph Layers 1 Chunks Type float32 numpy.ndarray - normalized_transmittance_filter2(wavelength)float32dask.array<chunksize=(750,), meta=np.ndarray>
- long_name :
- Normalized filter function data, filter 2. Measured transmittance value normalized to area under curve.
- units :
- 1
- centroid_wavelength :
- 501.0 nm
- FWHM :
- 10.8 nm
Array Chunk Bytes 2.93 kiB 2.93 kiB Shape (750,) (750,) Count 2 Graph Layers 1 Chunks Type float32 numpy.ndarray - wavelength_filter3(wavelength)float32dask.array<chunksize=(750,), meta=np.ndarray>
- long_name :
- Normalized filter function data, filter 3, wavelength value obtained during instrument characterization
- units :
- nm
- centroid_wavelength :
- 613.5 nm
- FWHM :
- 10.8 nm
Array Chunk Bytes 2.93 kiB 2.93 kiB Shape (750,) (750,) Count 2 Graph Layers 1 Chunks Type float32 numpy.ndarray - normalized_transmittance_filter3(wavelength)float32dask.array<chunksize=(750,), meta=np.ndarray>
- long_name :
- Normalized filter function data, filter 3. Measured transmittance value normalized to area under curve.
- units :
- 1
- centroid_wavelength :
- 613.5 nm
- FWHM :
- 10.8 nm
Array Chunk Bytes 2.93 kiB 2.93 kiB Shape (750,) (750,) Count 2 Graph Layers 1 Chunks Type float32 numpy.ndarray - wavelength_filter4(wavelength)float32dask.array<chunksize=(750,), meta=np.ndarray>
- long_name :
- Normalized filter function data, filter 4, wavelength value obtained during instrument characterization
- units :
- nm
- centroid_wavelength :
- 671.4 nm
- FWHM :
- 10.5 nm
Array Chunk Bytes 2.93 kiB 2.93 kiB Shape (750,) (750,) Count 2 Graph Layers 1 Chunks Type float32 numpy.ndarray - normalized_transmittance_filter4(wavelength)float32dask.array<chunksize=(750,), meta=np.ndarray>
- long_name :
- Normalized filter function data, filter 4. Measured transmittance value normalized to area under curve.
- units :
- 1
- centroid_wavelength :
- 671.4 nm
- FWHM :
- 10.5 nm
Array Chunk Bytes 2.93 kiB 2.93 kiB Shape (750,) (750,) Count 2 Graph Layers 1 Chunks Type float32 numpy.ndarray - wavelength_filter5(wavelength)float32dask.array<chunksize=(750,), meta=np.ndarray>
- long_name :
- Normalized filter function data, filter 5, wavelength value obtained during instrument characterization
- units :
- nm
- centroid_wavelength :
- 869.3 nm
- FWHM :
- 10.0 nm
Array Chunk Bytes 2.93 kiB 2.93 kiB Shape (750,) (750,) Count 2 Graph Layers 1 Chunks Type float32 numpy.ndarray - normalized_transmittance_filter5(wavelength)float32dask.array<chunksize=(750,), meta=np.ndarray>
- long_name :
- Normalized filter function data, filter 5. Measured transmittance value normalized to area under curve.
- units :
- 1
- centroid_wavelength :
- 869.3 nm
- FWHM :
- 10.0 nm
Array Chunk Bytes 2.93 kiB 2.93 kiB Shape (750,) (750,) Count 2 Graph Layers 1 Chunks Type float32 numpy.ndarray - wavelength_filter6(wavelength)float32dask.array<chunksize=(750,), meta=np.ndarray>
- long_name :
- Normalized filter function data, filter 6, wavelength value obtained during instrument characterization
- units :
- nm
- centroid_wavelength :
- 939.4 nm
- FWHM :
- 6.7 nm
Array Chunk Bytes 2.93 kiB 2.93 kiB Shape (750,) (750,) Count 2 Graph Layers 1 Chunks Type float32 numpy.ndarray - normalized_transmittance_filter6(wavelength)float32dask.array<chunksize=(750,), meta=np.ndarray>
- long_name :
- Normalized filter function data, filter 6. Measured transmittance value normalized to area under curve.
- units :
- 1
- centroid_wavelength :
- 939.4 nm
- FWHM :
- 6.7 nm
Array Chunk Bytes 2.93 kiB 2.93 kiB Shape (750,) (750,) Count 2 Graph Layers 1 Chunks Type float32 numpy.ndarray - wavelength_filter7(wavelength)float32dask.array<chunksize=(750,), meta=np.ndarray>
- long_name :
- Normalized filter function data, filter 7, wavelength value obtained during instrument characterization
- units :
- nm
- centroid_wavelength :
- 1624.2 nm
- FWHM :
- 14.8 nm
Array Chunk Bytes 2.93 kiB 2.93 kiB Shape (750,) (750,) Count 2 Graph Layers 1 Chunks Type float32 numpy.ndarray - normalized_transmittance_filter7(wavelength)float32dask.array<chunksize=(750,), meta=np.ndarray>
- long_name :
- Normalized filter function data, filter 7. Measured transmittance value normalized to area under curve.
- units :
- 1
- centroid_wavelength :
- 1624.2 nm
- FWHM :
- 14.8 nm
Array Chunk Bytes 2.93 kiB 2.93 kiB Shape (750,) (750,) Count 2 Graph Layers 1 Chunks Type float32 numpy.ndarray - offset_filter1(time)float32dask.array<chunksize=(4320,), meta=np.ndarray>
- long_name :
- Offset value subtracted from hemispheric and diffuse filter1 data
- units :
- mV
Array Chunk Bytes 16.88 kiB 16.88 kiB Shape (4320,) (4320,) Count 2 Graph Layers 1 Chunks Type float32 numpy.ndarray - offset_filter2(time)float32dask.array<chunksize=(4320,), meta=np.ndarray>
- long_name :
- Offset value subtracted from hemispheric and diffuse filter2 data
- units :
- mV
Array Chunk Bytes 16.88 kiB 16.88 kiB Shape (4320,) (4320,) Count 2 Graph Layers 1 Chunks Type float32 numpy.ndarray - offset_filter3(time)float32dask.array<chunksize=(4320,), meta=np.ndarray>
- long_name :
- Offset value subtracted from hemispheric and diffuse filter3 data
- units :
- mV
Array Chunk Bytes 16.88 kiB 16.88 kiB Shape (4320,) (4320,) Count 2 Graph Layers 1 Chunks Type float32 numpy.ndarray - offset_filter4(time)float32dask.array<chunksize=(4320,), meta=np.ndarray>
- long_name :
- Offset value subtracted from hemispheric and diffuse filter4 data
- units :
- mV
Array Chunk Bytes 16.88 kiB 16.88 kiB Shape (4320,) (4320,) Count 2 Graph Layers 1 Chunks Type float32 numpy.ndarray - offset_filter5(time)float32dask.array<chunksize=(4320,), meta=np.ndarray>
- long_name :
- Offset value subtracted from hemispheric and diffuse filter5 data
- units :
- mV
Array Chunk Bytes 16.88 kiB 16.88 kiB Shape (4320,) (4320,) Count 2 Graph Layers 1 Chunks Type float32 numpy.ndarray - offset_filter6(time)float32dask.array<chunksize=(4320,), meta=np.ndarray>
- long_name :
- Offset value subtracted from hemispheric and diffuse filter6 data
- units :
- mV
Array Chunk Bytes 16.88 kiB 16.88 kiB Shape (4320,) (4320,) Count 2 Graph Layers 1 Chunks Type float32 numpy.ndarray - offset_filter7(time)float32dask.array<chunksize=(4320,), meta=np.ndarray>
- long_name :
- Offset value subtracted from hemispheric and diffuse filter7 data
- units :
- mV
Array Chunk Bytes 16.88 kiB 16.88 kiB Shape (4320,) (4320,) Count 2 Graph Layers 1 Chunks Type float32 numpy.ndarray - diffuse_correction_filter1()float32...
- long_name :
- Cosine correction of filter1 diffuse, assuming Rayleigh sky at 45 deg zenith angle
- units :
- 1
array(0.993)
- diffuse_correction_filter2()float32...
- long_name :
- Cosine correction of filter2 diffuse, assuming Rayleigh sky at 45 deg zenith angle
- units :
- 1
array(0.999)
- diffuse_correction_filter3()float32...
- long_name :
- Cosine correction of filter3 diffuse, assuming Rayleigh sky at 45 deg zenith angle
- units :
- 1
array(1.)
- diffuse_correction_filter4()float32...
- long_name :
- Cosine correction of filter4 diffuse, assuming Rayleigh sky at 45 deg zenith angle
- units :
- 1
array(1.004)
- diffuse_correction_filter5()float32...
- long_name :
- Cosine correction of filter5 diffuse, assuming Rayleigh sky at 45 deg zenith angle
- units :
- 1
array(1.007)
- diffuse_correction_filter6()float32...
- long_name :
- Cosine correction of filter6 diffuse, assuming Rayleigh sky at 45 deg zenith angle
- units :
- 1
array(1.007)
- diffuse_correction_filter7()float32...
- long_name :
- Cosine correction of filter7 diffuse, assuming Rayleigh sky at 45 deg zenith angle
- units :
- 1
array(0.996)
- nominal_calibration_factor_filter1()float32...
- long_name :
- Nominal calibration factor using standard lamp, applied to filter1 data
- units :
- mV/(W/(m^2 nm))
array(91.797997)
- nominal_calibration_factor_filter2()float32...
- long_name :
- Nominal calibration factor using standard lamp, applied to filter2 data
- units :
- mV/(W/(m^2 nm))
array(85.822197)
- nominal_calibration_factor_filter3()float32...
- long_name :
- Nominal calibration factor using standard lamp, applied to filter3 data
- units :
- mV/(W/(m^2 nm))
array(98.297401)
- nominal_calibration_factor_filter4()float32...
- long_name :
- Nominal calibration factor using standard lamp, applied to filter4 data
- units :
- mV/(W/(m^2 nm))
array(86.158302)
- nominal_calibration_factor_filter5()float32...
- long_name :
- Nominal calibration factor using standard lamp, applied to filter5 data
- units :
- mV/(W/(m^2 nm))
array(164.6082)
- nominal_calibration_factor_filter6()float32...
- long_name :
- Nominal calibration factor using standard lamp, applied to filter6 data
- units :
- mV/(W/(m^2 nm))
array(340.009796)
- nominal_calibration_factor_filter7()float32...
- long_name :
- Nominal calibration factor using standard lamp, applied to filter7 data
- units :
- mV/(W/(m^2 nm))
array(30.)
- lat()float32...
- long_name :
- North latitude
- units :
- degree_N
- valid_min :
- -90.0
- valid_max :
- 90.0
- standard_name :
- latitude
array(36.881, dtype=float32)
- lon()float32...
- long_name :
- East longitude
- units :
- degree_E
- valid_min :
- -180.0
- valid_max :
- 180.0
- standard_name :
- longitude
array(-98.285, dtype=float32)
- alt()float32...
- long_name :
- Altitude above mean sea level
- units :
- m
- standard_name :
- altitude
array(360., dtype=float32)
- command_line :
- mfrsr7nch_ingest -s sgp -f E11
- Conventions :
- ARM-1.2
- process_version :
- ingest-mfrsr7nch-1.3-0.el7
- dod_version :
- mfrsr7nch-b1-1.1
- input_source :
- /data/collection/sgp/sgpmfrsr7nchE11.00/MFRSR_Table155.20210329070000.dat
- site_id :
- sgp
- platform_id :
- mfrsr7nch
- facility_id :
- E11
- data_level :
- b1
- location_description :
- Southern Great Plains (SGP), Byron, Oklahoma
- datastream :
- sgpmfrsr7nchE11.b1
- serial_number :
- Refer to logger_id and head_id
- sampling_interval :
- 20 seconds
- logger_software_version :
- 1.2
- logger_id :
- 1760
- head_id :
- 180056578
- mfr_internal_latitude :
- 36.881000
- mfr_internal_longitude :
- -98.285000
- filter_information :
- Filters 1-5 and 7 for aerosol, and filter 6 for water vapor.
- shadowband_timing :
- Due to shadowband motion, there is an inherent lag between the time stamp in the file and the time corresponding to the direct beam measurement which varies throughout the day. On average this lag is about five seconds, therefore five seconds are added to the timestamp when calculating solar position.
- cosine_correction_source :
- CosineCorr.sgpmfrsr7nchE11.20201209.dat
- diffuse_correction_source :
- DiffuseCorr.sgpmfrsr7nchE11.20201209.dat
- filter_trace_source :
- FilterInfo.sgpmfrsr7nchE11.20201209.dat
- nominal_calibration_source :
- NominalCal.sgpmfrsr7nchE11.20201209.dat
- offset_correction_source :
- Offsets are computed using a two hour average of the alltime_hemisp_narrowband data centered around the time of the maximum solar zenith angle. For higher and lower latitudes the averaging window can shrink to only include values where the solar zenith angle is >= 97 degrees. If less than 30 minutes of nighttime data is available the previously computed values will be used.
- qc_bit_comment :
- The QC field values are a bit packed representation of true/false values for the tests that may have been performed. A QC value of zero means that none of the tests performed on the value failed.
- qc_bit_1_description :
- Value is equal to missing_value.
- qc_bit_1_assessment :
- Bad
- qc_bit_2_description :
- Value is less than the valid_min.
- qc_bit_2_assessment :
- Bad
- qc_bit_3_description :
- Value is greater than the valid_max.
- qc_bit_3_assessment :
- Bad
- doi :
- 10.5439/1429369
- history :
- created by user dsmgr on machine flint at 2021-03-29 09:43:00, using ingest-mfrsr7nch-1.3-0.el7
- _file_dates :
- ['20210329']
- _file_times :
- ['070000']
- _datastream :
- sgpmfrsr7nchE11.b1
- _arm_standards_flag :
- 1
Clean up the object to CF Standards
In order to utilize all the ACT QC modules, we need to clean up the object to follow Climate and Forecast (CF) standards.
ds.clean.cleanup()
ds
<xarray.Dataset> Dimensions: (time: 4320, bench_angle: 181, wavelength: 750) Coordinates: * time (time) datetime64[ns] 2021-03-29... * bench_angle (bench_angle) float32 0.0 ... 180.0 Dimensions without coordinates: wavelength Data variables: (12/158) base_time datetime64[ns] 2021-03-29 time_offset (time) datetime64[ns] 2021-03-29... hemisp_narrowband_filter1 (time) float32 dask.array<chunksize=(4320,), meta=np.ndarray> qc_hemisp_narrowband_filter1 (time) int32 dask.array<chunksize=(4320,), meta=np.ndarray> hemisp_narrowband_filter2 (time) float32 dask.array<chunksize=(4320,), meta=np.ndarray> qc_hemisp_narrowband_filter2 (time) int32 dask.array<chunksize=(4320,), meta=np.ndarray> ... ... nominal_calibration_factor_filter5 float32 ... nominal_calibration_factor_filter6 float32 ... nominal_calibration_factor_filter7 float32 ... lat float32 ... lon float32 ... alt float32 ... Attributes: (12/31) command_line: mfrsr7nch_ingest -s sgp -f E11 Conventions: ARM-1.2 process_version: ingest-mfrsr7nch-1.3-0.el7 dod_version: mfrsr7nch-b1-1.1 input_source: /data/collection/sgp/sgpmfrsr7nchE11.00/MFRS... site_id: sgp ... ... doi: 10.5439/1429369 history: created by user dsmgr on machine flint at 20... _file_dates: ['20210329'] _file_times: ['070000'] _datastream: sgpmfrsr7nchE11.b1 _arm_standards_flag: 1
- time: 4320
- bench_angle: 181
- wavelength: 750
- time(time)datetime64[ns]2021-03-29T07:00:00 ... 2021-03-...
- long_name :
- Time offset from midnight
- standard_name :
- time
array(['2021-03-29T07:00:00.000000000', '2021-03-29T07:00:20.000000000', '2021-03-29T07:00:40.000000000', ..., '2021-03-30T06:59:00.000000000', '2021-03-30T06:59:20.000000000', '2021-03-30T06:59:40.000000000'], dtype='datetime64[ns]')
- bench_angle(bench_angle)float320.0 1.0 2.0 ... 178.0 179.0 180.0
- long_name :
- Angle of incidence during cosine bench measurements
- units :
- degree
- head_orientation :
- Head is oriented so shadowband is south
- South_to_North :
- 0 is S, 90 is zenith, 180 is N
- West_to_East :
- 0 is W, 90 is zenith, 180 is E
array([ 0., 1., 2., 3., 4., 5., 6., 7., 8., 9., 10., 11., 12., 13., 14., 15., 16., 17., 18., 19., 20., 21., 22., 23., 24., 25., 26., 27., 28., 29., 30., 31., 32., 33., 34., 35., 36., 37., 38., 39., 40., 41., 42., 43., 44., 45., 46., 47., 48., 49., 50., 51., 52., 53., 54., 55., 56., 57., 58., 59., 60., 61., 62., 63., 64., 65., 66., 67., 68., 69., 70., 71., 72., 73., 74., 75., 76., 77., 78., 79., 80., 81., 82., 83., 84., 85., 86., 87., 88., 89., 90., 91., 92., 93., 94., 95., 96., 97., 98., 99., 100., 101., 102., 103., 104., 105., 106., 107., 108., 109., 110., 111., 112., 113., 114., 115., 116., 117., 118., 119., 120., 121., 122., 123., 124., 125., 126., 127., 128., 129., 130., 131., 132., 133., 134., 135., 136., 137., 138., 139., 140., 141., 142., 143., 144., 145., 146., 147., 148., 149., 150., 151., 152., 153., 154., 155., 156., 157., 158., 159., 160., 161., 162., 163., 164., 165., 166., 167., 168., 169., 170., 171., 172., 173., 174., 175., 176., 177., 178., 179., 180.], dtype=float32)
- base_time()datetime64[ns]2021-03-29
- string :
- 2021-03-29 00:00:00 0:00
- long_name :
- Base time in Epoch
- ancillary_variables :
- time_offset
array('2021-03-29T00:00:00.000000000', dtype='datetime64[ns]')
- time_offset(time)datetime64[ns]2021-03-29T07:00:00 ... 2021-03-...
- long_name :
- Time offset from base_time
- ancillary_variables :
- base_time
array(['2021-03-29T07:00:00.000000000', '2021-03-29T07:00:20.000000000', '2021-03-29T07:00:40.000000000', ..., '2021-03-30T06:59:00.000000000', '2021-03-30T06:59:20.000000000', '2021-03-30T06:59:40.000000000'], dtype='datetime64[ns]')
- hemisp_narrowband_filter1(time)float32dask.array<chunksize=(4320,), meta=np.ndarray>
- long_name :
- Narrowband hemispheric irradiance, filter 1, offset and cosine corrected
- units :
- W/(m^2 nm)
- explanation_of_narrowband_channel :
- The nominal center wavelength is 415 nm, nominal half-power width is 10 nm
- corrections :
- offset subtracted, cosine corrected
- comment :
- sum of diffuse_hemisp_filter1 and direct_horizontal_filter1
- centroid_wavelength :
- 413.3 nm
- FWHM :
- 10.9 nm
- ancillary_variables :
- qc_hemisp_narrowband_filter1
Array Chunk Bytes 16.88 kiB 16.88 kiB Shape (4320,) (4320,) Count 2 Graph Layers 1 Chunks Type float32 numpy.ndarray - qc_hemisp_narrowband_filter1(time)int32dask.array<chunksize=(4320,), meta=np.ndarray>
- long_name :
- Quality check results on field: Narrowband hemispheric irradiance, filter 1, offset and cosine corrected
- units :
- 1
- flag_masks :
- [1, 2, 4]
- flag_meanings :
- ['Value is equal to missing_value.', 'Value is less than the fail_min.', 'Value is greater than the fail_max.']
- flag_assessments :
- ['Bad', 'Bad', 'Bad']
- fail_min :
- 0.0
- fail_max :
- 1.875
- standard_name :
- quality_flag
Array Chunk Bytes 16.88 kiB 16.88 kiB Shape (4320,) (4320,) Count 2 Graph Layers 1 Chunks Type int32 numpy.ndarray - hemisp_narrowband_filter2(time)float32dask.array<chunksize=(4320,), meta=np.ndarray>
- long_name :
- Narrowband hemispheric irradiance, filter 2, offset and cosine corrected
- units :
- W/(m^2 nm)
- explanation_of_narrowband_channel :
- The nominal center wavelength is 500 nm, nominal half-power width is 10 nm
- corrections :
- offset subtracted, cosine corrected
- comment :
- sum of diffuse_hemisp_filter2 and direct_horizontal_filter2
- centroid_wavelength :
- 501.0 nm
- FWHM :
- 10.8 nm
- ancillary_variables :
- qc_hemisp_narrowband_filter2
Array Chunk Bytes 16.88 kiB 16.88 kiB Shape (4320,) (4320,) Count 2 Graph Layers 1 Chunks Type float32 numpy.ndarray - qc_hemisp_narrowband_filter2(time)int32dask.array<chunksize=(4320,), meta=np.ndarray>
- long_name :
- Quality check results on field: Narrowband hemispheric irradiance, filter 2, offset and cosine corrected
- units :
- 1
- flag_masks :
- [1, 2, 4]
- flag_meanings :
- ['Value is equal to missing_value.', 'Value is less than the fail_min.', 'Value is greater than the fail_max.']
- flag_assessments :
- ['Bad', 'Bad', 'Bad']
- fail_min :
- 0.0
- fail_max :
- 2.0
- standard_name :
- quality_flag
Array Chunk Bytes 16.88 kiB 16.88 kiB Shape (4320,) (4320,) Count 2 Graph Layers 1 Chunks Type int32 numpy.ndarray - hemisp_narrowband_filter3(time)float32dask.array<chunksize=(4320,), meta=np.ndarray>
- long_name :
- Narrowband hemispheric irradiance, filter 3, offset and cosine corrected
- units :
- W/(m^2 nm)
- explanation_of_narrowband_channel :
- The nominal center wavelength is 615 nm, nominal half-power width is 10 nm
- corrections :
- offset subtracted, cosine corrected
- comment :
- sum of diffuse_hemisp_filter3 and direct_horizontal_filter3
- centroid_wavelength :
- 613.5 nm
- FWHM :
- 10.8 nm
- ancillary_variables :
- qc_hemisp_narrowband_filter3
Array Chunk Bytes 16.88 kiB 16.88 kiB Shape (4320,) (4320,) Count 2 Graph Layers 1 Chunks Type float32 numpy.ndarray - qc_hemisp_narrowband_filter3(time)int32dask.array<chunksize=(4320,), meta=np.ndarray>
- long_name :
- Quality check results on field: Narrowband hemispheric irradiance, filter 3, offset and cosine corrected
- units :
- 1
- flag_masks :
- [1, 2, 4]
- flag_meanings :
- ['Value is equal to missing_value.', 'Value is less than the fail_min.', 'Value is greater than the fail_max.']
- flag_assessments :
- ['Bad', 'Bad', 'Bad']
- fail_min :
- 0.0
- fail_max :
- 1.875
- standard_name :
- quality_flag
Array Chunk Bytes 16.88 kiB 16.88 kiB Shape (4320,) (4320,) Count 2 Graph Layers 1 Chunks Type int32 numpy.ndarray - hemisp_narrowband_filter4(time)float32dask.array<chunksize=(4320,), meta=np.ndarray>
- long_name :
- Narrowband hemispheric irradiance, filter 4, offset and cosine corrected
- units :
- W/(m^2 nm)
- explanation_of_narrowband_channel :
- The nominal center wavelength is 673 nm, nominal half-power width is 10 nm
- corrections :
- offset subtracted, cosine corrected
- comment :
- sum of diffuse_hemisp_filter4 and direct_horizontal_filter4
- centroid_wavelength :
- 671.4 nm
- FWHM :
- 10.5 nm
- ancillary_variables :
- qc_hemisp_narrowband_filter4
Array Chunk Bytes 16.88 kiB 16.88 kiB Shape (4320,) (4320,) Count 2 Graph Layers 1 Chunks Type float32 numpy.ndarray - qc_hemisp_narrowband_filter4(time)int32dask.array<chunksize=(4320,), meta=np.ndarray>
- long_name :
- Quality check results on field: Narrowband hemispheric irradiance, filter 4, offset and cosine corrected
- units :
- 1
- flag_masks :
- [1, 2, 4]
- flag_meanings :
- ['Value is equal to missing_value.', 'Value is less than the fail_min.', 'Value is greater than the fail_max.']
- flag_assessments :
- ['Bad', 'Bad', 'Bad']
- fail_min :
- 0.0
- fail_max :
- 1.75
- standard_name :
- quality_flag
Array Chunk Bytes 16.88 kiB 16.88 kiB Shape (4320,) (4320,) Count 2 Graph Layers 1 Chunks Type int32 numpy.ndarray - hemisp_narrowband_filter5(time)float32dask.array<chunksize=(4320,), meta=np.ndarray>
- long_name :
- Narrowband hemispheric irradiance, filter 5, offset and cosine corrected
- units :
- W/(m^2 nm)
- explanation_of_narrowband_channel :
- The nominal center wavelength is 870 nm, nominal half-power width is 10 nm
- corrections :
- offset subtracted, cosine corrected
- comment :
- sum of diffuse_hemisp_filter5 and direct_horizontal_filter5
- centroid_wavelength :
- 869.3 nm
- FWHM :
- 10.0 nm
- ancillary_variables :
- qc_hemisp_narrowband_filter5
Array Chunk Bytes 16.88 kiB 16.88 kiB Shape (4320,) (4320,) Count 2 Graph Layers 1 Chunks Type float32 numpy.ndarray - qc_hemisp_narrowband_filter5(time)int32dask.array<chunksize=(4320,), meta=np.ndarray>
- long_name :
- Quality check results on field: Narrowband hemispheric irradiance, filter 5, offset and cosine corrected
- units :
- 1
- flag_masks :
- [1, 2, 4]
- flag_meanings :
- ['Value is equal to missing_value.', 'Value is less than the fail_min.', 'Value is greater than the fail_max.']
- flag_assessments :
- ['Bad', 'Bad', 'Bad']
- fail_min :
- 0.0
- fail_max :
- 1.25
- standard_name :
- quality_flag
Array Chunk Bytes 16.88 kiB 16.88 kiB Shape (4320,) (4320,) Count 2 Graph Layers 1 Chunks Type int32 numpy.ndarray - hemisp_narrowband_filter6(time)float32dask.array<chunksize=(4320,), meta=np.ndarray>
- long_name :
- Narrowband hemispheric irradiance, filter 6, offset and cosine corrected
- units :
- W/(m^2 nm)
- explanation_of_narrowband_channel :
- The nominal center wavelength is 940 nm, nominal half-power width is 10 nm
- corrections :
- offset subtracted, cosine corrected
- comment :
- sum of diffuse_hemisp_filter6 and direct_horizontal_filter6
- centroid_wavelength :
- 939.4 nm
- FWHM :
- 6.7 nm
- ancillary_variables :
- qc_hemisp_narrowband_filter6
Array Chunk Bytes 16.88 kiB 16.88 kiB Shape (4320,) (4320,) Count 2 Graph Layers 1 Chunks Type float32 numpy.ndarray - qc_hemisp_narrowband_filter6(time)int32dask.array<chunksize=(4320,), meta=np.ndarray>
- long_name :
- Quality check results on field: Narrowband hemispheric irradiance, filter 6, offset and cosine corrected
- units :
- 1
- flag_masks :
- [1, 2, 4]
- flag_meanings :
- ['Value is equal to missing_value.', 'Value is less than the fail_min.', 'Value is greater than the fail_max.']
- flag_assessments :
- ['Bad', 'Bad', 'Bad']
- fail_min :
- 0.0
- fail_max :
- 1.5
- standard_name :
- quality_flag
Array Chunk Bytes 16.88 kiB 16.88 kiB Shape (4320,) (4320,) Count 2 Graph Layers 1 Chunks Type int32 numpy.ndarray - hemisp_narrowband_filter7(time)float32dask.array<chunksize=(4320,), meta=np.ndarray>
- long_name :
- Narrowband hemispheric irradiance, filter 7, offset and cosine corrected
- units :
- W/(m^2 nm)
- explanation_of_narrowband_channel :
- The nominal center wavelength is 1625 nm, nominal half-power width is 10 nm
- corrections :
- offset subtracted, cosine corrected
- comment :
- sum of diffuse_hemisp_filter7 and direct_horizontal_filter7
- centroid_wavelength :
- 1624.2 nm
- FWHM :
- 14.8 nm
- ancillary_variables :
- qc_hemisp_narrowband_filter7
Array Chunk Bytes 16.88 kiB 16.88 kiB Shape (4320,) (4320,) Count 2 Graph Layers 1 Chunks Type float32 numpy.ndarray - qc_hemisp_narrowband_filter7(time)int32dask.array<chunksize=(4320,), meta=np.ndarray>
- long_name :
- Quality check results on field: Narrowband hemispheric irradiance, filter 7, offset and cosine corrected
- units :
- 1
- flag_masks :
- [1, 2, 4]
- flag_meanings :
- ['Value is equal to missing_value.', 'Value is less than the fail_min.', 'Value is greater than the fail_max.']
- flag_assessments :
- ['Bad', 'Bad', 'Bad']
- fail_min :
- 0.0
- fail_max :
- 5.0
- standard_name :
- quality_flag
Array Chunk Bytes 16.88 kiB 16.88 kiB Shape (4320,) (4320,) Count 2 Graph Layers 1 Chunks Type int32 numpy.ndarray - diffuse_hemisp_narrowband_filter1(time)float32dask.array<chunksize=(4320,), meta=np.ndarray>
- long_name :
- Narrowband diffuse hemispheric irradiance, filter 1, offset and cosine corrected
- units :
- W/(m^2 nm)
- explanation_of_narrowband_channel :
- The nominal center wavelength is 415 nm, nominal half-power width is 10 nm
- corrections :
- offset subtracted, cosine corrected
- centroid_wavelength :
- 413.3 nm
- FWHM :
- 10.9 nm
- ancillary_variables :
- qc_diffuse_hemisp_narrowband_filter1
Array Chunk Bytes 16.88 kiB 16.88 kiB Shape (4320,) (4320,) Count 2 Graph Layers 1 Chunks Type float32 numpy.ndarray - qc_diffuse_hemisp_narrowband_filter1(time)int32dask.array<chunksize=(4320,), meta=np.ndarray>
- long_name :
- Quality check results on field: Narrowband diffuse hemispheric irradiance, filter 1, offset and cosine corrected
- units :
- 1
- flag_masks :
- [1, 2, 4]
- flag_meanings :
- ['Value is equal to missing_value.', 'Value is less than the fail_min.', 'Value is greater than the fail_max.']
- flag_assessments :
- ['Bad', 'Bad', 'Bad']
- fail_min :
- 0.0
- fail_max :
- 1.875
- standard_name :
- quality_flag
Array Chunk Bytes 16.88 kiB 16.88 kiB Shape (4320,) (4320,) Count 2 Graph Layers 1 Chunks Type int32 numpy.ndarray - diffuse_hemisp_narrowband_filter2(time)float32dask.array<chunksize=(4320,), meta=np.ndarray>
- long_name :
- Narrowband diffuse hemispheric irradiance, filter 2, offset and cosine corrected
- units :
- W/(m^2 nm)
- explanation_of_narrowband_channel :
- The nominal center wavelength is 500 nm, nominal half-power width is 10 nm
- corrections :
- offset subtracted, cosine corrected
- centroid_wavelength :
- 501.0 nm
- FWHM :
- 10.8 nm
- ancillary_variables :
- qc_diffuse_hemisp_narrowband_filter2
Array Chunk Bytes 16.88 kiB 16.88 kiB Shape (4320,) (4320,) Count 2 Graph Layers 1 Chunks Type float32 numpy.ndarray - qc_diffuse_hemisp_narrowband_filter2(time)int32dask.array<chunksize=(4320,), meta=np.ndarray>
- long_name :
- Quality check results on field: Narrowband diffuse hemispheric irradiance, filter 2, offset and cosine corrected
- units :
- 1
- flag_masks :
- [1, 2, 4]
- flag_meanings :
- ['Value is equal to missing_value.', 'Value is less than the fail_min.', 'Value is greater than the fail_max.']
- flag_assessments :
- ['Bad', 'Bad', 'Bad']
- fail_min :
- 0.0
- fail_max :
- 2.0
- standard_name :
- quality_flag
Array Chunk Bytes 16.88 kiB 16.88 kiB Shape (4320,) (4320,) Count 2 Graph Layers 1 Chunks Type int32 numpy.ndarray - diffuse_hemisp_narrowband_filter3(time)float32dask.array<chunksize=(4320,), meta=np.ndarray>
- long_name :
- Narrowband diffuse hemispheric irradiance, filter 3, offset and cosine corrected
- units :
- W/(m^2 nm)
- explanation_of_narrowband_channel :
- The nominal center wavelength is 615 nm, nominal half-power width is 10 nm
- corrections :
- offset subtracted, cosine corrected
- centroid_wavelength :
- 613.5 nm
- FWHM :
- 10.8 nm
- ancillary_variables :
- qc_diffuse_hemisp_narrowband_filter3
Array Chunk Bytes 16.88 kiB 16.88 kiB Shape (4320,) (4320,) Count 2 Graph Layers 1 Chunks Type float32 numpy.ndarray - qc_diffuse_hemisp_narrowband_filter3(time)int32dask.array<chunksize=(4320,), meta=np.ndarray>
- long_name :
- Quality check results on field: Narrowband diffuse hemispheric irradiance, filter 3, offset and cosine corrected
- units :
- 1
- flag_masks :
- [1, 2, 4]
- flag_meanings :
- ['Value is equal to missing_value.', 'Value is less than the fail_min.', 'Value is greater than the fail_max.']
- flag_assessments :
- ['Bad', 'Bad', 'Bad']
- fail_min :
- 0.0
- fail_max :
- 1.875
- standard_name :
- quality_flag
Array Chunk Bytes 16.88 kiB 16.88 kiB Shape (4320,) (4320,) Count 2 Graph Layers 1 Chunks Type int32 numpy.ndarray - diffuse_hemisp_narrowband_filter4(time)float32dask.array<chunksize=(4320,), meta=np.ndarray>
- long_name :
- Narrowband diffuse hemispheric irradiance, filter 4, offset and cosine corrected
- units :
- W/(m^2 nm)
- explanation_of_narrowband_channel :
- The nominal center wavelength is 673 nm, nominal half-power width is 10 nm
- corrections :
- offset subtracted, cosine corrected
- centroid_wavelength :
- 671.4 nm
- FWHM :
- 10.5 nm
- ancillary_variables :
- qc_diffuse_hemisp_narrowband_filter4
Array Chunk Bytes 16.88 kiB 16.88 kiB Shape (4320,) (4320,) Count 2 Graph Layers 1 Chunks Type float32 numpy.ndarray - qc_diffuse_hemisp_narrowband_filter4(time)int32dask.array<chunksize=(4320,), meta=np.ndarray>
- long_name :
- Quality check results on field: Narrowband diffuse hemispheric irradiance, filter 4, offset and cosine corrected
- units :
- 1
- flag_masks :
- [1, 2, 4]
- flag_meanings :
- ['Value is equal to missing_value.', 'Value is less than the fail_min.', 'Value is greater than the fail_max.']
- flag_assessments :
- ['Bad', 'Bad', 'Bad']
- fail_min :
- 0.0
- fail_max :
- 1.75
- standard_name :
- quality_flag
Array Chunk Bytes 16.88 kiB 16.88 kiB Shape (4320,) (4320,) Count 2 Graph Layers 1 Chunks Type int32 numpy.ndarray - diffuse_hemisp_narrowband_filter5(time)float32dask.array<chunksize=(4320,), meta=np.ndarray>
- long_name :
- Narrowband diffuse hemispheric irradiance, filter 5, offset and cosine corrected
- units :
- W/(m^2 nm)
- explanation_of_narrowband_channel :
- The nominal center wavelength is 870 nm, nominal half-power width is 10 nm
- corrections :
- offset subtracted, cosine corrected
- centroid_wavelength :
- 869.3 nm
- FWHM :
- 10.0 nm
- ancillary_variables :
- qc_diffuse_hemisp_narrowband_filter5
Array Chunk Bytes 16.88 kiB 16.88 kiB Shape (4320,) (4320,) Count 2 Graph Layers 1 Chunks Type float32 numpy.ndarray - qc_diffuse_hemisp_narrowband_filter5(time)int32dask.array<chunksize=(4320,), meta=np.ndarray>
- long_name :
- Quality check results on field: Narrowband diffuse hemispheric irradiance, filter 5, offset and cosine corrected
- units :
- 1
- flag_masks :
- [1, 2, 4]
- flag_meanings :
- ['Value is equal to missing_value.', 'Value is less than the fail_min.', 'Value is greater than the fail_max.']
- flag_assessments :
- ['Bad', 'Bad', 'Bad']
- fail_min :
- 0.0
- fail_max :
- 1.25
- standard_name :
- quality_flag
Array Chunk Bytes 16.88 kiB 16.88 kiB Shape (4320,) (4320,) Count 2 Graph Layers 1 Chunks Type int32 numpy.ndarray - diffuse_hemisp_narrowband_filter6(time)float32dask.array<chunksize=(4320,), meta=np.ndarray>
- long_name :
- Narrowband diffuse hemispheric irradiance, filter 6, offset and cosine corrected
- units :
- W/(m^2 nm)
- explanation_of_narrowband_channel :
- The nominal center wavelength is 940 nm, nominal half-power width is 10 nm
- corrections :
- offset subtracted, cosine corrected
- centroid_wavelength :
- 939.4 nm
- FWHM :
- 6.7 nm
- ancillary_variables :
- qc_diffuse_hemisp_narrowband_filter6
Array Chunk Bytes 16.88 kiB 16.88 kiB Shape (4320,) (4320,) Count 2 Graph Layers 1 Chunks Type float32 numpy.ndarray - qc_diffuse_hemisp_narrowband_filter6(time)int32dask.array<chunksize=(4320,), meta=np.ndarray>
- long_name :
- Quality check results on field: Narrowband diffuse hemispheric irradiance, filter 6, offset and cosine corrected
- units :
- 1
- flag_masks :
- [1, 2, 4]
- flag_meanings :
- ['Value is equal to missing_value.', 'Value is less than the fail_min.', 'Value is greater than the fail_max.']
- flag_assessments :
- ['Bad', 'Bad', 'Bad']
- fail_min :
- 0.0
- fail_max :
- 1.5
- standard_name :
- quality_flag
Array Chunk Bytes 16.88 kiB 16.88 kiB Shape (4320,) (4320,) Count 2 Graph Layers 1 Chunks Type int32 numpy.ndarray - diffuse_hemisp_narrowband_filter7(time)float32dask.array<chunksize=(4320,), meta=np.ndarray>
- long_name :
- Narrowband diffuse hemispheric irradiance, filter 7, offset and cosine corrected
- units :
- W/(m^2 nm)
- explanation_of_narrowband_channel :
- The nominal center wavelength is 1625 nm, nominal half-power width is 10 nm
- corrections :
- offset subtracted, cosine corrected
- centroid_wavelength :
- 1624.2 nm
- FWHM :
- 14.8 nm
- ancillary_variables :
- qc_diffuse_hemisp_narrowband_filter7
Array Chunk Bytes 16.88 kiB 16.88 kiB Shape (4320,) (4320,) Count 2 Graph Layers 1 Chunks Type float32 numpy.ndarray - qc_diffuse_hemisp_narrowband_filter7(time)int32dask.array<chunksize=(4320,), meta=np.ndarray>
- long_name :
- Quality check results on field: Narrowband diffuse hemispheric irradiance, filter 7, offset and cosine corrected
- units :
- 1
- flag_masks :
- [1, 2, 4]
- flag_meanings :
- ['Value is equal to missing_value.', 'Value is less than the fail_min.', 'Value is greater than the fail_max.']
- flag_assessments :
- ['Bad', 'Bad', 'Bad']
- fail_min :
- 0.0
- fail_max :
- 5.0
- standard_name :
- quality_flag
Array Chunk Bytes 16.88 kiB 16.88 kiB Shape (4320,) (4320,) Count 2 Graph Layers 1 Chunks Type int32 numpy.ndarray - direct_normal_narrowband_filter1(time)float32dask.array<chunksize=(4320,), meta=np.ndarray>
- long_name :
- Narrowband direct normal irradiance, filter 1, cosine corrected
- units :
- W/(m^2 nm)
- explanation_of_narrowband_channel :
- The nominal center wavelength is 415 nm, nominal half-power width is 10 nm
- corrections :
- cosine corrected
- centroid_wavelength :
- 413.3 nm
- FWHM :
- 10.9 nm
- ancillary_variables :
- qc_direct_normal_narrowband_filter1
Array Chunk Bytes 16.88 kiB 16.88 kiB Shape (4320,) (4320,) Count 2 Graph Layers 1 Chunks Type float32 numpy.ndarray - qc_direct_normal_narrowband_filter1(time)int32dask.array<chunksize=(4320,), meta=np.ndarray>
- long_name :
- Quality check results on field: Narrowband direct normal irradiance, filter 1, cosine corrected
- units :
- 1
- flag_masks :
- [1, 2, 4]
- flag_meanings :
- ['Value is equal to missing_value.', 'Value is less than the fail_min.', 'Value is greater than the fail_max.']
- flag_assessments :
- ['Bad', 'Bad', 'Bad']
- fail_min :
- 0.0
- fail_max :
- 1.875
- standard_name :
- quality_flag
Array Chunk Bytes 16.88 kiB 16.88 kiB Shape (4320,) (4320,) Count 2 Graph Layers 1 Chunks Type int32 numpy.ndarray - direct_normal_narrowband_filter2(time)float32dask.array<chunksize=(4320,), meta=np.ndarray>
- long_name :
- Narrowband direct normal irradiance, filter 2, cosine corrected
- units :
- W/(m^2 nm)
- explanation_of_narrowband_channel :
- The nominal center wavelength is 500 nm, nominal half-power width is 10 nm
- corrections :
- cosine corrected
- centroid_wavelength :
- 501.0 nm
- FWHM :
- 10.8 nm
- ancillary_variables :
- qc_direct_normal_narrowband_filter2
Array Chunk Bytes 16.88 kiB 16.88 kiB Shape (4320,) (4320,) Count 2 Graph Layers 1 Chunks Type float32 numpy.ndarray - qc_direct_normal_narrowband_filter2(time)int32dask.array<chunksize=(4320,), meta=np.ndarray>
- long_name :
- Quality check results on field: Narrowband direct normal irradiance, filter 2, cosine corrected
- units :
- 1
- flag_masks :
- [1, 2, 4]
- flag_meanings :
- ['Value is equal to missing_value.', 'Value is less than the fail_min.', 'Value is greater than the fail_max.']
- flag_assessments :
- ['Bad', 'Bad', 'Bad']
- fail_min :
- 0.0
- fail_max :
- 2.0
- standard_name :
- quality_flag
Array Chunk Bytes 16.88 kiB 16.88 kiB Shape (4320,) (4320,) Count 2 Graph Layers 1 Chunks Type int32 numpy.ndarray - direct_normal_narrowband_filter3(time)float32dask.array<chunksize=(4320,), meta=np.ndarray>
- long_name :
- Narrowband direct normal irradiance, filter 3, cosine corrected
- units :
- W/(m^2 nm)
- explanation_of_narrowband_channel :
- The nominal center wavelength is 615 nm, nominal half-power width is 10 nm
- corrections :
- cosine corrected
- centroid_wavelength :
- 613.5 nm
- FWHM :
- 10.8 nm
- ancillary_variables :
- qc_direct_normal_narrowband_filter3
Array Chunk Bytes 16.88 kiB 16.88 kiB Shape (4320,) (4320,) Count 2 Graph Layers 1 Chunks Type float32 numpy.ndarray - qc_direct_normal_narrowband_filter3(time)int32dask.array<chunksize=(4320,), meta=np.ndarray>
- long_name :
- Quality check results on field: Narrowband direct normal irradiance, filter 3, cosine corrected
- units :
- 1
- flag_masks :
- [1, 2, 4]
- flag_meanings :
- ['Value is equal to missing_value.', 'Value is less than the fail_min.', 'Value is greater than the fail_max.']
- flag_assessments :
- ['Bad', 'Bad', 'Bad']
- fail_min :
- 0.0
- fail_max :
- 1.875
- standard_name :
- quality_flag
Array Chunk Bytes 16.88 kiB 16.88 kiB Shape (4320,) (4320,) Count 2 Graph Layers 1 Chunks Type int32 numpy.ndarray - direct_normal_narrowband_filter4(time)float32dask.array<chunksize=(4320,), meta=np.ndarray>
- long_name :
- Narrowband direct normal irradiance, filter 4, cosine corrected
- units :
- W/(m^2 nm)
- explanation_of_narrowband_channel :
- The nominal center wavelength is 673 nm, nominal half-power width is 10 nm
- corrections :
- cosine corrected
- centroid_wavelength :
- 671.4 nm
- FWHM :
- 10.5 nm
- ancillary_variables :
- qc_direct_normal_narrowband_filter4
Array Chunk Bytes 16.88 kiB 16.88 kiB Shape (4320,) (4320,) Count 2 Graph Layers 1 Chunks Type float32 numpy.ndarray - qc_direct_normal_narrowband_filter4(time)int32dask.array<chunksize=(4320,), meta=np.ndarray>
- long_name :
- Quality check results on field: Narrowband direct normal irradiance, filter 4, cosine corrected
- units :
- 1
- flag_masks :
- [1, 2, 4]
- flag_meanings :
- ['Value is equal to missing_value.', 'Value is less than the fail_min.', 'Value is greater than the fail_max.']
- flag_assessments :
- ['Bad', 'Bad', 'Bad']
- fail_min :
- 0.0
- fail_max :
- 1.75
- standard_name :
- quality_flag
Array Chunk Bytes 16.88 kiB 16.88 kiB Shape (4320,) (4320,) Count 2 Graph Layers 1 Chunks Type int32 numpy.ndarray - direct_normal_narrowband_filter5(time)float32dask.array<chunksize=(4320,), meta=np.ndarray>
- long_name :
- Narrowband direct normal irradiance, filter 5, cosine corrected
- units :
- W/(m^2 nm)
- explanation_of_narrowband_channel :
- The nominal center wavelength is 870 nm, nominal half-power width is 10 nm
- corrections :
- cosine corrected
- centroid_wavelength :
- 869.3 nm
- FWHM :
- 10.0 nm
- ancillary_variables :
- qc_direct_normal_narrowband_filter5
Array Chunk Bytes 16.88 kiB 16.88 kiB Shape (4320,) (4320,) Count 2 Graph Layers 1 Chunks Type float32 numpy.ndarray - qc_direct_normal_narrowband_filter5(time)int32dask.array<chunksize=(4320,), meta=np.ndarray>
- long_name :
- Quality check results on field: Narrowband direct normal irradiance, filter 5, cosine corrected
- units :
- 1
- flag_masks :
- [1, 2, 4]
- flag_meanings :
- ['Value is equal to missing_value.', 'Value is less than the fail_min.', 'Value is greater than the fail_max.']
- flag_assessments :
- ['Bad', 'Bad', 'Bad']
- fail_min :
- 0.0
- fail_max :
- 1.25
- standard_name :
- quality_flag
Array Chunk Bytes 16.88 kiB 16.88 kiB Shape (4320,) (4320,) Count 2 Graph Layers 1 Chunks Type int32 numpy.ndarray - direct_normal_narrowband_filter6(time)float32dask.array<chunksize=(4320,), meta=np.ndarray>
- long_name :
- Narrowband direct normal irradiance, filter 6, cosine corrected
- units :
- W/(m^2 nm)
- explanation_of_narrowband_channel :
- The nominal center wavelength is 940 nm, nominal half-power width is 10 nm
- corrections :
- cosine corrected
- centroid_wavelength :
- 939.4 nm
- FWHM :
- 6.7 nm
- ancillary_variables :
- qc_direct_normal_narrowband_filter6
Array Chunk Bytes 16.88 kiB 16.88 kiB Shape (4320,) (4320,) Count 2 Graph Layers 1 Chunks Type float32 numpy.ndarray - qc_direct_normal_narrowband_filter6(time)int32dask.array<chunksize=(4320,), meta=np.ndarray>
- long_name :
- Quality check results on field: Narrowband direct normal irradiance, filter 6, cosine corrected
- units :
- 1
- flag_masks :
- [1, 2, 4]
- flag_meanings :
- ['Value is equal to missing_value.', 'Value is less than the fail_min.', 'Value is greater than the fail_max.']
- flag_assessments :
- ['Bad', 'Bad', 'Bad']
- fail_min :
- 0.0
- fail_max :
- 1.5
- standard_name :
- quality_flag
Array Chunk Bytes 16.88 kiB 16.88 kiB Shape (4320,) (4320,) Count 2 Graph Layers 1 Chunks Type int32 numpy.ndarray - direct_normal_narrowband_filter7(time)float32dask.array<chunksize=(4320,), meta=np.ndarray>
- long_name :
- Narrowband direct normal irradiance, filter 7, cosine corrected
- units :
- W/(m^2 nm)
- explanation_of_narrowband_channel :
- The nominal center wavelength is 1625 nm, nominal half-power width is 10 nm
- corrections :
- cosine corrected
- centroid_wavelength :
- 1624.2 nm
- FWHM :
- 14.8 nm
- ancillary_variables :
- qc_direct_normal_narrowband_filter7
Array Chunk Bytes 16.88 kiB 16.88 kiB Shape (4320,) (4320,) Count 2 Graph Layers 1 Chunks Type float32 numpy.ndarray - qc_direct_normal_narrowband_filter7(time)int32dask.array<chunksize=(4320,), meta=np.ndarray>
- long_name :
- Quality check results on field: Narrowband direct normal irradiance, filter 7, cosine corrected
- units :
- 1
- flag_masks :
- [1, 2, 4]
- flag_meanings :
- ['Value is equal to missing_value.', 'Value is less than the fail_min.', 'Value is greater than the fail_max.']
- flag_assessments :
- ['Bad', 'Bad', 'Bad']
- fail_min :
- 0.0
- fail_max :
- 5.0
- standard_name :
- quality_flag
Array Chunk Bytes 16.88 kiB 16.88 kiB Shape (4320,) (4320,) Count 2 Graph Layers 1 Chunks Type int32 numpy.ndarray - alltime_hemisp_narrowband_filter1(time)float32dask.array<chunksize=(4320,), meta=np.ndarray>
- long_name :
- Alltime narrowband hemispheric irradiance, filter 1
- units :
- mV
- explanation_of_narrowband_channel :
- The nominal center wavelength is 415 nm, nominal half-power width is 10 nm
- corrections :
- none
- centroid_wavelength :
- 413.3 nm
- FWHM :
- 10.9 nm
- ancillary_variables :
- qc_alltime_hemisp_narrowband_filter1
Array Chunk Bytes 16.88 kiB 16.88 kiB Shape (4320,) (4320,) Count 2 Graph Layers 1 Chunks Type float32 numpy.ndarray - qc_alltime_hemisp_narrowband_filter1(time)int32dask.array<chunksize=(4320,), meta=np.ndarray>
- long_name :
- Quality check results on field: Alltime narrowband hemispheric irradiance, filter 1
- units :
- 1
- flag_masks :
- [1, 2, 4]
- flag_meanings :
- ['Value is equal to missing_value.', 'Value is less than the fail_min.', 'Value is greater than the fail_max.']
- flag_assessments :
- ['Bad', 'Bad', 'Bad']
- fail_min :
- -50.0
- fail_max :
- 4095.0
- standard_name :
- quality_flag
Array Chunk Bytes 16.88 kiB 16.88 kiB Shape (4320,) (4320,) Count 2 Graph Layers 1 Chunks Type int32 numpy.ndarray - alltime_hemisp_narrowband_filter2(time)float32dask.array<chunksize=(4320,), meta=np.ndarray>
- long_name :
- Alltime narrowband hemispheric irradiance, filter 2
- units :
- mV
- explanation_of_narrowband_channel :
- The nominal center wavelength is 500 nm, nominal half-power width is 10 nm
- corrections :
- none
- centroid_wavelength :
- 501.0 nm
- FWHM :
- 10.8 nm
- ancillary_variables :
- qc_alltime_hemisp_narrowband_filter2
Array Chunk Bytes 16.88 kiB 16.88 kiB Shape (4320,) (4320,) Count 2 Graph Layers 1 Chunks Type float32 numpy.ndarray - qc_alltime_hemisp_narrowband_filter2(time)int32dask.array<chunksize=(4320,), meta=np.ndarray>
- long_name :
- Quality check results on field: Alltime narrowband hemispheric irradiance, filter 2
- units :
- 1
- flag_masks :
- [1, 2, 4]
- flag_meanings :
- ['Value is equal to missing_value.', 'Value is less than the fail_min.', 'Value is greater than the fail_max.']
- flag_assessments :
- ['Bad', 'Bad', 'Bad']
- fail_min :
- -50.0
- fail_max :
- 4095.0
- standard_name :
- quality_flag
Array Chunk Bytes 16.88 kiB 16.88 kiB Shape (4320,) (4320,) Count 2 Graph Layers 1 Chunks Type int32 numpy.ndarray - alltime_hemisp_narrowband_filter3(time)float32dask.array<chunksize=(4320,), meta=np.ndarray>
- long_name :
- Alltime narrowband hemispheric irradiance, filter 3
- units :
- mV
- explanation_of_narrowband_channel :
- The nominal center wavelength is 615 nm, nominal half-power width is 10 nm
- corrections :
- none
- centroid_wavelength :
- 613.5 nm
- FWHM :
- 10.8 nm
- ancillary_variables :
- qc_alltime_hemisp_narrowband_filter3
Array Chunk Bytes 16.88 kiB 16.88 kiB Shape (4320,) (4320,) Count 2 Graph Layers 1 Chunks Type float32 numpy.ndarray - qc_alltime_hemisp_narrowband_filter3(time)int32dask.array<chunksize=(4320,), meta=np.ndarray>
- long_name :
- Quality check results on field: Alltime narrowband hemispheric irradiance, filter 3
- units :
- 1
- flag_masks :
- [1, 2, 4]
- flag_meanings :
- ['Value is equal to missing_value.', 'Value is less than the fail_min.', 'Value is greater than the fail_max.']
- flag_assessments :
- ['Bad', 'Bad', 'Bad']
- fail_min :
- -50.0
- fail_max :
- 4095.0
- standard_name :
- quality_flag
Array Chunk Bytes 16.88 kiB 16.88 kiB Shape (4320,) (4320,) Count 2 Graph Layers 1 Chunks Type int32 numpy.ndarray - alltime_hemisp_narrowband_filter4(time)float32dask.array<chunksize=(4320,), meta=np.ndarray>
- long_name :
- Alltime narrowband hemispheric irradiance, filter 4
- units :
- mV
- explanation_of_narrowband_channel :
- The nominal center wavelength is 673 nm, nominal half-power width is 10 nm
- corrections :
- none
- centroid_wavelength :
- 671.4 nm
- FWHM :
- 10.5 nm
- ancillary_variables :
- qc_alltime_hemisp_narrowband_filter4
Array Chunk Bytes 16.88 kiB 16.88 kiB Shape (4320,) (4320,) Count 2 Graph Layers 1 Chunks Type float32 numpy.ndarray - qc_alltime_hemisp_narrowband_filter4(time)int32dask.array<chunksize=(4320,), meta=np.ndarray>
- long_name :
- Quality check results on field: Alltime narrowband hemispheric irradiance, filter 4
- units :
- 1
- flag_masks :
- [1, 2, 4]
- flag_meanings :
- ['Value is equal to missing_value.', 'Value is less than the fail_min.', 'Value is greater than the fail_max.']
- flag_assessments :
- ['Bad', 'Bad', 'Bad']
- fail_min :
- -50.0
- fail_max :
- 4095.0
- standard_name :
- quality_flag
Array Chunk Bytes 16.88 kiB 16.88 kiB Shape (4320,) (4320,) Count 2 Graph Layers 1 Chunks Type int32 numpy.ndarray - alltime_hemisp_narrowband_filter5(time)float32dask.array<chunksize=(4320,), meta=np.ndarray>
- long_name :
- Alltime narrowband hemispheric irradiance, filter 5
- units :
- mV
- explanation_of_narrowband_channel :
- The nominal center wavelength is 870 nm, nominal half-power width is 10 nm
- corrections :
- none
- centroid_wavelength :
- 869.3 nm
- FWHM :
- 10.0 nm
- ancillary_variables :
- qc_alltime_hemisp_narrowband_filter5
Array Chunk Bytes 16.88 kiB 16.88 kiB Shape (4320,) (4320,) Count 2 Graph Layers 1 Chunks Type float32 numpy.ndarray - qc_alltime_hemisp_narrowband_filter5(time)int32dask.array<chunksize=(4320,), meta=np.ndarray>
- long_name :
- Quality check results on field: Alltime narrowband hemispheric irradiance, filter 5
- units :
- 1
- flag_masks :
- [1, 2, 4]
- flag_meanings :
- ['Value is equal to missing_value.', 'Value is less than the fail_min.', 'Value is greater than the fail_max.']
- flag_assessments :
- ['Bad', 'Bad', 'Bad']
- fail_min :
- -50.0
- fail_max :
- 4095.0
- standard_name :
- quality_flag
Array Chunk Bytes 16.88 kiB 16.88 kiB Shape (4320,) (4320,) Count 2 Graph Layers 1 Chunks Type int32 numpy.ndarray - alltime_hemisp_narrowband_filter6(time)float32dask.array<chunksize=(4320,), meta=np.ndarray>
- long_name :
- Alltime narrowband hemispheric irradiance, filter 6
- units :
- mV
- explanation_of_narrowband_channel :
- The nominal center wavelength is 940 nm, nominal half-power width is 10 nm
- corrections :
- none
- centroid_wavelength :
- 939.4 nm
- FWHM :
- 6.7 nm
- ancillary_variables :
- qc_alltime_hemisp_narrowband_filter6
Array Chunk Bytes 16.88 kiB 16.88 kiB Shape (4320,) (4320,) Count 2 Graph Layers 1 Chunks Type float32 numpy.ndarray - qc_alltime_hemisp_narrowband_filter6(time)int32dask.array<chunksize=(4320,), meta=np.ndarray>
- long_name :
- Quality check results on field: Alltime narrowband hemispheric irradiance, filter 6
- units :
- 1
- flag_masks :
- [1, 2, 4]
- flag_meanings :
- ['Value is equal to missing_value.', 'Value is less than the fail_min.', 'Value is greater than the fail_max.']
- flag_assessments :
- ['Bad', 'Bad', 'Bad']
- fail_min :
- -50.0
- fail_max :
- 4095.0
- standard_name :
- quality_flag
Array Chunk Bytes 16.88 kiB 16.88 kiB Shape (4320,) (4320,) Count 2 Graph Layers 1 Chunks Type int32 numpy.ndarray - alltime_hemisp_narrowband_filter7(time)float32dask.array<chunksize=(4320,), meta=np.ndarray>
- long_name :
- Alltime narrowband hemispheric irradiance, filter 7
- units :
- mV
- explanation_of_narrowband_channel :
- The nominal center wavelength is 1625 nm, nominal half-power width is 10 nm
- corrections :
- none
- centroid_wavelength :
- 1624.2 nm
- FWHM :
- 14.8 nm
- ancillary_variables :
- qc_alltime_hemisp_narrowband_filter7
Array Chunk Bytes 16.88 kiB 16.88 kiB Shape (4320,) (4320,) Count 2 Graph Layers 1 Chunks Type float32 numpy.ndarray - qc_alltime_hemisp_narrowband_filter7(time)int32dask.array<chunksize=(4320,), meta=np.ndarray>
- long_name :
- Quality check results on field: Alltime narrowband hemispheric irradiance, filter 7
- units :
- 1
- flag_masks :
- [1, 2, 4]
- flag_meanings :
- ['Value is equal to missing_value.', 'Value is less than the fail_min.', 'Value is greater than the fail_max.']
- flag_assessments :
- ['Bad', 'Bad', 'Bad']
- fail_min :
- -50.0
- fail_max :
- 4095.0
- standard_name :
- quality_flag
Array Chunk Bytes 16.88 kiB 16.88 kiB Shape (4320,) (4320,) Count 2 Graph Layers 1 Chunks Type int32 numpy.ndarray - direct_horizontal_narrowband_filter1(time)float32dask.array<chunksize=(4320,), meta=np.ndarray>
- long_name :
- Narrowband direct horizontal irradiance, filter 1, cosine corrected
- units :
- W/(m^2 nm)
- explanation_of_narrowband_channel :
- The nominal center wavelength is 415 nm, nominal half-power width is 10 nm
- corrections :
- cosine corrected
- centroid_wavelength :
- 413.3 nm
- FWHM :
- 10.9 nm
- ancillary_variables :
- qc_direct_horizontal_narrowband_filter1
Array Chunk Bytes 16.88 kiB 16.88 kiB Shape (4320,) (4320,) Count 2 Graph Layers 1 Chunks Type float32 numpy.ndarray - qc_direct_horizontal_narrowband_filter1(time)int32dask.array<chunksize=(4320,), meta=np.ndarray>
- long_name :
- Quality check results on field: Narrowband direct horizontal irradiance, filter 1, cosine corrected
- units :
- 1
- flag_masks :
- [1, 2, 4]
- flag_meanings :
- ['Value is equal to missing_value.', 'Value is less than the fail_min.', 'Value is greater than the fail_max.']
- flag_assessments :
- ['Bad', 'Bad', 'Bad']
- fail_min :
- 0.0
- fail_max :
- 1.875
- standard_name :
- quality_flag
Array Chunk Bytes 16.88 kiB 16.88 kiB Shape (4320,) (4320,) Count 2 Graph Layers 1 Chunks Type int32 numpy.ndarray - direct_horizontal_narrowband_filter2(time)float32dask.array<chunksize=(4320,), meta=np.ndarray>
- long_name :
- Narrowband direct horizontal irradiance, filter 2, cosine corrected
- units :
- W/(m^2 nm)
- explanation_of_narrowband_channel :
- The nominal center wavelength is 500 nm, nominal half-power width is 10 nm
- corrections :
- cosine corrected
- centroid_wavelength :
- 501.0 nm
- FWHM :
- 10.8 nm
- ancillary_variables :
- qc_direct_horizontal_narrowband_filter2
Array Chunk Bytes 16.88 kiB 16.88 kiB Shape (4320,) (4320,) Count 2 Graph Layers 1 Chunks Type float32 numpy.ndarray - qc_direct_horizontal_narrowband_filter2(time)int32dask.array<chunksize=(4320,), meta=np.ndarray>
- long_name :
- Quality check results on field: Narrowband direct horizontal irradiance, filter 2, cosine corrected
- units :
- 1
- flag_masks :
- [1, 2, 4]
- flag_meanings :
- ['Value is equal to missing_value.', 'Value is less than the fail_min.', 'Value is greater than the fail_max.']
- flag_assessments :
- ['Bad', 'Bad', 'Bad']
- fail_min :
- 0.0
- fail_max :
- 2.0
- standard_name :
- quality_flag
Array Chunk Bytes 16.88 kiB 16.88 kiB Shape (4320,) (4320,) Count 2 Graph Layers 1 Chunks Type int32 numpy.ndarray - direct_horizontal_narrowband_filter3(time)float32dask.array<chunksize=(4320,), meta=np.ndarray>
- long_name :
- Narrowband direct horizontal irradiance, filter 3, cosine corrected
- units :
- W/(m^2 nm)
- explanation_of_narrowband_channel :
- The nominal center wavelength is 615 nm, nominal half-power width is 10 nm
- corrections :
- cosine corrected
- centroid_wavelength :
- 613.5 nm
- FWHM :
- 10.8 nm
- ancillary_variables :
- qc_direct_horizontal_narrowband_filter3
Array Chunk Bytes 16.88 kiB 16.88 kiB Shape (4320,) (4320,) Count 2 Graph Layers 1 Chunks Type float32 numpy.ndarray - qc_direct_horizontal_narrowband_filter3(time)int32dask.array<chunksize=(4320,), meta=np.ndarray>
- long_name :
- Quality check results on field: Narrowband direct horizontal irradiance, filter 3, cosine corrected
- units :
- 1
- flag_masks :
- [1, 2, 4]
- flag_meanings :
- ['Value is equal to missing_value.', 'Value is less than the fail_min.', 'Value is greater than the fail_max.']
- flag_assessments :
- ['Bad', 'Bad', 'Bad']
- fail_min :
- 0.0
- fail_max :
- 1.875
- standard_name :
- quality_flag
Array Chunk Bytes 16.88 kiB 16.88 kiB Shape (4320,) (4320,) Count 2 Graph Layers 1 Chunks Type int32 numpy.ndarray - direct_horizontal_narrowband_filter4(time)float32dask.array<chunksize=(4320,), meta=np.ndarray>
- long_name :
- Narrowband direct horizontal irradiance, filter 4, cosine corrected
- units :
- W/(m^2 nm)
- explanation_of_narrowband_channel :
- The nominal center wavelength is 673 nm, nominal half-power width is 10 nm
- corrections :
- cosine corrected
- centroid_wavelength :
- 671.4 nm
- FWHM :
- 10.5 nm
- ancillary_variables :
- qc_direct_horizontal_narrowband_filter4
Array Chunk Bytes 16.88 kiB 16.88 kiB Shape (4320,) (4320,) Count 2 Graph Layers 1 Chunks Type float32 numpy.ndarray - qc_direct_horizontal_narrowband_filter4(time)int32dask.array<chunksize=(4320,), meta=np.ndarray>
- long_name :
- Quality check results on field: Narrowband direct horizontal irradiance, filter 4, cosine corrected
- units :
- 1
- flag_masks :
- [1, 2, 4]
- flag_meanings :
- ['Value is equal to missing_value.', 'Value is less than the fail_min.', 'Value is greater than the fail_max.']
- flag_assessments :
- ['Bad', 'Bad', 'Bad']
- fail_min :
- 0.0
- fail_max :
- 1.75
- standard_name :
- quality_flag
Array Chunk Bytes 16.88 kiB 16.88 kiB Shape (4320,) (4320,) Count 2 Graph Layers 1 Chunks Type int32 numpy.ndarray - direct_horizontal_narrowband_filter5(time)float32dask.array<chunksize=(4320,), meta=np.ndarray>
- long_name :
- Narrowband direct horizontal irradiance, filter 5, cosine corrected
- units :
- W/(m^2 nm)
- explanation_of_narrowband_channel :
- The nominal center wavelength is 870 nm, nominal half-power width is 10 nm
- corrections :
- cosine corrected
- centroid_wavelength :
- 869.3 nm
- FWHM :
- 10.0 nm
- ancillary_variables :
- qc_direct_horizontal_narrowband_filter5
Array Chunk Bytes 16.88 kiB 16.88 kiB Shape (4320,) (4320,) Count 2 Graph Layers 1 Chunks Type float32 numpy.ndarray - qc_direct_horizontal_narrowband_filter5(time)int32dask.array<chunksize=(4320,), meta=np.ndarray>
- long_name :
- Quality check results on field: Narrowband direct horizontal irradiance, filter 5, cosine corrected
- units :
- 1
- flag_masks :
- [1, 2, 4]
- flag_meanings :
- ['Value is equal to missing_value.', 'Value is less than the fail_min.', 'Value is greater than the fail_max.']
- flag_assessments :
- ['Bad', 'Bad', 'Bad']
- fail_min :
- 0.0
- fail_max :
- 1.25
- standard_name :
- quality_flag
Array Chunk Bytes 16.88 kiB 16.88 kiB Shape (4320,) (4320,) Count 2 Graph Layers 1 Chunks Type int32 numpy.ndarray - direct_horizontal_narrowband_filter6(time)float32dask.array<chunksize=(4320,), meta=np.ndarray>
- long_name :
- Narrowband direct horizontal irradiance, filter 6, cosine corrected
- units :
- W/(m^2 nm)
- explanation_of_narrowband_channel :
- The nominal center wavelength is 940 nm, nominal half-power width is 10 nm
- corrections :
- cosine corrected
- centroid_wavelength :
- 939.4 nm
- FWHM :
- 6.7 nm
- ancillary_variables :
- qc_direct_horizontal_narrowband_filter6
Array Chunk Bytes 16.88 kiB 16.88 kiB Shape (4320,) (4320,) Count 2 Graph Layers 1 Chunks Type float32 numpy.ndarray - qc_direct_horizontal_narrowband_filter6(time)int32dask.array<chunksize=(4320,), meta=np.ndarray>
- long_name :
- Quality check results on field: Narrowband direct horizontal irradiance, filter 6, cosine corrected
- units :
- 1
- flag_masks :
- [1, 2, 4]
- flag_meanings :
- ['Value is equal to missing_value.', 'Value is less than the fail_min.', 'Value is greater than the fail_max.']
- flag_assessments :
- ['Bad', 'Bad', 'Bad']
- fail_min :
- 0.0
- fail_max :
- 1.5
- standard_name :
- quality_flag
Array Chunk Bytes 16.88 kiB 16.88 kiB Shape (4320,) (4320,) Count 2 Graph Layers 1 Chunks Type int32 numpy.ndarray - direct_horizontal_narrowband_filter7(time)float32dask.array<chunksize=(4320,), meta=np.ndarray>
- long_name :
- Narrowband direct horizontal irradiance, filter 7, cosine corrected
- units :
- W/(m^2 nm)
- explanation_of_narrowband_channel :
- The nominal center wavelength is 1625 nm, nominal half-power width is 10 nm
- corrections :
- cosine corrected
- centroid_wavelength :
- 1624.2 nm
- FWHM :
- 14.8 nm
- ancillary_variables :
- qc_direct_horizontal_narrowband_filter7
Array Chunk Bytes 16.88 kiB 16.88 kiB Shape (4320,) (4320,) Count 2 Graph Layers 1 Chunks Type float32 numpy.ndarray - qc_direct_horizontal_narrowband_filter7(time)int32dask.array<chunksize=(4320,), meta=np.ndarray>
- long_name :
- Quality check results on field: Narrowband direct horizontal irradiance, filter 7, cosine corrected
- units :
- 1
- flag_masks :
- [1, 2, 4]
- flag_meanings :
- ['Value is equal to missing_value.', 'Value is less than the fail_min.', 'Value is greater than the fail_max.']
- flag_assessments :
- ['Bad', 'Bad', 'Bad']
- fail_min :
- 0.0
- fail_max :
- 5.0
- standard_name :
- quality_flag
Array Chunk Bytes 16.88 kiB 16.88 kiB Shape (4320,) (4320,) Count 2 Graph Layers 1 Chunks Type int32 numpy.ndarray - direct_diffuse_ratio_filter1(time)float32dask.array<chunksize=(4320,), meta=np.ndarray>
- long_name :
- Ratio of direct_normal_filter1 to diffuse_hemisp_filter1
- units :
- 1
- centroid_wavelength :
- 413.3 nm
- FWHM :
- 10.9 nm
- ancillary_variables :
- qc_direct_diffuse_ratio_filter1
Array Chunk Bytes 16.88 kiB 16.88 kiB Shape (4320,) (4320,) Count 2 Graph Layers 1 Chunks Type float32 numpy.ndarray - qc_direct_diffuse_ratio_filter1(time)int32dask.array<chunksize=(4320,), meta=np.ndarray>
- long_name :
- Quality check results on field: Ratio of direct_normal_filter1 to diffuse_hemisp_filter1
- units :
- 1
- flag_masks :
- [1, 2, 4]
- flag_meanings :
- ['Value is equal to missing_value.', 'Value is less than the fail_min.', 'Value is greater than the fail_max.']
- flag_assessments :
- ['Bad', 'Bad', 'Bad']
- fail_min :
- 0.0
- fail_max :
- 5.0
- standard_name :
- quality_flag
Array Chunk Bytes 16.88 kiB 16.88 kiB Shape (4320,) (4320,) Count 2 Graph Layers 1 Chunks Type int32 numpy.ndarray - direct_diffuse_ratio_filter2(time)float32dask.array<chunksize=(4320,), meta=np.ndarray>
- long_name :
- Ratio of direct_normal_filter2 to diffuse_hemisp_filter2
- units :
- 1
- centroid_wavelength :
- 501.0 nm
- FWHM :
- 10.8 nm
- ancillary_variables :
- qc_direct_diffuse_ratio_filter2
Array Chunk Bytes 16.88 kiB 16.88 kiB Shape (4320,) (4320,) Count 2 Graph Layers 1 Chunks Type float32 numpy.ndarray - qc_direct_diffuse_ratio_filter2(time)int32dask.array<chunksize=(4320,), meta=np.ndarray>
- long_name :
- Quality check results on field: Ratio of direct_normal_filter2 to diffuse_hemisp_filter2
- units :
- 1
- flag_masks :
- [1, 2, 4]
- flag_meanings :
- ['Value is equal to missing_value.', 'Value is less than the fail_min.', 'Value is greater than the fail_max.']
- flag_assessments :
- ['Bad', 'Bad', 'Bad']
- fail_min :
- 0.0
- fail_max :
- 6.0
- standard_name :
- quality_flag
Array Chunk Bytes 16.88 kiB 16.88 kiB Shape (4320,) (4320,) Count 2 Graph Layers 1 Chunks Type int32 numpy.ndarray - direct_diffuse_ratio_filter3(time)float32dask.array<chunksize=(4320,), meta=np.ndarray>
- long_name :
- Ratio of direct_normal_filter3 to diffuse_hemisp_filter3
- units :
- 1
- centroid_wavelength :
- 613.5 nm
- FWHM :
- 10.8 nm
- ancillary_variables :
- qc_direct_diffuse_ratio_filter3
Array Chunk Bytes 16.88 kiB 16.88 kiB Shape (4320,) (4320,) Count 2 Graph Layers 1 Chunks Type float32 numpy.ndarray - qc_direct_diffuse_ratio_filter3(time)int32dask.array<chunksize=(4320,), meta=np.ndarray>
- long_name :
- Quality check results on field: Ratio of direct_normal_filter3 to diffuse_hemisp_filter3
- units :
- 1
- flag_masks :
- [1, 2, 4]
- flag_meanings :
- ['Value is equal to missing_value.', 'Value is less than the fail_min.', 'Value is greater than the fail_max.']
- flag_assessments :
- ['Bad', 'Bad', 'Bad']
- fail_min :
- 0.0
- fail_max :
- 10.0
- standard_name :
- quality_flag
Array Chunk Bytes 16.88 kiB 16.88 kiB Shape (4320,) (4320,) Count 2 Graph Layers 1 Chunks Type int32 numpy.ndarray - direct_diffuse_ratio_filter4(time)float32dask.array<chunksize=(4320,), meta=np.ndarray>
- long_name :
- Ratio of direct_normal_filter4 to diffuse_hemisp_filter4
- units :
- 1
- centroid_wavelength :
- 671.4 nm
- FWHM :
- 10.5 nm
- ancillary_variables :
- qc_direct_diffuse_ratio_filter4
Array Chunk Bytes 16.88 kiB 16.88 kiB Shape (4320,) (4320,) Count 2 Graph Layers 1 Chunks Type float32 numpy.ndarray - qc_direct_diffuse_ratio_filter4(time)int32dask.array<chunksize=(4320,), meta=np.ndarray>
- long_name :
- Quality check results on field: Ratio of direct_normal_filter4 to diffuse_hemisp_filter4
- units :
- 1
- flag_masks :
- [1, 2, 4]
- flag_meanings :
- ['Value is equal to missing_value.', 'Value is less than the fail_min.', 'Value is greater than the fail_max.']
- flag_assessments :
- ['Bad', 'Bad', 'Bad']
- fail_min :
- 0.0
- fail_max :
- 14.0
- standard_name :
- quality_flag
Array Chunk Bytes 16.88 kiB 16.88 kiB Shape (4320,) (4320,) Count 2 Graph Layers 1 Chunks Type int32 numpy.ndarray - direct_diffuse_ratio_filter5(time)float32dask.array<chunksize=(4320,), meta=np.ndarray>
- long_name :
- Ratio of direct_normal_filter5 to diffuse_hemisp_filter5
- units :
- 1
- centroid_wavelength :
- 869.3 nm
- FWHM :
- 10.0 nm
- ancillary_variables :
- qc_direct_diffuse_ratio_filter5
Array Chunk Bytes 16.88 kiB 16.88 kiB Shape (4320,) (4320,) Count 2 Graph Layers 1 Chunks Type float32 numpy.ndarray - qc_direct_diffuse_ratio_filter5(time)int32dask.array<chunksize=(4320,), meta=np.ndarray>
- long_name :
- Quality check results on field: Ratio of direct_normal_filter5 to diffuse_hemisp_filter5
- units :
- 1
- flag_masks :
- [1, 2, 4]
- flag_meanings :
- ['Value is equal to missing_value.', 'Value is less than the fail_min.', 'Value is greater than the fail_max.']
- flag_assessments :
- ['Bad', 'Bad', 'Bad']
- fail_min :
- 0.0
- fail_max :
- 25.0
- standard_name :
- quality_flag
Array Chunk Bytes 16.88 kiB 16.88 kiB Shape (4320,) (4320,) Count 2 Graph Layers 1 Chunks Type int32 numpy.ndarray - direct_diffuse_ratio_filter6(time)float32dask.array<chunksize=(4320,), meta=np.ndarray>
- long_name :
- Ratio of direct_normal_filter6 to diffuse_hemisp_filter6
- units :
- 1
- centroid_wavelength :
- 939.4 nm
- FWHM :
- 6.7 nm
- ancillary_variables :
- qc_direct_diffuse_ratio_filter6
Array Chunk Bytes 16.88 kiB 16.88 kiB Shape (4320,) (4320,) Count 2 Graph Layers 1 Chunks Type float32 numpy.ndarray - qc_direct_diffuse_ratio_filter6(time)int32dask.array<chunksize=(4320,), meta=np.ndarray>
- long_name :
- Quality check results on field: Ratio of direct_normal_filter6 to diffuse_hemisp_filter6
- units :
- 1
- flag_masks :
- [1, 2, 4]
- flag_meanings :
- ['Value is equal to missing_value.', 'Value is less than the fail_min.', 'Value is greater than the fail_max.']
- flag_assessments :
- ['Bad', 'Bad', 'Bad']
- fail_min :
- 0.0
- fail_max :
- 25.0
- standard_name :
- quality_flag
Array Chunk Bytes 16.88 kiB 16.88 kiB Shape (4320,) (4320,) Count 2 Graph Layers 1 Chunks Type int32 numpy.ndarray - direct_diffuse_ratio_filter7(time)float32dask.array<chunksize=(4320,), meta=np.ndarray>
- long_name :
- Ratio of direct_normal_filter6 to diffuse_hemisp_filter7
- units :
- 1
- centroid_wavelength :
- 1624.2 nm
- FWHM :
- 14.8 nm
- ancillary_variables :
- qc_direct_diffuse_ratio_filter7
Array Chunk Bytes 16.88 kiB 16.88 kiB Shape (4320,) (4320,) Count 2 Graph Layers 1 Chunks Type float32 numpy.ndarray - qc_direct_diffuse_ratio_filter7(time)int32dask.array<chunksize=(4320,), meta=np.ndarray>
- long_name :
- Quality check results on field: Ratio of direct_normal_filter6 to diffuse_hemisp_filter7
- units :
- 1
- flag_masks :
- [1, 2, 4]
- flag_meanings :
- ['Value is equal to missing_value.', 'Value is less than the fail_min.', 'Value is greater than the fail_max.']
- flag_assessments :
- ['Bad', 'Bad', 'Bad']
- fail_min :
- 0.0
- fail_max :
- 25.0
- standard_name :
- quality_flag
Array Chunk Bytes 16.88 kiB 16.88 kiB Shape (4320,) (4320,) Count 2 Graph Layers 1 Chunks Type int32 numpy.ndarray - head_temp(time)float32dask.array<chunksize=(4320,), meta=np.ndarray>
- long_name :
- Detector cluster temperature
- units :
- degC
- ancillary_variables :
- qc_head_temp
Array Chunk Bytes 16.88 kiB 16.88 kiB Shape (4320,) (4320,) Count 2 Graph Layers 1 Chunks Type float32 numpy.ndarray - qc_head_temp(time)int32dask.array<chunksize=(4320,), meta=np.ndarray>
- long_name :
- Quality check results on field: Detector cluster temperature
- units :
- 1
- flag_masks :
- [1, 2, 4]
- flag_meanings :
- ['Value is equal to missing_value.', 'Value is less than the fail_min.', 'Value is greater than the fail_max.']
- flag_assessments :
- ['Bad', 'Bad', 'Bad']
- fail_min :
- 30.0
- fail_max :
- 50.0
- standard_name :
- quality_flag
Array Chunk Bytes 16.88 kiB 16.88 kiB Shape (4320,) (4320,) Count 2 Graph Layers 1 Chunks Type int32 numpy.ndarray - head_temp2(time)float32dask.array<chunksize=(4320,), meta=np.ndarray>
- long_name :
- HTC300 Heater controller temperature
- units :
- degC
- ancillary_variables :
- qc_head_temp2
Array Chunk Bytes 16.88 kiB 16.88 kiB Shape (4320,) (4320,) Count 2 Graph Layers 1 Chunks Type float32 numpy.ndarray - qc_head_temp2(time)int32dask.array<chunksize=(4320,), meta=np.ndarray>
- long_name :
- Quality check results on field: HTC300 Heater controller temperature
- units :
- 1
- flag_masks :
- [1, 2, 4]
- flag_meanings :
- ['Value is equal to missing_value.', 'Value is less than the fail_min.', 'Value is greater than the fail_max.']
- flag_assessments :
- ['Bad', 'Bad', 'Bad']
- fail_min :
- 30.0
- fail_max :
- 50.0
- standard_name :
- quality_flag
Array Chunk Bytes 16.88 kiB 16.88 kiB Shape (4320,) (4320,) Count 2 Graph Layers 1 Chunks Type int32 numpy.ndarray - logger_temperature(time)float32dask.array<chunksize=(4320,), meta=np.ndarray>
- long_name :
- Internal logger temperature
- units :
- degC
- ancillary_variables :
- qc_logger_temperature
Array Chunk Bytes 16.88 kiB 16.88 kiB Shape (4320,) (4320,) Count 2 Graph Layers 1 Chunks Type float32 numpy.ndarray - qc_logger_temperature(time)int32dask.array<chunksize=(4320,), meta=np.ndarray>
- long_name :
- Quality check results on field: Internal logger temperature
- units :
- 1
- flag_masks :
- [1, 2, 4]
- flag_meanings :
- ['Value is equal to missing_value.', 'Value is less than the fail_min.', 'Value is greater than the fail_max.']
- flag_assessments :
- ['Bad', 'Bad', 'Bad']
- fail_min :
- -55.0
- fail_max :
- 85.0
- standard_name :
- quality_flag
Array Chunk Bytes 16.88 kiB 16.88 kiB Shape (4320,) (4320,) Count 2 Graph Layers 1 Chunks Type int32 numpy.ndarray - logger_volt(time)float32dask.array<chunksize=(4320,), meta=np.ndarray>
- long_name :
- Data logger supply voltage
- units :
- V
- ancillary_variables :
- qc_logger_volt
Array Chunk Bytes 16.88 kiB 16.88 kiB Shape (4320,) (4320,) Count 2 Graph Layers 1 Chunks Type float32 numpy.ndarray - qc_logger_volt(time)int32dask.array<chunksize=(4320,), meta=np.ndarray>
- long_name :
- Quality check results on field: Data logger supply voltage
- units :
- 1
- flag_masks :
- [1, 2, 4]
- flag_meanings :
- ['Value is equal to missing_value.', 'Value is less than the fail_min.', 'Value is greater than the fail_max.']
- flag_assessments :
- ['Bad', 'Bad', 'Bad']
- fail_min :
- 10.0
- fail_max :
- 15.0
- standard_name :
- quality_flag
Array Chunk Bytes 16.88 kiB 16.88 kiB Shape (4320,) (4320,) Count 2 Graph Layers 1 Chunks Type int32 numpy.ndarray - solar_zenith_angle(time)float32dask.array<chunksize=(4320,), meta=np.ndarray>
- long_name :
- Apparent solar zenith angle
- units :
- degree
- corrections :
- Solar zenith angle corrected for refraction by atmosphere
Array Chunk Bytes 16.88 kiB 16.88 kiB Shape (4320,) (4320,) Count 2 Graph Layers 1 Chunks Type float32 numpy.ndarray - cosine_solar_zenith_angle(time)float32dask.array<chunksize=(4320,), meta=np.ndarray>
- long_name :
- Cosine of apparent solar zenith angle
- units :
- 1
- corrections :
- Cosine solar zenith angle corrected for refraction by atmosphere
Array Chunk Bytes 16.88 kiB 16.88 kiB Shape (4320,) (4320,) Count 2 Graph Layers 1 Chunks Type float32 numpy.ndarray - elevation_angle(time)float32dask.array<chunksize=(4320,), meta=np.ndarray>
- long_name :
- Elevation angle
- units :
- degree
Array Chunk Bytes 16.88 kiB 16.88 kiB Shape (4320,) (4320,) Count 2 Graph Layers 1 Chunks Type float32 numpy.ndarray - airmass(time)float32dask.array<chunksize=(4320,), meta=np.ndarray>
- long_name :
- Airmass
- units :
- 1
- corrections :
- Approximately 1/cosine_solar_zenith_angle with corrections for refraction and spherical atmosphere
Array Chunk Bytes 16.88 kiB 16.88 kiB Shape (4320,) (4320,) Count 2 Graph Layers 1 Chunks Type float32 numpy.ndarray - azimuth_angle(time)float32dask.array<chunksize=(4320,), meta=np.ndarray>
- long_name :
- Azimuth angle
- units :
- degree
Array Chunk Bytes 16.88 kiB 16.88 kiB Shape (4320,) (4320,) Count 2 Graph Layers 1 Chunks Type float32 numpy.ndarray - computed_cosine_correction_filter1(time)float32dask.array<chunksize=(4320,), meta=np.ndarray>
- long_name :
- Cosine correction applied to direct_horizontal_filter1
- units :
- 1
- centroid_wavelength :
- 413.3 nm
- FWHM :
- 10.9 nm
Array Chunk Bytes 16.88 kiB 16.88 kiB Shape (4320,) (4320,) Count 2 Graph Layers 1 Chunks Type float32 numpy.ndarray - computed_cosine_correction_filter2(time)float32dask.array<chunksize=(4320,), meta=np.ndarray>
- long_name :
- Cosine correction applied to direct_horizontal_filter2
- units :
- 1
- centroid_wavelength :
- 501.0 nm
- FWHM :
- 10.8 nm
Array Chunk Bytes 16.88 kiB 16.88 kiB Shape (4320,) (4320,) Count 2 Graph Layers 1 Chunks Type float32 numpy.ndarray - computed_cosine_correction_filter3(time)float32dask.array<chunksize=(4320,), meta=np.ndarray>
- long_name :
- Cosine correction applied to direct_horizontal_filter3
- units :
- 1
- centroid_wavelength :
- 613.5 nm
- FWHM :
- 10.8 nm
Array Chunk Bytes 16.88 kiB 16.88 kiB Shape (4320,) (4320,) Count 2 Graph Layers 1 Chunks Type float32 numpy.ndarray - computed_cosine_correction_filter4(time)float32dask.array<chunksize=(4320,), meta=np.ndarray>
- long_name :
- Cosine correction applied to direct_horizontal_filter4
- units :
- 1
- centroid_wavelength :
- 671.4 nm
- FWHM :
- 10.5 nm
Array Chunk Bytes 16.88 kiB 16.88 kiB Shape (4320,) (4320,) Count 2 Graph Layers 1 Chunks Type float32 numpy.ndarray - computed_cosine_correction_filter5(time)float32dask.array<chunksize=(4320,), meta=np.ndarray>
- long_name :
- Cosine correction applied to direct_horizontal_filter5
- units :
- 1
- centroid_wavelength :
- 869.3 nm
- FWHM :
- 10.0 nm
Array Chunk Bytes 16.88 kiB 16.88 kiB Shape (4320,) (4320,) Count 2 Graph Layers 1 Chunks Type float32 numpy.ndarray - computed_cosine_correction_filter6(time)float32dask.array<chunksize=(4320,), meta=np.ndarray>
- long_name :
- Cosine correction applied to direct_horizontal_filter6
- units :
- 1
- centroid_wavelength :
- 939.4 nm
- FWHM :
- 6.7 nm
Array Chunk Bytes 16.88 kiB 16.88 kiB Shape (4320,) (4320,) Count 2 Graph Layers 1 Chunks Type float32 numpy.ndarray - computed_cosine_correction_filter7(time)float32dask.array<chunksize=(4320,), meta=np.ndarray>
- long_name :
- Cosine correction applied to direct_horizontal_filter7
- units :
- 1
- centroid_wavelength :
- 1624.2 nm
- FWHM :
- 14.8 nm
Array Chunk Bytes 16.88 kiB 16.88 kiB Shape (4320,) (4320,) Count 2 Graph Layers 1 Chunks Type float32 numpy.ndarray - cosine_correction_sn_filter1(bench_angle)float32dask.array<chunksize=(181,), meta=np.ndarray>
- long_name :
- Cosine correction, south to north, filter1
- units :
- 1
- comment :
- Read from cosine_correction_source
- centroid_wavelength :
- 413.3 nm
- FWHM :
- 10.9 nm
Array Chunk Bytes 724 B 724 B Shape (181,) (181,) Count 2 Graph Layers 1 Chunks Type float32 numpy.ndarray - cosine_correction_sn_filter2(bench_angle)float32dask.array<chunksize=(181,), meta=np.ndarray>
- long_name :
- Cosine correction, south to north, filter2
- units :
- 1
- comment :
- Read from cosine_correction_source
- centroid_wavelength :
- 501.0 nm
- FWHM :
- 10.8 nm
Array Chunk Bytes 724 B 724 B Shape (181,) (181,) Count 2 Graph Layers 1 Chunks Type float32 numpy.ndarray - cosine_correction_sn_filter3(bench_angle)float32dask.array<chunksize=(181,), meta=np.ndarray>
- long_name :
- Cosine correction, south to north, filter3
- units :
- 1
- comment :
- Read from cosine_correction_source
- centroid_wavelength :
- 613.5 nm
- FWHM :
- 10.8 nm
Array Chunk Bytes 724 B 724 B Shape (181,) (181,) Count 2 Graph Layers 1 Chunks Type float32 numpy.ndarray - cosine_correction_sn_filter4(bench_angle)float32dask.array<chunksize=(181,), meta=np.ndarray>
- long_name :
- Cosine correction, south to north, filter4
- units :
- 1
- comment :
- Read from cosine_correction_source
- centroid_wavelength :
- 671.4 nm
- FWHM :
- 10.5 nm
Array Chunk Bytes 724 B 724 B Shape (181,) (181,) Count 2 Graph Layers 1 Chunks Type float32 numpy.ndarray - cosine_correction_sn_filter5(bench_angle)float32dask.array<chunksize=(181,), meta=np.ndarray>
- long_name :
- Cosine correction, south to north, filter5
- units :
- 1
- comment :
- Read from cosine_correction_source
- centroid_wavelength :
- 869.3 nm
- FWHM :
- 10.0 nm
Array Chunk Bytes 724 B 724 B Shape (181,) (181,) Count 2 Graph Layers 1 Chunks Type float32 numpy.ndarray - cosine_correction_sn_filter6(bench_angle)float32dask.array<chunksize=(181,), meta=np.ndarray>
- long_name :
- Cosine correction, south to north, filter6
- units :
- 1
- comment :
- Read from cosine_correction_source
- centroid_wavelength :
- 939.4 nm
- FWHM :
- 6.7 nm
Array Chunk Bytes 724 B 724 B Shape (181,) (181,) Count 2 Graph Layers 1 Chunks Type float32 numpy.ndarray - cosine_correction_sn_filter7(bench_angle)float32dask.array<chunksize=(181,), meta=np.ndarray>
- long_name :
- Cosine correction, south to north, filter7
- units :
- 1
- comment :
- Read from cosine_correction_source
- centroid_wavelength :
- 1624.2 nm
- FWHM :
- 14.8 nm
Array Chunk Bytes 724 B 724 B Shape (181,) (181,) Count 2 Graph Layers 1 Chunks Type float32 numpy.ndarray - cosine_correction_we_filter1(bench_angle)float32dask.array<chunksize=(181,), meta=np.ndarray>
- long_name :
- Cosine correction, west to east, filter1
- units :
- 1
- comment :
- Read from cosine_correction_source
- centroid_wavelength :
- 413.3 nm
- FWHM :
- 10.9 nm
Array Chunk Bytes 724 B 724 B Shape (181,) (181,) Count 2 Graph Layers 1 Chunks Type float32 numpy.ndarray - cosine_correction_we_filter2(bench_angle)float32dask.array<chunksize=(181,), meta=np.ndarray>
- long_name :
- Cosine correction, west to east, filter2
- units :
- 1
- comment :
- Read from cosine_correction_source
- centroid_wavelength :
- 501.0 nm
- FWHM :
- 10.8 nm
Array Chunk Bytes 724 B 724 B Shape (181,) (181,) Count 2 Graph Layers 1 Chunks Type float32 numpy.ndarray - cosine_correction_we_filter3(bench_angle)float32dask.array<chunksize=(181,), meta=np.ndarray>
- long_name :
- Cosine correction, west to east, filter3
- units :
- 1
- comment :
- Read from cosine_correction_source
- centroid_wavelength :
- 613.5 nm
- FWHM :
- 10.8 nm
Array Chunk Bytes 724 B 724 B Shape (181,) (181,) Count 2 Graph Layers 1 Chunks Type float32 numpy.ndarray - cosine_correction_we_filter4(bench_angle)float32dask.array<chunksize=(181,), meta=np.ndarray>
- long_name :
- Cosine correction, west to east, filter4
- units :
- 1
- comment :
- Read from cosine_correction_source
- centroid_wavelength :
- 671.4 nm
- FWHM :
- 10.5 nm
Array Chunk Bytes 724 B 724 B Shape (181,) (181,) Count 2 Graph Layers 1 Chunks Type float32 numpy.ndarray - cosine_correction_we_filter5(bench_angle)float32dask.array<chunksize=(181,), meta=np.ndarray>
- long_name :
- Cosine correction, west to east, filter5
- units :
- 1
- comment :
- Read from cosine_correction_source
- centroid_wavelength :
- 869.3 nm
- FWHM :
- 10.0 nm
Array Chunk Bytes 724 B 724 B Shape (181,) (181,) Count 2 Graph Layers 1 Chunks Type float32 numpy.ndarray - cosine_correction_we_filter6(bench_angle)float32dask.array<chunksize=(181,), meta=np.ndarray>
- long_name :
- Cosine correction, west to east, filter6
- units :
- 1
- comment :
- Read from cosine_correction_source
- centroid_wavelength :
- 939.4 nm
- FWHM :
- 6.7 nm
Array Chunk Bytes 724 B 724 B Shape (181,) (181,) Count 2 Graph Layers 1 Chunks Type float32 numpy.ndarray - cosine_correction_we_filter7(bench_angle)float32dask.array<chunksize=(181,), meta=np.ndarray>
- long_name :
- Cosine correction, west to east, filter7
- units :
- 1
- comment :
- Read from cosine_correction_source
- centroid_wavelength :
- 1624.2 nm
- FWHM :
- 14.8 nm
Array Chunk Bytes 724 B 724 B Shape (181,) (181,) Count 2 Graph Layers 1 Chunks Type float32 numpy.ndarray - wavelength_filter1(wavelength)float32dask.array<chunksize=(750,), meta=np.ndarray>
- long_name :
- Normalized filter function data, filter 1, wavelength value obtained during instrument characterization
- units :
- nm
- centroid_wavelength :
- 413.3 nm
- FWHM :
- 10.9 nm
Array Chunk Bytes 2.93 kiB 2.93 kiB Shape (750,) (750,) Count 2 Graph Layers 1 Chunks Type float32 numpy.ndarray - normalized_transmittance_filter1(wavelength)float32dask.array<chunksize=(750,), meta=np.ndarray>
- long_name :
- Normalized filter function data, filter 1. Measured transmittance value normalized to area under curve.
- units :
- 1
- centroid_wavelength :
- 413.3 nm
- FWHM :
- 10.9 nm
Array Chunk Bytes 2.93 kiB 2.93 kiB Shape (750,) (750,) Count 2 Graph Layers 1 Chunks Type float32 numpy.ndarray - wavelength_filter2(wavelength)float32dask.array<chunksize=(750,), meta=np.ndarray>
- long_name :
- Normalized filter function data, filter 2, wavelength value obtained during instrument characterization
- units :
- nm
- centroid_wavelength :
- 501.0 nm
- FWHM :
- 10.8 nm
Array Chunk Bytes 2.93 kiB 2.93 kiB Shape (750,) (750,) Count 2 Graph Layers 1 Chunks Type float32 numpy.ndarray - normalized_transmittance_filter2(wavelength)float32dask.array<chunksize=(750,), meta=np.ndarray>
- long_name :
- Normalized filter function data, filter 2. Measured transmittance value normalized to area under curve.
- units :
- 1
- centroid_wavelength :
- 501.0 nm
- FWHM :
- 10.8 nm
Array Chunk Bytes 2.93 kiB 2.93 kiB Shape (750,) (750,) Count 2 Graph Layers 1 Chunks Type float32 numpy.ndarray - wavelength_filter3(wavelength)float32dask.array<chunksize=(750,), meta=np.ndarray>
- long_name :
- Normalized filter function data, filter 3, wavelength value obtained during instrument characterization
- units :
- nm
- centroid_wavelength :
- 613.5 nm
- FWHM :
- 10.8 nm
Array Chunk Bytes 2.93 kiB 2.93 kiB Shape (750,) (750,) Count 2 Graph Layers 1 Chunks Type float32 numpy.ndarray - normalized_transmittance_filter3(wavelength)float32dask.array<chunksize=(750,), meta=np.ndarray>
- long_name :
- Normalized filter function data, filter 3. Measured transmittance value normalized to area under curve.
- units :
- 1
- centroid_wavelength :
- 613.5 nm
- FWHM :
- 10.8 nm
Array Chunk Bytes 2.93 kiB 2.93 kiB Shape (750,) (750,) Count 2 Graph Layers 1 Chunks Type float32 numpy.ndarray - wavelength_filter4(wavelength)float32dask.array<chunksize=(750,), meta=np.ndarray>
- long_name :
- Normalized filter function data, filter 4, wavelength value obtained during instrument characterization
- units :
- nm
- centroid_wavelength :
- 671.4 nm
- FWHM :
- 10.5 nm
Array Chunk Bytes 2.93 kiB 2.93 kiB Shape (750,) (750,) Count 2 Graph Layers 1 Chunks Type float32 numpy.ndarray - normalized_transmittance_filter4(wavelength)float32dask.array<chunksize=(750,), meta=np.ndarray>
- long_name :
- Normalized filter function data, filter 4. Measured transmittance value normalized to area under curve.
- units :
- 1
- centroid_wavelength :
- 671.4 nm
- FWHM :
- 10.5 nm
Array Chunk Bytes 2.93 kiB 2.93 kiB Shape (750,) (750,) Count 2 Graph Layers 1 Chunks Type float32 numpy.ndarray - wavelength_filter5(wavelength)float32dask.array<chunksize=(750,), meta=np.ndarray>
- long_name :
- Normalized filter function data, filter 5, wavelength value obtained during instrument characterization
- units :
- nm
- centroid_wavelength :
- 869.3 nm
- FWHM :
- 10.0 nm
Array Chunk Bytes 2.93 kiB 2.93 kiB Shape (750,) (750,) Count 2 Graph Layers 1 Chunks Type float32 numpy.ndarray - normalized_transmittance_filter5(wavelength)float32dask.array<chunksize=(750,), meta=np.ndarray>
- long_name :
- Normalized filter function data, filter 5. Measured transmittance value normalized to area under curve.
- units :
- 1
- centroid_wavelength :
- 869.3 nm
- FWHM :
- 10.0 nm
Array Chunk Bytes 2.93 kiB 2.93 kiB Shape (750,) (750,) Count 2 Graph Layers 1 Chunks Type float32 numpy.ndarray - wavelength_filter6(wavelength)float32dask.array<chunksize=(750,), meta=np.ndarray>
- long_name :
- Normalized filter function data, filter 6, wavelength value obtained during instrument characterization
- units :
- nm
- centroid_wavelength :
- 939.4 nm
- FWHM :
- 6.7 nm
Array Chunk Bytes 2.93 kiB 2.93 kiB Shape (750,) (750,) Count 2 Graph Layers 1 Chunks Type float32 numpy.ndarray - normalized_transmittance_filter6(wavelength)float32dask.array<chunksize=(750,), meta=np.ndarray>
- long_name :
- Normalized filter function data, filter 6. Measured transmittance value normalized to area under curve.
- units :
- 1
- centroid_wavelength :
- 939.4 nm
- FWHM :
- 6.7 nm
Array Chunk Bytes 2.93 kiB 2.93 kiB Shape (750,) (750,) Count 2 Graph Layers 1 Chunks Type float32 numpy.ndarray - wavelength_filter7(wavelength)float32dask.array<chunksize=(750,), meta=np.ndarray>
- long_name :
- Normalized filter function data, filter 7, wavelength value obtained during instrument characterization
- units :
- nm
- centroid_wavelength :
- 1624.2 nm
- FWHM :
- 14.8 nm
Array Chunk Bytes 2.93 kiB 2.93 kiB Shape (750,) (750,) Count 2 Graph Layers 1 Chunks Type float32 numpy.ndarray - normalized_transmittance_filter7(wavelength)float32dask.array<chunksize=(750,), meta=np.ndarray>
- long_name :
- Normalized filter function data, filter 7. Measured transmittance value normalized to area under curve.
- units :
- 1
- centroid_wavelength :
- 1624.2 nm
- FWHM :
- 14.8 nm
Array Chunk Bytes 2.93 kiB 2.93 kiB Shape (750,) (750,) Count 2 Graph Layers 1 Chunks Type float32 numpy.ndarray - offset_filter1(time)float32dask.array<chunksize=(4320,), meta=np.ndarray>
- long_name :
- Offset value subtracted from hemispheric and diffuse filter1 data
- units :
- mV
Array Chunk Bytes 16.88 kiB 16.88 kiB Shape (4320,) (4320,) Count 2 Graph Layers 1 Chunks Type float32 numpy.ndarray - offset_filter2(time)float32dask.array<chunksize=(4320,), meta=np.ndarray>
- long_name :
- Offset value subtracted from hemispheric and diffuse filter2 data
- units :
- mV
Array Chunk Bytes 16.88 kiB 16.88 kiB Shape (4320,) (4320,) Count 2 Graph Layers 1 Chunks Type float32 numpy.ndarray - offset_filter3(time)float32dask.array<chunksize=(4320,), meta=np.ndarray>
- long_name :
- Offset value subtracted from hemispheric and diffuse filter3 data
- units :
- mV
Array Chunk Bytes 16.88 kiB 16.88 kiB Shape (4320,) (4320,) Count 2 Graph Layers 1 Chunks Type float32 numpy.ndarray - offset_filter4(time)float32dask.array<chunksize=(4320,), meta=np.ndarray>
- long_name :
- Offset value subtracted from hemispheric and diffuse filter4 data
- units :
- mV
Array Chunk Bytes 16.88 kiB 16.88 kiB Shape (4320,) (4320,) Count 2 Graph Layers 1 Chunks Type float32 numpy.ndarray - offset_filter5(time)float32dask.array<chunksize=(4320,), meta=np.ndarray>
- long_name :
- Offset value subtracted from hemispheric and diffuse filter5 data
- units :
- mV
Array Chunk Bytes 16.88 kiB 16.88 kiB Shape (4320,) (4320,) Count 2 Graph Layers 1 Chunks Type float32 numpy.ndarray - offset_filter6(time)float32dask.array<chunksize=(4320,), meta=np.ndarray>
- long_name :
- Offset value subtracted from hemispheric and diffuse filter6 data
- units :
- mV
Array Chunk Bytes 16.88 kiB 16.88 kiB Shape (4320,) (4320,) Count 2 Graph Layers 1 Chunks Type float32 numpy.ndarray - offset_filter7(time)float32dask.array<chunksize=(4320,), meta=np.ndarray>
- long_name :
- Offset value subtracted from hemispheric and diffuse filter7 data
- units :
- mV
Array Chunk Bytes 16.88 kiB 16.88 kiB Shape (4320,) (4320,) Count 2 Graph Layers 1 Chunks Type float32 numpy.ndarray - diffuse_correction_filter1()float32...
- long_name :
- Cosine correction of filter1 diffuse, assuming Rayleigh sky at 45 deg zenith angle
- units :
- 1
array(0.993)
- diffuse_correction_filter2()float32...
- long_name :
- Cosine correction of filter2 diffuse, assuming Rayleigh sky at 45 deg zenith angle
- units :
- 1
array(0.999)
- diffuse_correction_filter3()float32...
- long_name :
- Cosine correction of filter3 diffuse, assuming Rayleigh sky at 45 deg zenith angle
- units :
- 1
array(1.)
- diffuse_correction_filter4()float32...
- long_name :
- Cosine correction of filter4 diffuse, assuming Rayleigh sky at 45 deg zenith angle
- units :
- 1
array(1.004)
- diffuse_correction_filter5()float32...
- long_name :
- Cosine correction of filter5 diffuse, assuming Rayleigh sky at 45 deg zenith angle
- units :
- 1
array(1.007)
- diffuse_correction_filter6()float32...
- long_name :
- Cosine correction of filter6 diffuse, assuming Rayleigh sky at 45 deg zenith angle
- units :
- 1
array(1.007)
- diffuse_correction_filter7()float32...
- long_name :
- Cosine correction of filter7 diffuse, assuming Rayleigh sky at 45 deg zenith angle
- units :
- 1
array(0.996)
- nominal_calibration_factor_filter1()float32...
- long_name :
- Nominal calibration factor using standard lamp, applied to filter1 data
- units :
- mV/(W/(m^2 nm))
array(91.797997)
- nominal_calibration_factor_filter2()float32...
- long_name :
- Nominal calibration factor using standard lamp, applied to filter2 data
- units :
- mV/(W/(m^2 nm))
array(85.822197)
- nominal_calibration_factor_filter3()float32...
- long_name :
- Nominal calibration factor using standard lamp, applied to filter3 data
- units :
- mV/(W/(m^2 nm))
array(98.297401)
- nominal_calibration_factor_filter4()float32...
- long_name :
- Nominal calibration factor using standard lamp, applied to filter4 data
- units :
- mV/(W/(m^2 nm))
array(86.158302)
- nominal_calibration_factor_filter5()float32...
- long_name :
- Nominal calibration factor using standard lamp, applied to filter5 data
- units :
- mV/(W/(m^2 nm))
array(164.6082)
- nominal_calibration_factor_filter6()float32...
- long_name :
- Nominal calibration factor using standard lamp, applied to filter6 data
- units :
- mV/(W/(m^2 nm))
array(340.009796)
- nominal_calibration_factor_filter7()float32...
- long_name :
- Nominal calibration factor using standard lamp, applied to filter7 data
- units :
- mV/(W/(m^2 nm))
array(30.)
- lat()float32...
- long_name :
- North latitude
- units :
- degree_N
- valid_min :
- -90.0
- valid_max :
- 90.0
- standard_name :
- latitude
array(36.881, dtype=float32)
- lon()float32...
- long_name :
- East longitude
- units :
- degree_E
- valid_min :
- -180.0
- valid_max :
- 180.0
- standard_name :
- longitude
array(-98.285, dtype=float32)
- alt()float32...
- long_name :
- Altitude above mean sea level
- units :
- m
- standard_name :
- altitude
array(360., dtype=float32)
- command_line :
- mfrsr7nch_ingest -s sgp -f E11
- Conventions :
- ARM-1.2
- process_version :
- ingest-mfrsr7nch-1.3-0.el7
- dod_version :
- mfrsr7nch-b1-1.1
- input_source :
- /data/collection/sgp/sgpmfrsr7nchE11.00/MFRSR_Table155.20210329070000.dat
- site_id :
- sgp
- platform_id :
- mfrsr7nch
- facility_id :
- E11
- data_level :
- b1
- location_description :
- Southern Great Plains (SGP), Byron, Oklahoma
- datastream :
- sgpmfrsr7nchE11.b1
- serial_number :
- Refer to logger_id and head_id
- sampling_interval :
- 20 seconds
- logger_software_version :
- 1.2
- logger_id :
- 1760
- head_id :
- 180056578
- mfr_internal_latitude :
- 36.881000
- mfr_internal_longitude :
- -98.285000
- filter_information :
- Filters 1-5 and 7 for aerosol, and filter 6 for water vapor.
- shadowband_timing :
- Due to shadowband motion, there is an inherent lag between the time stamp in the file and the time corresponding to the direct beam measurement which varies throughout the day. On average this lag is about five seconds, therefore five seconds are added to the timestamp when calculating solar position.
- cosine_correction_source :
- CosineCorr.sgpmfrsr7nchE11.20201209.dat
- diffuse_correction_source :
- DiffuseCorr.sgpmfrsr7nchE11.20201209.dat
- filter_trace_source :
- FilterInfo.sgpmfrsr7nchE11.20201209.dat
- nominal_calibration_source :
- NominalCal.sgpmfrsr7nchE11.20201209.dat
- offset_correction_source :
- Offsets are computed using a two hour average of the alltime_hemisp_narrowband data centered around the time of the maximum solar zenith angle. For higher and lower latitudes the averaging window can shrink to only include values where the solar zenith angle is >= 97 degrees. If less than 30 minutes of nighttime data is available the previously computed values will be used.
- doi :
- 10.5439/1429369
- history :
- created by user dsmgr on machine flint at 2021-03-29 09:43:00, using ingest-mfrsr7nch-1.3-0.el7
- _file_dates :
- ['20210329']
- _file_times :
- ['070000']
- _datastream :
- sgpmfrsr7nchE11.b1
- _arm_standards_flag :
- 1
First Visualization
Let’s plot up some data to see what we’re working with. For this example, we’ll use diffuse_hemisp_narrowband_filter4
variable = 'diffuse_hemisp_narrowband_filter4'
# Create a plotting display object with 2 plots
display = act.plotting.TimeSeriesDisplay(ds, figsize=(15, 10), subplot_shape=(2,))
# Plot up the diffuse variable in the first plot
display.plot(variable, subplot_index=(0,))
# Plot up a day/night background
display.day_night_background(subplot_index=(0,))
# Plot up the QC variable in the second plot
display.qc_flag_block_plot(variable, subplot_index=(1,))
plt.show()
/Users/mgrover/miniforge3/envs/pyart-docs/lib/python3.10/site-packages/act/utils/datetime_utils.py:132: FutureWarning: Unlike other reduction functions (e.g. `skew`, `kurtosis`), the default behavior of `mode` typically preserves the axis it acts along. In SciPy 1.11.0, this behavior will change: the default value of `keepdims` will become False, the `axis` over which the statistic is taken will be eliminated, and the value None will no longer be accepted. Set `keepdims` to True or False to avoid this warning.
mode = stats.mode(np.diff(time))
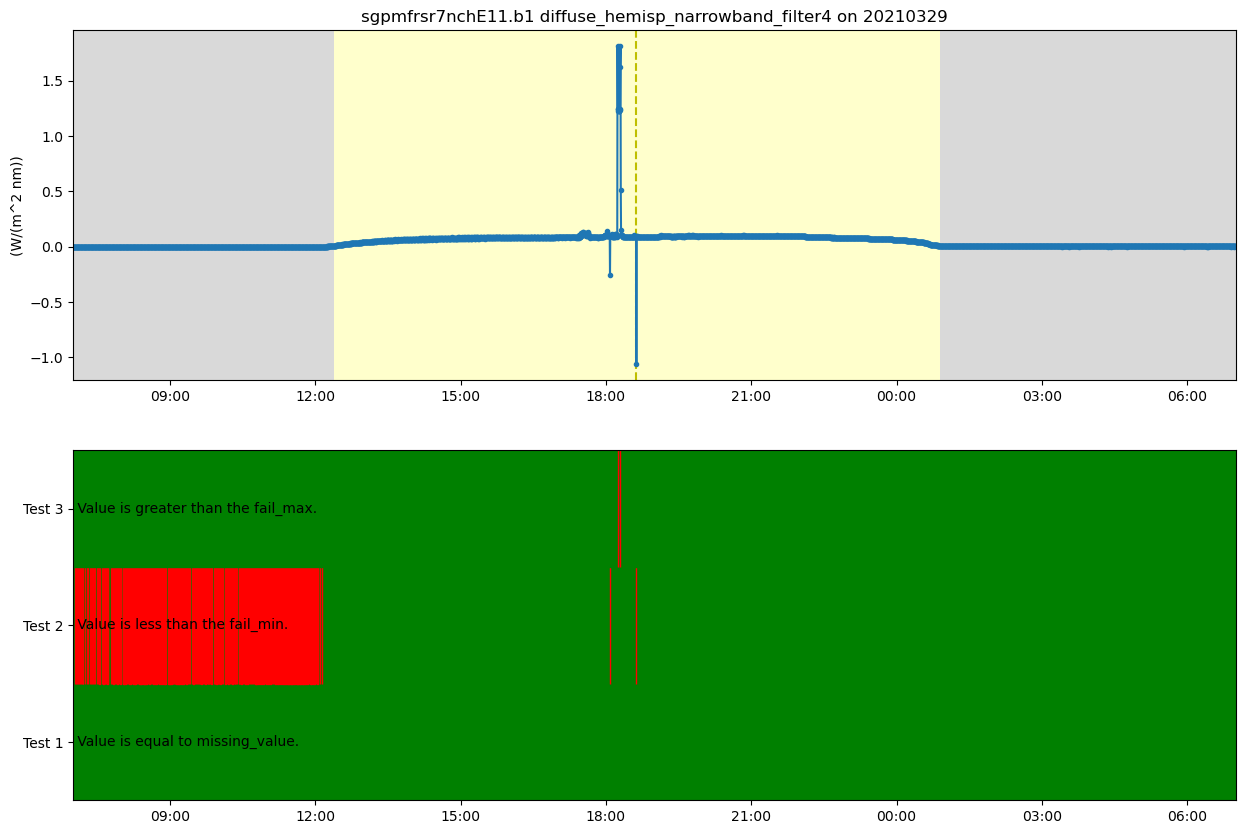
Filter Data
Let’s try and filter some of those outliers out based on the embedded QC in the files.
# Now lets remove some of these outliers
ds.qcfilter.datafilter(variable, rm_tests=[2, 3], del_qc_var=False)
# And plot the data again
# Create a plotting display object with 2 plots
display = act.plotting.TimeSeriesDisplay(ds, figsize=(15, 10), subplot_shape=(2,))
# Plot up the diffuse variable in the first plot
display.plot(variable, subplot_index=(0,))
# Plot up a day/night background
display.day_night_background(subplot_index=(0,))
# Plot up the QC variable in the second plot
display.qc_flag_block_plot(variable, subplot_index=(1,))
plt.show()
/Users/mgrover/miniforge3/envs/pyart-docs/lib/python3.10/site-packages/act/utils/datetime_utils.py:132: FutureWarning: Unlike other reduction functions (e.g. `skew`, `kurtosis`), the default behavior of `mode` typically preserves the axis it acts along. In SciPy 1.11.0, this behavior will change: the default value of `keepdims` will become False, the `axis` over which the statistic is taken will be eliminated, and the value None will no longer be accepted. Set `keepdims` to True or False to avoid this warning.
mode = stats.mode(np.diff(time))
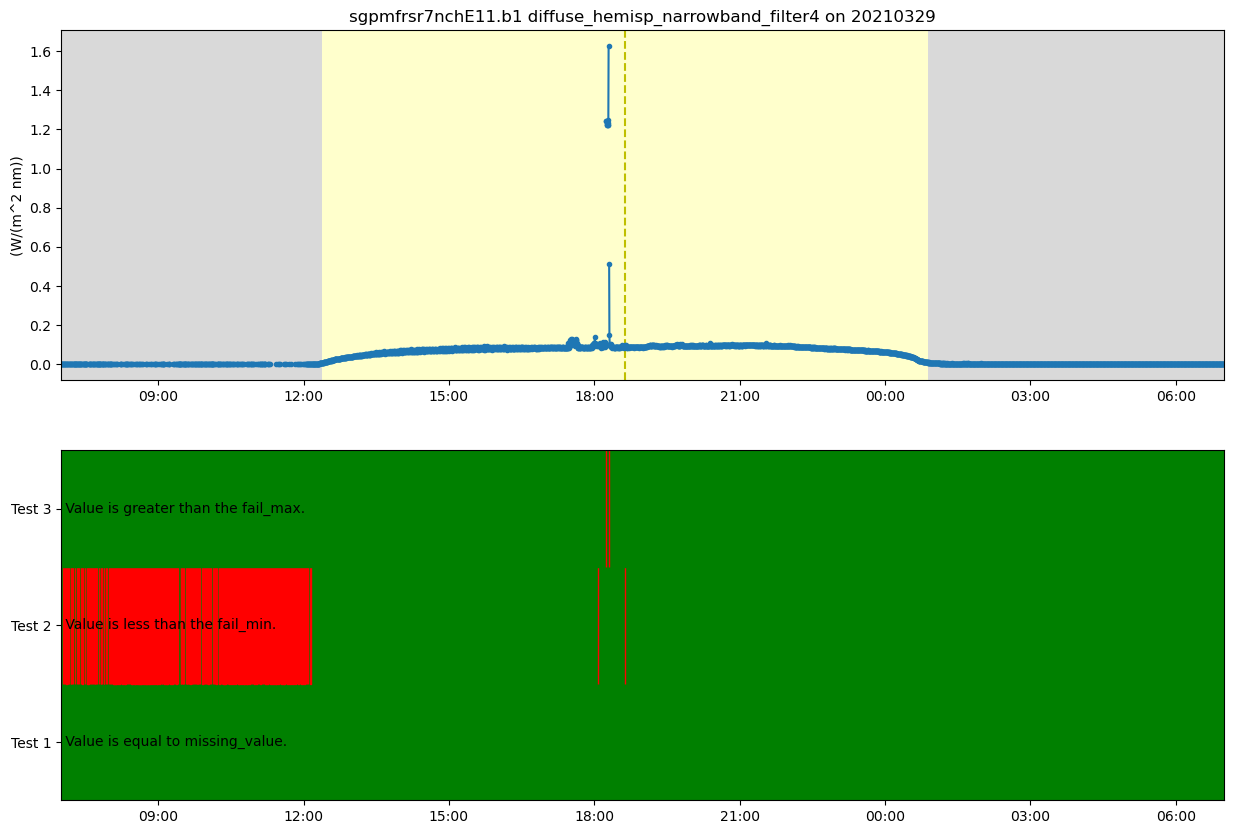
Instrument Specific QC Tests
ACT has a growing library of instrument specific tests such as the fast-fourier transform test to detect shading which was adapted from Alexandrov et al 2007. The adaption is that it is applied in a moving window style approach. Note - Check out the webpage as an example of how we are including references to papers behind the codes
Let’s apply it and see how it compares with the DQR!
# Apply test
ds = act.qc.fft_shading_test(ds, variable=variable)
# Create a plotting display object with 2 plots
display = act.plotting.TimeSeriesDisplay(ds, figsize=(15, 10), subplot_shape=(2,))
# Plot up the diffuse variable in the first plot
display.plot(variable, subplot_index=(0,))
# Plot up a day/night background
display.day_night_background(subplot_index=(0,))
# Plot up the QC variable in the second plot
display.qc_flag_block_plot(variable, subplot_index=(1,))
plt.show()
/Users/mgrover/miniforge3/envs/pyart-docs/lib/python3.10/site-packages/act/utils/datetime_utils.py:132: FutureWarning: Unlike other reduction functions (e.g. `skew`, `kurtosis`), the default behavior of `mode` typically preserves the axis it acts along. In SciPy 1.11.0, this behavior will change: the default value of `keepdims` will become False, the `axis` over which the statistic is taken will be eliminated, and the value None will no longer be accepted. Set `keepdims` to True or False to avoid this warning.
mode = stats.mode(np.diff(time))
/Users/mgrover/miniforge3/envs/pyart-docs/lib/python3.10/site-packages/act/utils/datetime_utils.py:132: FutureWarning: Unlike other reduction functions (e.g. `skew`, `kurtosis`), the default behavior of `mode` typically preserves the axis it acts along. In SciPy 1.11.0, this behavior will change: the default value of `keepdims` will become False, the `axis` over which the statistic is taken will be eliminated, and the value None will no longer be accepted. Set `keepdims` to True or False to avoid this warning.
mode = stats.mode(np.diff(time))
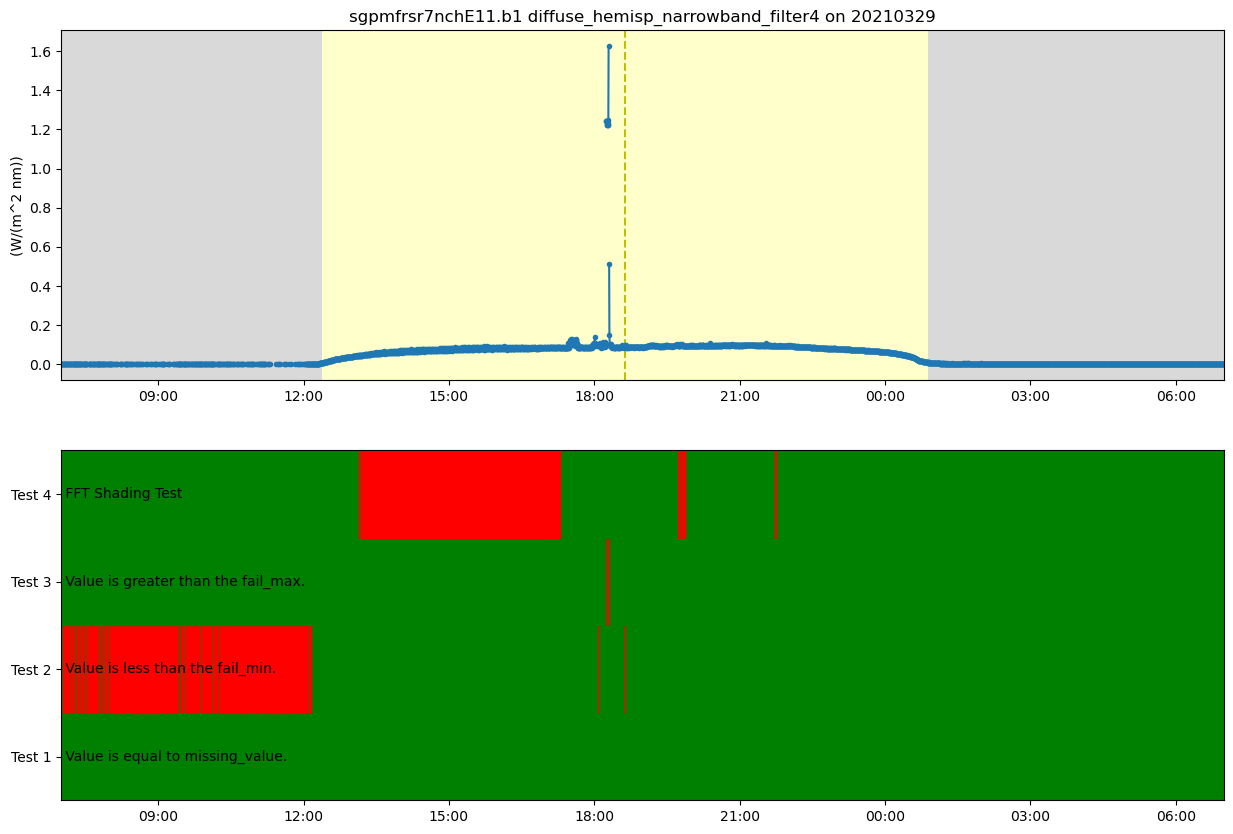
Conclusion
In this tutorial, we have shown you how to download data from ARM’s Data Live web service, visualize the data and QC information, filter data based on the QC, and add new QC tests to the dataset. After all this work, you can easily save the xarray object to a NetCDF file using obj.to_netcdf('filename.nc')
and all that data will be saved and usable in Python and ACT.
Please checkout the ACT Github repository for the latest and greatest information, including our documentation which has examples that can be downloading in python or Jupyter Notebook formats.
Second ACT!
But wait, there’s more to ACT that we can explore together or that you can do on your own! These examples are going to be more condensed than the above but should still provide you the insight you need to run and do your own things!
We are going to need some additional libraries to help out though!
Wind Roses
# Let's download a month of surface meteorological data from the SGP central facility!
results = act.discovery.download_data(username, token, 'sgpmetE13.b1', '2021-05-01', '2021-05-31')
# Read that data into an object (this will concatenate it all for you)
ds = act.io.armfiles.read_netcdf(results)
# Now we can plot up a wind rose of that entire month's worth of data
windrose = act.plotting.WindRoseDisplay(ds, figsize=(10,8))
windrose.plot('wdir_vec_mean', 'wspd_vec_mean', spd_bins=np.linspace(0, 10, 5))
windrose.axes[0].legend()
plt.show()
[DOWNLOADING] sgpmetE13.b1.20210514.000000.cdf
[DOWNLOADING] sgpmetE13.b1.20210516.000000.cdf
[DOWNLOADING] sgpmetE13.b1.20210519.000000.cdf
[DOWNLOADING] sgpmetE13.b1.20210513.000000.cdf
[DOWNLOADING] sgpmetE13.b1.20210512.000000.cdf
[DOWNLOADING] sgpmetE13.b1.20210520.000000.cdf
[DOWNLOADING] sgpmetE13.b1.20210517.000000.cdf
[DOWNLOADING] sgpmetE13.b1.20210518.000000.cdf
[DOWNLOADING] sgpmetE13.b1.20210522.000000.cdf
[DOWNLOADING] sgpmetE13.b1.20210521.000000.cdf
[DOWNLOADING] sgpmetE13.b1.20210523.000000.cdf
[DOWNLOADING] sgpmetE13.b1.20210515.000000.cdf
[DOWNLOADING] sgpmetE13.b1.20210511.000000.cdf
[DOWNLOADING] sgpmetE13.b1.20210502.000000.cdf
[DOWNLOADING] sgpmetE13.b1.20210501.000000.cdf
[DOWNLOADING] sgpmetE13.b1.20210509.000000.cdf
[DOWNLOADING] sgpmetE13.b1.20210505.000000.cdf
[DOWNLOADING] sgpmetE13.b1.20210508.000000.cdf
[DOWNLOADING] sgpmetE13.b1.20210510.000000.cdf
[DOWNLOADING] sgpmetE13.b1.20210503.000000.cdf
[DOWNLOADING] sgpmetE13.b1.20210504.000000.cdf
[DOWNLOADING] sgpmetE13.b1.20210506.000000.cdf
[DOWNLOADING] sgpmetE13.b1.20210507.000000.cdf
[DOWNLOADING] sgpmetE13.b1.20210528.000000.cdf
[DOWNLOADING] sgpmetE13.b1.20210529.000000.cdf
[DOWNLOADING] sgpmetE13.b1.20210530.000000.cdf
[DOWNLOADING] sgpmetE13.b1.20210531.000000.cdf
[DOWNLOADING] sgpmetE13.b1.20210525.000000.cdf
[DOWNLOADING] sgpmetE13.b1.20210524.000000.cdf
[DOWNLOADING] sgpmetE13.b1.20210526.000000.cdf
[DOWNLOADING] sgpmetE13.b1.20210527.000000.cdf
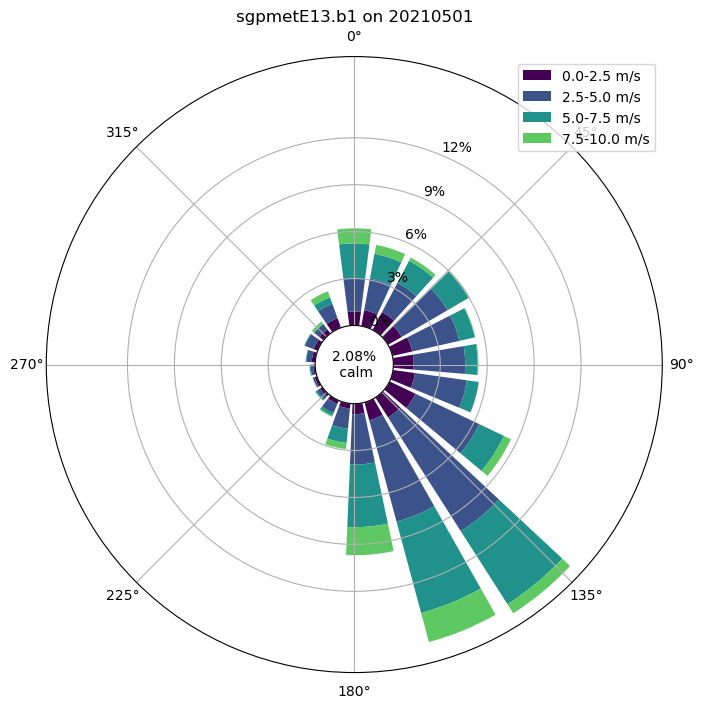
Present Weather Detector Codes
With the MET system at the main site, there’s also a present weather detector (PWD) deployed. This PWD reports the present weather in WMO codes but can be easily decoded using a utility in ACT. With this information, you can make fancy plots like the DQ Office plots for the PWD.
# Let's just use one of the files from the previous example
ds = act.io.armfiles.read_netcdf(results[21])
# Pass it to the function to decode it along with the variable name
ds = act.utils.inst_utils.decode_present_weather(ds, variable='pwd_pw_code_inst')
# We're going to print out the first 10 decoded values that weren't 0
# This shows the utility of also being able to use the built-in xarray
# features like where!
print(list(ds['pwd_pw_code_inst_decoded'].where(ds.pwd_pw_code_inst > 0, drop=True).values[0:10]))
['Rain, not freezing, slight', 'Rain, not freezing, slight', 'Drizzle, not freezing, slight', 'Drizzle, not freezing, slight', 'Drizzle, not freezing, slight', 'Drizzle, not freezing, slight', 'Drizzle, not freezing, slight', 'Drizzle, not freezing, slight', 'Drizzle, not freezing, slight', 'Drizzle, not freezing, slight']
Doppler Lidar Wind Retrievals
This will show you how you can process the doppler lidar PPI scans to produce wind profiles based on Newsom et al 2016.
# We're going to use some test data that already exists within ACT
ds = act.io.armfiles.read_netcdf(act.tests.sample_files.EXAMPLE_DLPPI_MULTI)
# Returns the wind retrieval information in a new object by default
# Note that the default snr_threshold of 0.008 was too high for the first profile
# Reducing it to 0.002 makes it show up but the quality of the data is likely suspect.
wind_ds = act.retrievals.compute_winds_from_ppi(ds, snr_threshold=0.002)
# Plot it up
display = act.plotting.TimeSeriesDisplay(wind_ds)
display.plot_barbs_from_spd_dir('wind_direction', 'wind_speed', invert_y_axis=False)
#Update the x-limits to make sure both wind profiles are shown
display.axes[0].set_xlim([np.datetime64('2019-10-15T11:45'), np.datetime64('2019-10-15T12:30')])
plt.show()
/Users/mgrover/miniforge3/envs/pyart-docs/lib/python3.10/site-packages/act/plotting/plot.py:80: UserWarning: Could not discern datastreamname and dict or tuple were not provided. Using defaultname of act_datastream!
warnings.warn(
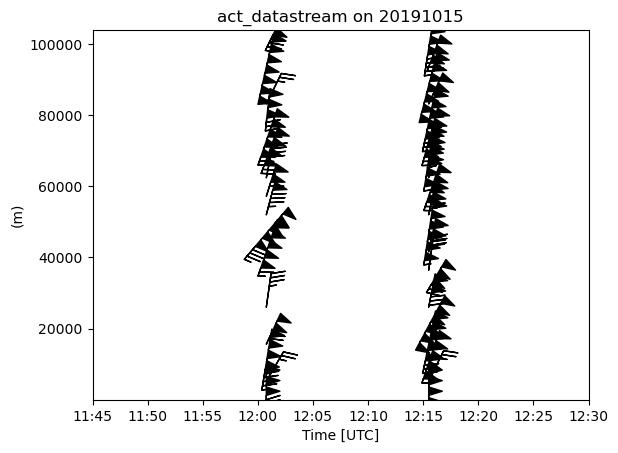
Radiosonde Plotting and More!
This will take you through how to plot up a Skew-T plot along with a geographic plot of the radiosonde track on a map. Additionally, will run this through a retrieval to calculate the PBL height using the Liu Liang method.
# Import MetPy if possible
import metpy
# Read in sample radiosonde data and plot up a Skew-T
ds = act.io.armfiles.read_netcdf(act.tests.EXAMPLE_SONDE1)
skewt = act.plotting.SkewTDisplay(ds, figsize=(10, 8))
skewt.plot_from_u_and_v('u_wind', 'v_wind', 'pres', 'tdry', 'dp')
plt.show()
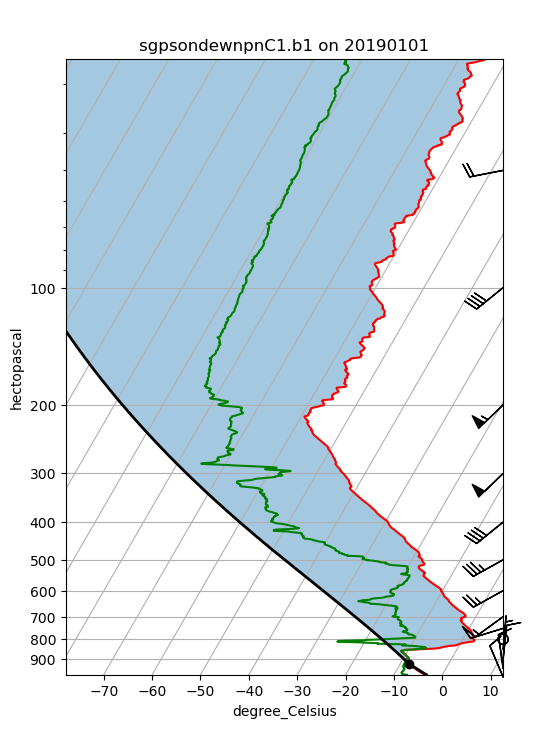
# Now let's plot up the radiosonde path on a map!
display = act.plotting.GeographicPlotDisplay(ds)
display.geoplot(data_field='pres', title='Radiosonde Path')
plt.show()
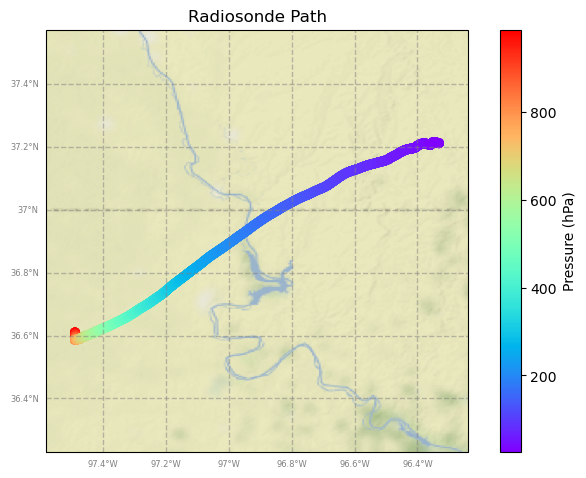
# We need to update the units on temperature before running the retrieval
ds.utils.change_units(variables='tdry', desired_unit='degree_Celsius')
obj = act.retrievals.calculate_pbl_liu_liang(ds)
print('Regime = ', obj['pblht_regime_liu_liang'].values, '\nPBL Height = ', int(obj['pblht_liu_liang'].values))
Regime = NRL
PBL Height = 950
Look at Data from SAIL
ACT is not just limited in ARM data… you can also work with data from other organizations, such as NOAA!
During the SAIL and SPLASH field campaigns in Colorado, they deployed a NOAA Snow Level Radar, “FMCW”, around the domain. Let’s download and plot some data! In addition to this radar data, we can also read in some Parsivel data, which is a Laser Disdrometer!
Load in the Data
We use the act.discovery
module to load in data for the PSL, and remotely read in the data for the Parsivel data.
# Use the ACT downloader to download a file from the
# Kettle Ponds site on 8/01/2022 between 2200 and 2300 UTC.
data_22_utc = act.discovery.download_noaa_psl_data(
site='kps', instrument='Radar FMCW Moment', startdate='20220801', hour='22')
data_23_utc = act.discovery.download_noaa_psl_data(
site='kps', instrument='Radar FMCW Moment', startdate='20220801', hour='23')
# Read in the .raw files from both hours. Spectra data are also downloaded.
radar_ds = act.io.noaapsl.read_psl_radar_fmcw_moment([result_22[-1], result_23[-1]])
# Read in the parsivel text files.
url = ['https://downloads.psl.noaa.gov/psd2/data/realtime/DisdrometerParsivel/Stats/kps/2022/213/kps2221322_stats.txt',
'https://downloads.psl.noaa.gov/psd2/data/realtime/DisdrometerParsivel/Stats/kps/2022/213/kps2221323_stats.txt']
parsivel_ds = act.io.noaapsl.read_psl_parsivel(url)
Downloading dkps2221322a.mom
Downloading hkps2221322a.mom
Downloading kps2221322.raw
Downloading dkps2221323a.mom
Downloading hkps2221323a.mom
Downloading kps2221323.raw
---------------------------------------------------------------------------
NameError Traceback (most recent call last)
Input In [15], in <cell line: 9>()
5 data_23_utc = act.discovery.download_noaa_psl_data(
6 site='kps', instrument='Radar FMCW Moment', startdate='20220801', hour='23')
8 # Read in the .raw files from both hours. Spectra data are also downloaded.
----> 9 radar_ds = act.io.noaapsl.read_psl_radar_fmcw_moment([result_22[-1], result_23[-1]])
11 # Read in the parsivel text files.
12 url = ['https://downloads.psl.noaa.gov/psd2/data/realtime/DisdrometerParsivel/Stats/kps/2022/213/kps2221322_stats.txt',
13 'https://downloads.psl.noaa.gov/psd2/data/realtime/DisdrometerParsivel/Stats/kps/2022/213/kps2221323_stats.txt']
NameError: name 'result_22' is not defined
Visualize using the TimeSeriesDisplay
Now that we have our data, we can visualize it using a TimeSeriesDisplay
!
# Create a TimeSeriesDisplay object using both datasets.
display = act.plotting.TimeSeriesDisplay(
{"NOAA Site KPS PSL Radar FMCW": radar_ds, "NOAA Site KPS Parsivel": parsivel_ds},
subplot_shape=(2,), figsize=(10, 10))
# Plot PSL Radar followed by the parsivel data.
display.plot('reflectivity_uncalibrated', dsname='NOAA Site KPS PSL Radar FMCW',
cmap='act_HomeyerRainbow', subplot_index=(0,))
display.plot('number_density_drops', dsname='NOAA Site KPS Parsivel',
cmap='act_HomeyerRainbow', subplot_index=(1,))
# Adjust ylims of parsivel plot.
display.axes[1].set_ylim([0, 10])
plt.show()
Conclusions and Additional Resources
Within this notebook, we detailed the key functionality of the Atmospheric data Community Toolkit (ACT), and how to go about analyzing different atmospheric and climate datasets using this package.
If you are interested in additional examples, be sure to check out: